DeepAoANet: Learning Angle of Arrival From Software Defined Radios With Deep Neural Networks
IEEE ACCESS(2022)
Abstract
Direction finding and positioning systems based on RF signals are significantly impacted by multipath propagation, particularly in indoor environments. Existing algorithms (e.g MUSIC) perform poorly in resolving Angle of Arrival (AoA) in the presence of multipath or when operating in a weak signal regime. We note that digitally sampled RF frontends allow for the easy analysis of signals, and their delayed components. Low-cost Software-Defined Radio (SDR) modules enable Channel State Information (CSI) extraction across a wide spectrum, motivating the design of an enhanced AoA solution. We propose a Deep Learning approach for deriving AoA from a single snapshot of the SDR multichannel data. We compare and contrast deep-learning based angle classification and regression models, to estimate up to two AoAs accurately. We have implemented the inference engines on different platforms to extract AoAs in real-time, demonstrating the computational tractability of our approach. To demonstrate the utility of our approach we have collected IQ (In-phase and Quadrature components) samples from a four-element Universal Linear Array (ULA) in various Line-of-Sight (LOS) and Non-Line-of-Sight (NLOS) environments, and published the dataset. Our proposed method demonstrates excellent reliability in determining number of impinging signals and realized mean absolute AoA errors less than 2 degrees.
MoreTranslated text
Key words
Covariance matrices, Multiple signal classification, Deep learning, Signal to noise ratio, Training, Support vector machines, Software radio, Angle-of-Arrival, deep neural networks, machine learning, signal processing, software defined radio
AI Read Science
Must-Reading Tree
Example
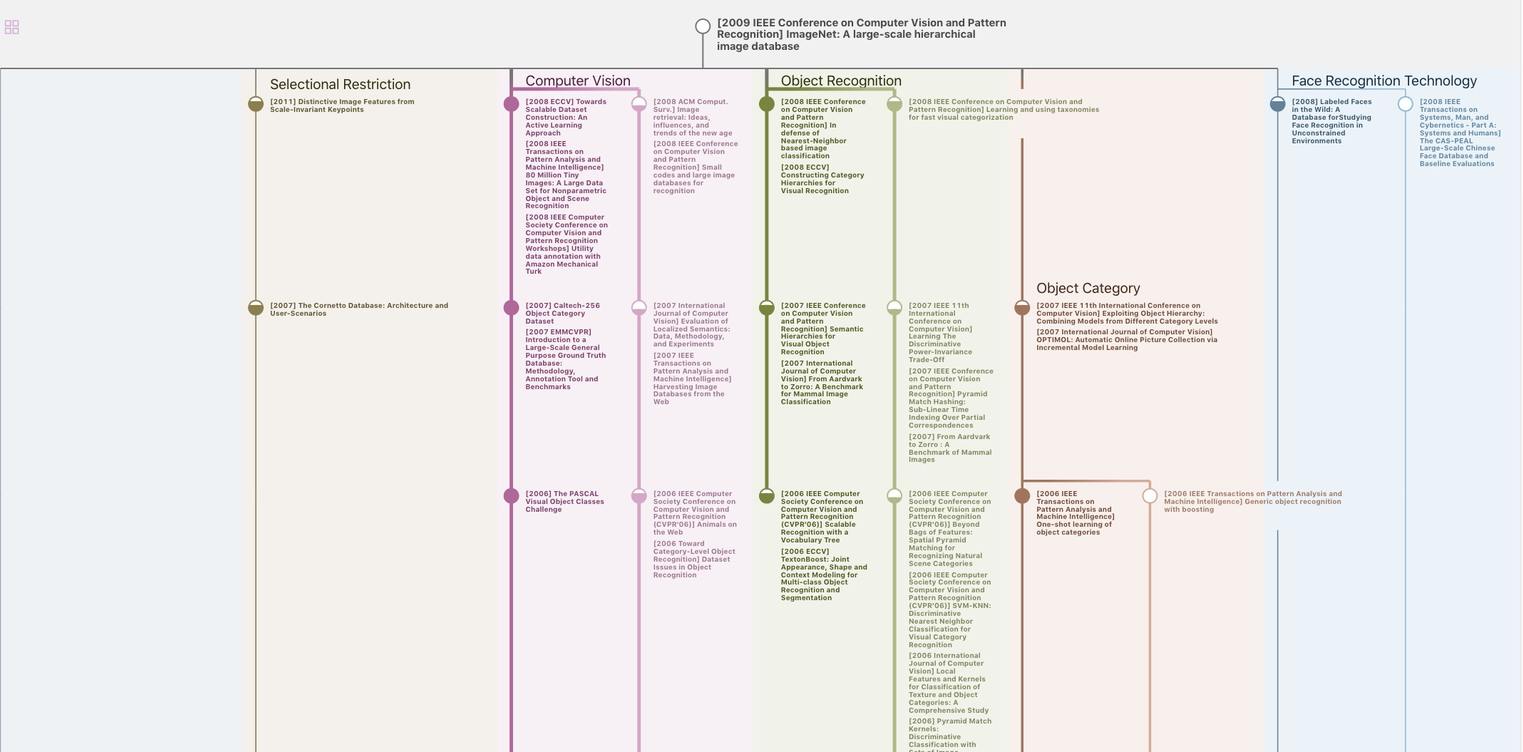
Generate MRT to find the research sequence of this paper
Chat Paper
Summary is being generated by the instructions you defined