Matrix Factor Analysis: From Least Squares to Iterative Projection
JOURNAL OF BUSINESS & ECONOMIC STATISTICS(2024)
摘要
In this article, we study large-dimensional matrix factor models and estimate the factor loading matrices and factor score matrix by minimizing square loss function. Interestingly, the resultant estimators coincide with the Projected Estimators (PE) in Yu et al.(2022), which was proposed from the perspective of simultaneous reduction of the dimensionality and the magnitudes of the idiosyncratic error matrix. In other word, we provide a least-square interpretation of the PE for matrix factor model, which parallels to the least-square interpretation of the PCA for the vector factor model. We derive the convergence rates of the theoretical minimizers under sub-Gaussian tails. Considering the robustness to the heavy tails of the idiosyncratic errors, we extend the least squares to minimizing the Huber loss function, which leads to a weighted iterative projection approach to compute and learn the parameters. We also derive the convergence rates of the theoretical minimizers of the Huber loss function under bounded (2+e)th moment of the idiosyncratic errors. We conduct extensive numerical studies to investigate the empirical performance of the proposed Huber estimators relative to the state-of-the-art ones. The Huber estimators perform robustly and much better than existing ones when the data are heavy-tailed, and as a result can be used as a safe replacement in practice. An application to a Fama-French financial portfolio dataset demonstrates the empirical advantage of the Huber estimator.
更多查看译文
关键词
Huber loss,Least squares,Matrix factor model,Projection estimation
AI 理解论文
溯源树
样例
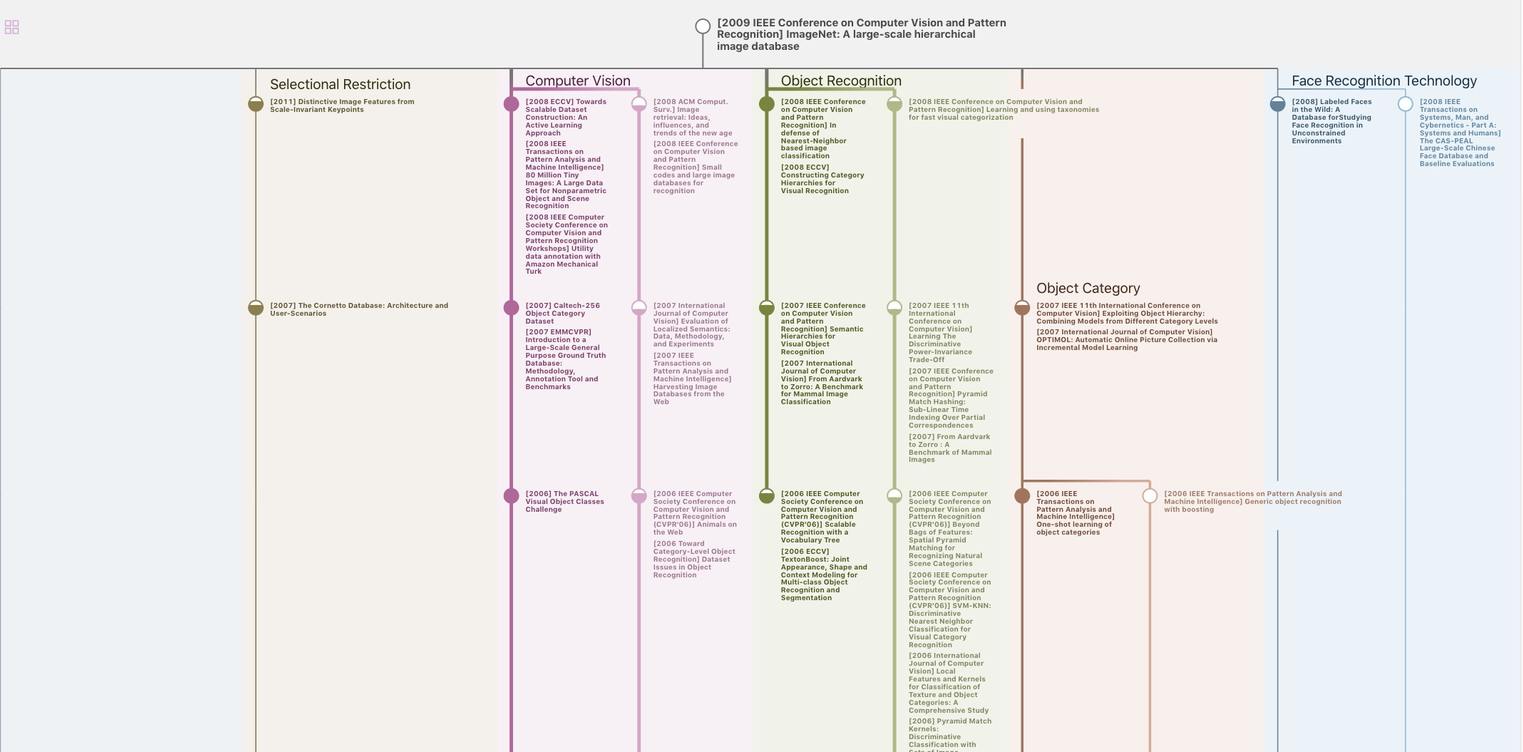
生成溯源树,研究论文发展脉络
Chat Paper
正在生成论文摘要