Trainable Preprocessing for Reduced Precision Neural Networks
29TH EUROPEAN SIGNAL PROCESSING CONFERENCE (EUSIPCO 2021)(2021)
摘要
Applications of neural networks are emerging in many fields and are frequently implemented in embedded environment, introducing power, throughput and latency constraints next to accuracy. Although practical computer vision solutions always involve some kind of preprocessing, most research focuses on the network itself. As a result, the preprocessing remains optimized for the human perception and is not tuned to neural networks. We propose the optimization of preprocesing along with the network using backpropagation and gradient descent. This open up the accuracy versus implementation cost design space towards more cost-efficient implementations by exploiting reduced precision input. In particular, we evaluate the effect of two preprocessing techniques: color conversion and dithering, using CIFAR10 and ImageNet datasets with different networks.
更多查看译文
关键词
Data Preprocessing, Quantized Neural Networks
AI 理解论文
溯源树
样例
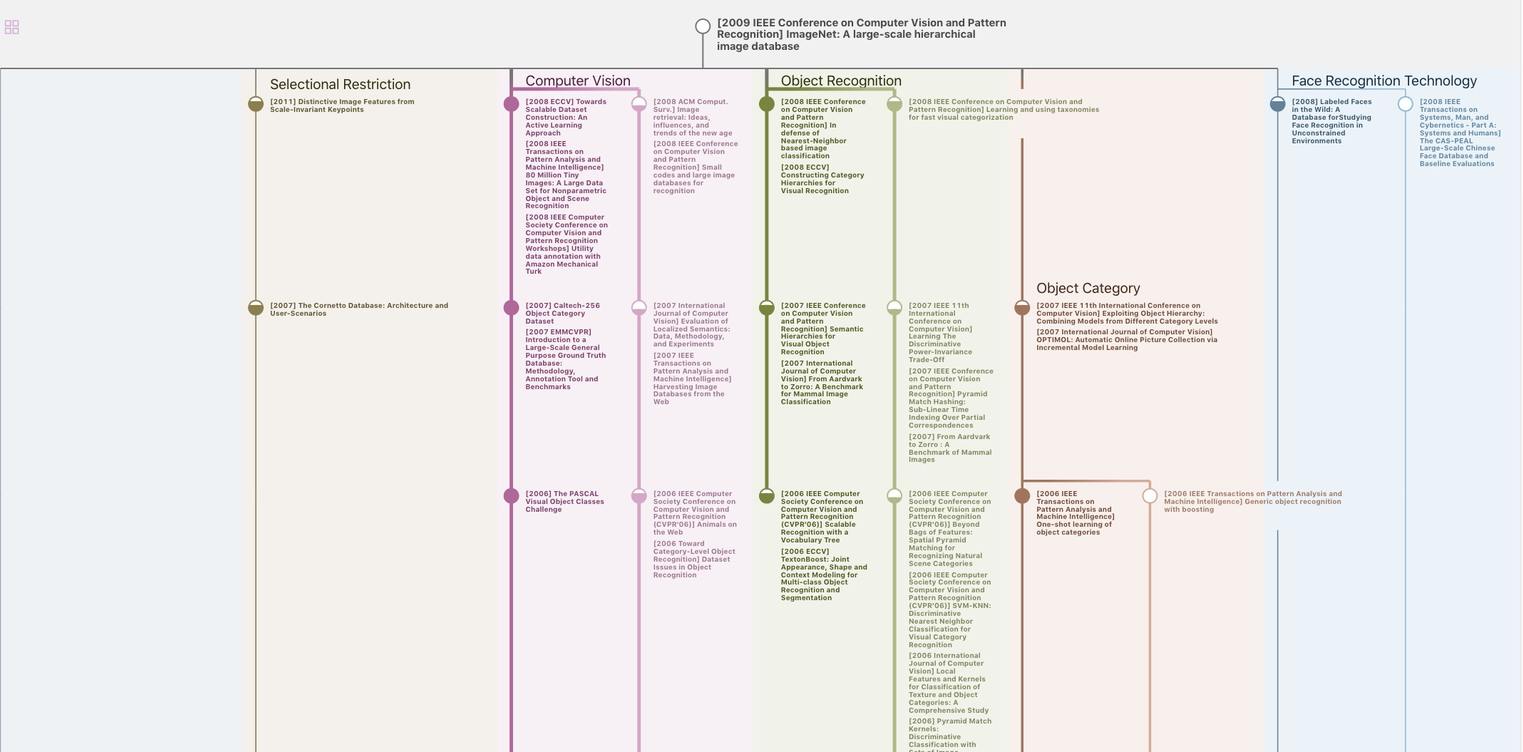
生成溯源树,研究论文发展脉络
Chat Paper
正在生成论文摘要