Temporal shuffling for defending deep action recognition models against adversarial attacks
NEURAL NETWORKS(2024)
摘要
Recently, video-based action recognition methods using convolutional neural networks (CNNs) achieve remarkable recognition performance. However, there is still lack of understanding about the generalization mechanism of action recognition models. In this paper, we suggest that action recognition models rely on the motion information less than expected, and thus they are robust to randomization of frame orders. Furthermore, we find that motion monotonicity remaining after randomization also contributes to such robustness. Based on this observation, we develop a novel defense method using temporal shuffling of input videos against adversarial attacks for action recognition models. Another observation enabling our defense method is that adversarial perturbations on videos are sensitive to temporal destruction. To the best of our knowledge, this is the first attempt to design a defense method without additional training for 3D CNN-based video action recognition models.
更多查看译文
关键词
Adversarial attack/defense,Action recognition,Temporal information in action recognition
AI 理解论文
溯源树
样例
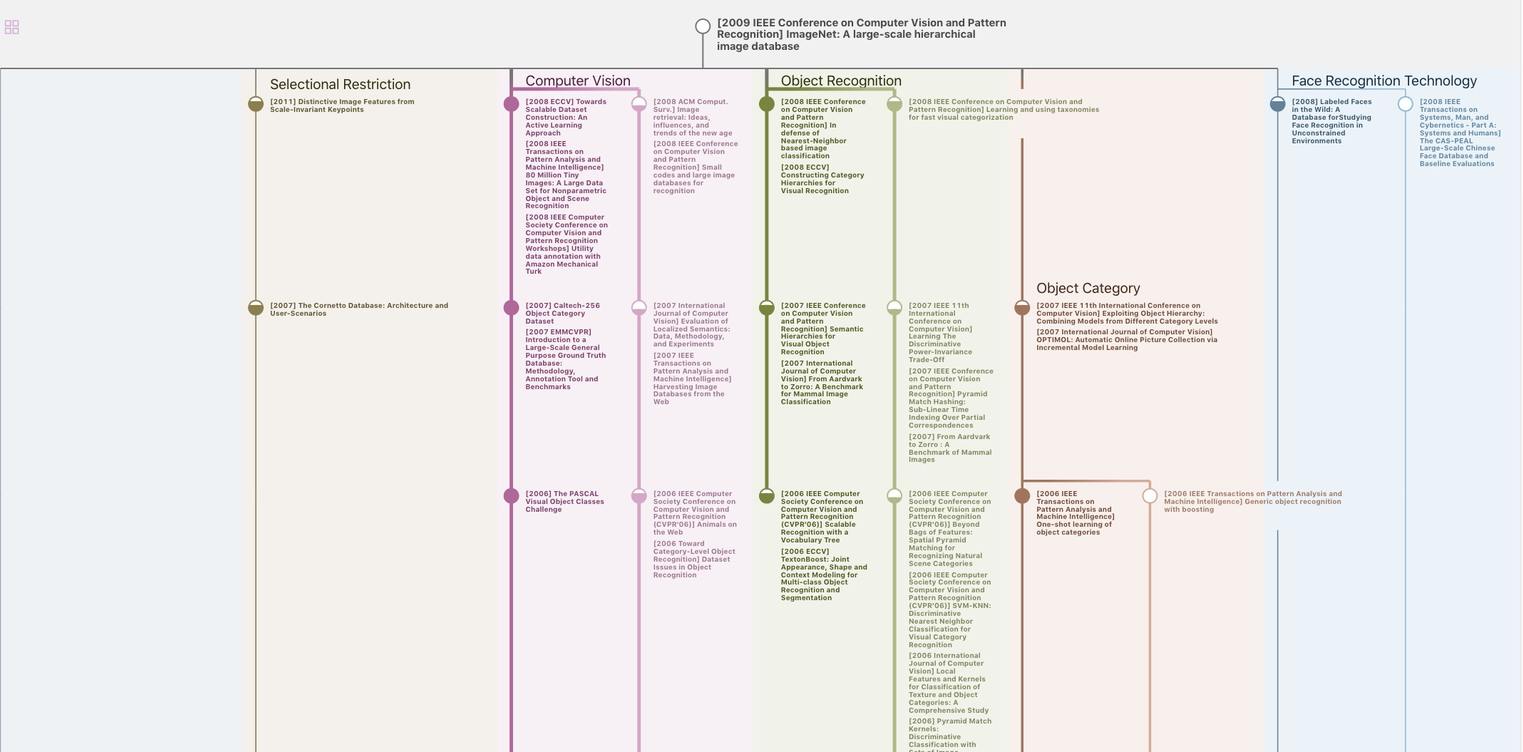
生成溯源树,研究论文发展脉络
Chat Paper
正在生成论文摘要