Deep Q-network Based Dynamic Power Allocation for Cell-Free Massive MIMO
IEEE International Workshop on Computer Aided Modeling and Design of Communication Links and Networks(2021)
摘要
Numerical optimization has been investigated for decades to address complex problems. Many effective methods, e.g., the weighted minimum mean square error (WMMSE) algorithm, have been developed for a large variety of applications in wireless communication systems. However, these methods often require high computational cost creating a serious gap between theoretical analysis and real-time processing. Recently data-driven methods have attracted a lot of attention due to their near-optimal performance with affordable computational cost. Deep Q-network (DQN) is one of the most promising optimization techniques for future wireless communication systems. In this paper, we investigate the DQN method to allocate the downlink transmission power in cell-free (CF) massive multiple-input multiple-output (MIMO) systems. We consider the sum spectral efficiency (SE) optimization problem for systems with mobile user equipment (UEs). The DQN is trained by the rewards of trial-and-error interactions with the environment over time. It takes as input the long-term fading information and it outputs the downlink transmission power values. The numerical results, obtained from a particular 3GPP scenario, show that the DQN outperforms WMMSE in terms of sum-SE and has a less execution time.
更多查看译文
关键词
Cell-free massive MIMO,deep reinforcement learning,power allocation
AI 理解论文
溯源树
样例
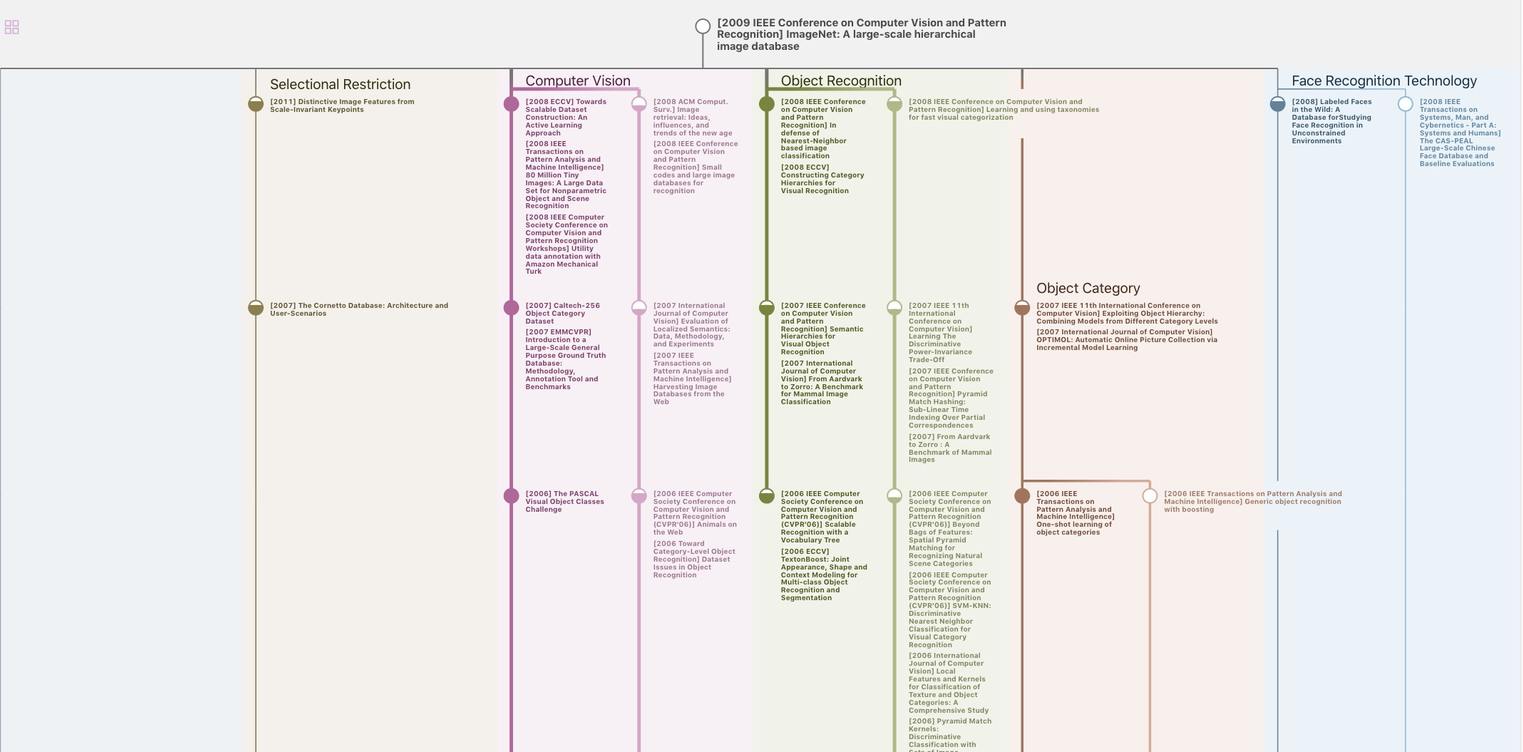
生成溯源树,研究论文发展脉络
Chat Paper
正在生成论文摘要