Virtual biopsy in prostate cancer: can machine learning distinguish low and high aggressive tumors on MRI?
2021 43RD ANNUAL INTERNATIONAL CONFERENCE OF THE IEEE ENGINEERING IN MEDICINE & BIOLOGY SOCIETY (EMBC)(2021)
摘要
In the last decades, MRI was proven a useful tool for the diagnosis and characterization of Prostate Cancer (PCa). In the literature, many studies focused on characterizing PCa aggressiveness, but a few have distinguished between low-aggressive (Gleason Grade Group (GG) <=2) and high-aggressive (GG>=3) PCas based on biparametric MRI (bpMRI). In this study, 108 PCas were collected from two different centers and were divided into training, testing, and validation set. From Apparent Diffusion Coefficient (ADC) map and T2-Weighted Image (T2WI), we extracted texture features, both 3D and 2D, and we implemented three different methods of Feature Selection (FS): Minimum Redundance Maximum Relevance (MRMR), Affinity Propagation (AP), and Genetic Algorithm (GA). From the resulting subsets of predictors, we trained Support Vector Machine (SVM), Decision Tree, and Ensemble Learning classifiers on the training set, and we evaluated their prediction ability on the testing set. Then, for each FS method, we chose the best classifier, based on both training and testing performances, and we further assessed their generalization capability on the validation set. Between the three best models, a Decision Tree was trained using only two features extracted from the ADC map and selected by MRMR, achieving, on the validation set, an Area Under the ROC (AUC) equal to 81%, with sensitivity and specificity of 77% and 93%, respectively.
更多查看译文
关键词
virtual biopsy,prostate cancer,machine learning,high aggressive tumors
AI 理解论文
溯源树
样例
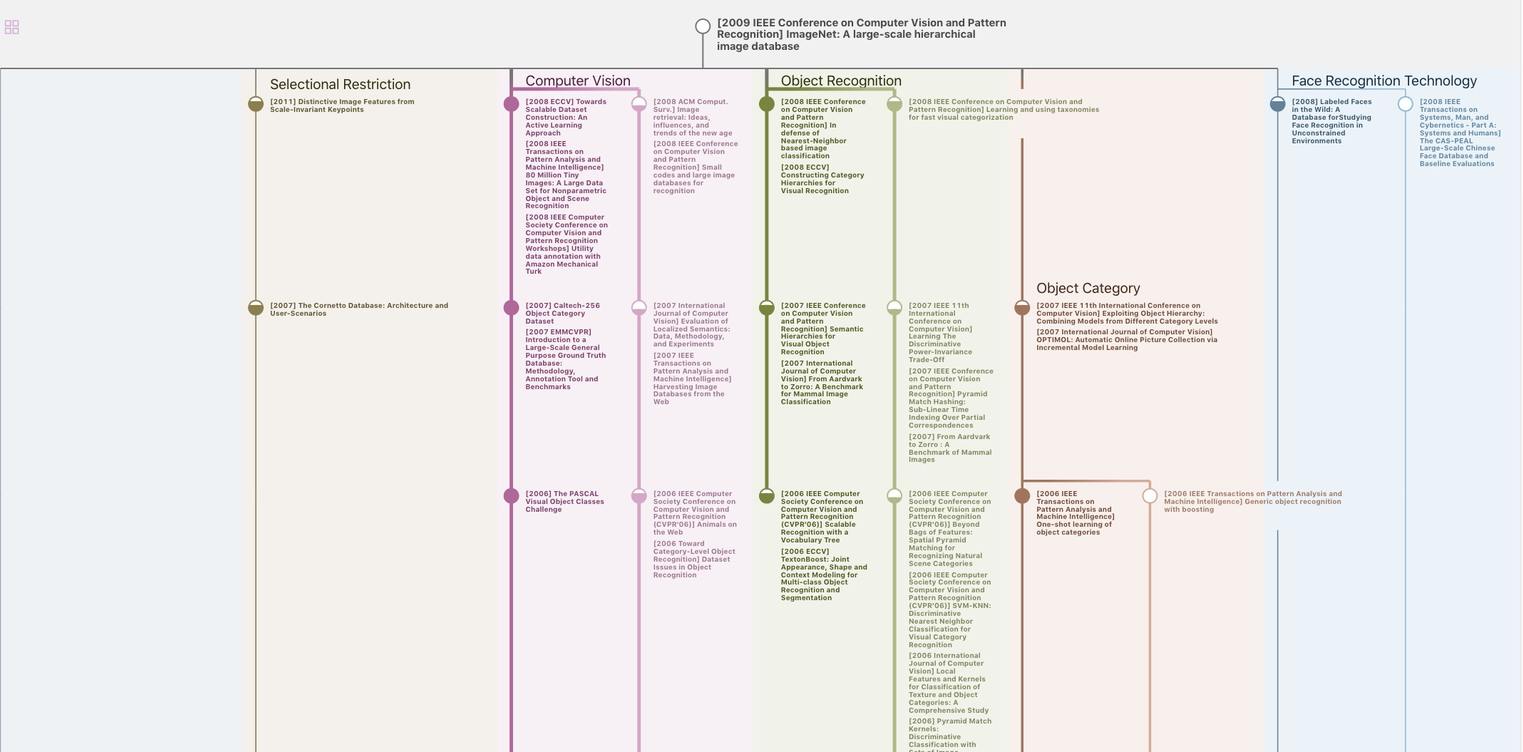
生成溯源树,研究论文发展脉络
Chat Paper
正在生成论文摘要