Impact of Image Pre-Processing Methods on Computed Tomography Radiomics Features in Chronic Obstructive Pulmonary Disease.
PHYSICS IN MEDICINE AND BIOLOGY(2021)
摘要
Computed tomography (CT) imaging texture-based radiomics analysis can be used to assess chronic obstructive pulmonary disease (COPD). However, different image pre-processing methods are commonly used, and how these different methods impact radiomics features and lung disease assessment, is unknown. The purpose of this study was to develop an image pre-processing pipeline to investigate how various pre-processing combinations impact radiomics features and their use for COPD assessment. Spirometry and CT images were obtained from the multi-centered Canadian Cohort of Obstructive Lung Disease study. Participants were divided based on assessment site and were further dichotomized as No COPD or COPD within their participant groups. An image pre-processing pipeline was developed, calculating 32 grey level co-occurrence matrix radiomics features. The pipeline included lung segmentation, airway segmentation or no segmentation, image resampling or no resampling, and either no pre-processing, binning, edgmentation, or thresholding pre-processing techniques. A three-way analysis of variance was used for method comparison. A nested 10-fold cross validation using logistic regression and multiple linear regression models were constructed to classify COPD and assess correlation with lung function, respectively. Logistic regression performance was evaluated using the area under the receiver operating characteristic curve (AUC). A total of 1210 participants (Sites 1-8: No COPD: n = 447, COPD: n = 413; and Site 9: No COPD: n = 155, COPD: n = 195) were evaluated. Between the two participant groups, at least 16/32 features were different between airway segmentation/no segmentation (P <= 0.04), at least 29/32 features were different between no resampling/resampling (P <= 0.04), and 32/32 features were different between the pre-processing techniques (P < 0.0001). Features generated using the resampling/edgmentation and resampling/thresholding pre-processing combinations, regardless of airway segmentation, performed the best in COPD classification (AUC >= 0.718), and explained the most variance with lung function (R (2) >= 0.353). Therefore, the image pre-processing methods completed prior to CT radiomics feature extraction significantly impacted extracted features and their ability to assess COPD.
更多查看译文
关键词
computed tomography,chronic obstructive pulmonary disease,image pre-processing,radiomics analysis
AI 理解论文
溯源树
样例
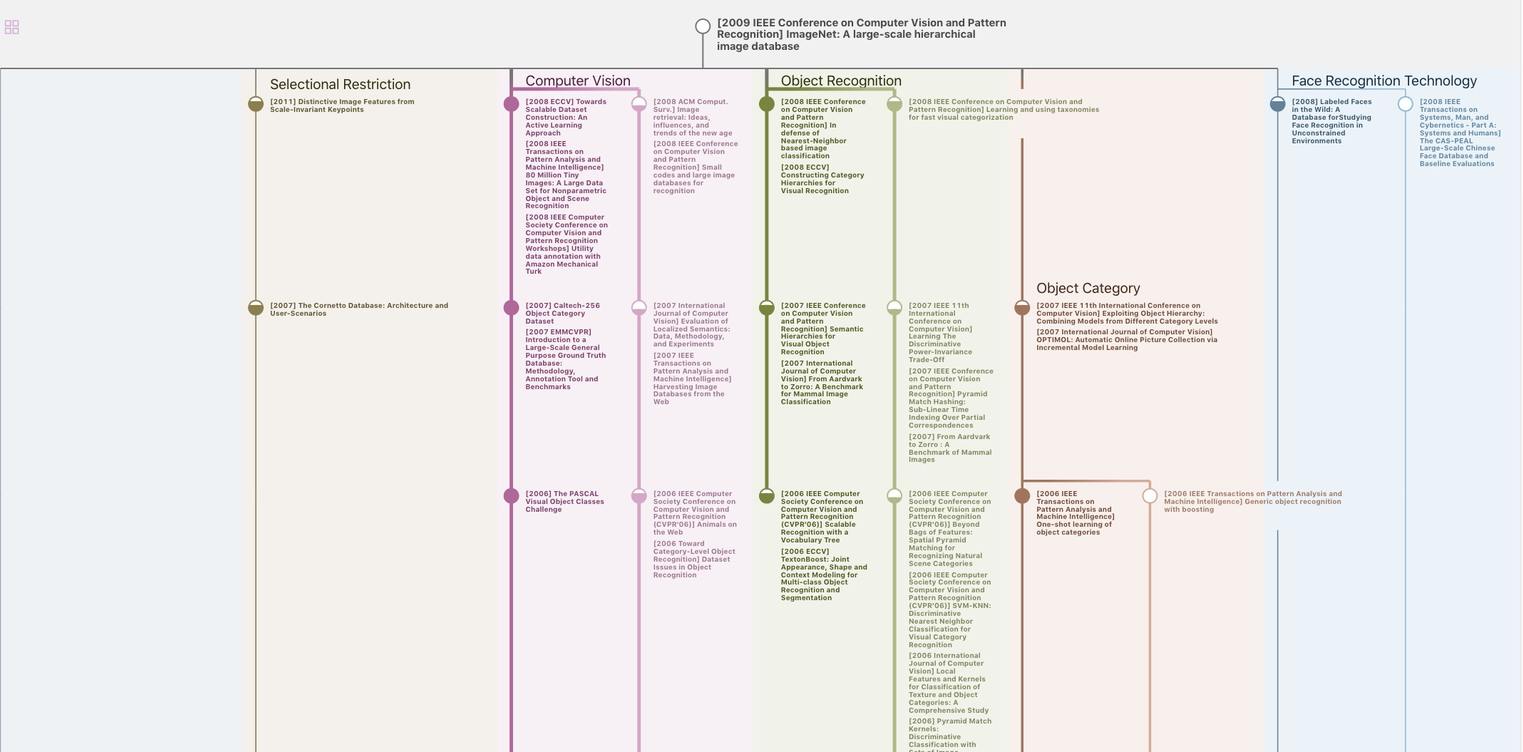
生成溯源树,研究论文发展脉络
Chat Paper
正在生成论文摘要