The Majority Can Help the Minority: Context-rich Minority Oversampling for Long-tailed Classification
IEEE Conference on Computer Vision and Pattern Recognition(2022)
摘要
The problem of class imbalanced data is that the gener-alization performance of the classifier deteriorates due to the lack of data from minority classes. In this paper, we pro-pose a novel minority over-sampling method to augment di-versified minority samples by leveraging the rich context of the majority classes as background images. To diversify the minority samples, our key idea is to paste an image from a minority class onto rich-context images from a majority class, using them as background images. Our method is simple and can be easily combined with the existing long-tailed recognition methods. We empirically prove the effectiveness of the proposed oversampling method through extensive experiments and ablation studies. Without any architectural changes or complex algorithms, our method achieves state-of-the-art performance on various long-tailed classification benchmarks. Our code is made available at https://github.com/naver-ai/cmo.
更多查看译文
关键词
Transfer/low-shot/long-tail learning, Recognition: detection,categorization,retrieval
AI 理解论文
溯源树
样例
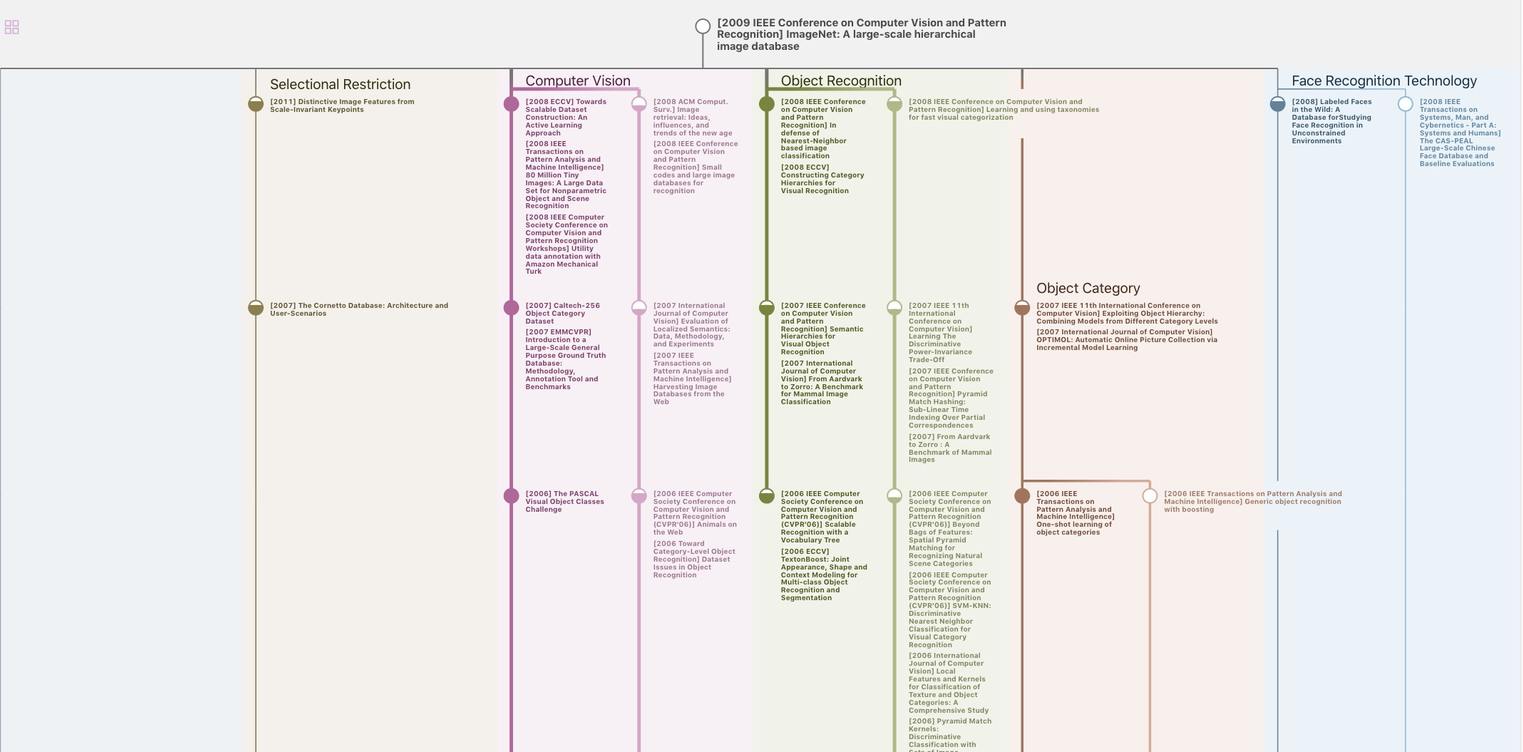
生成溯源树,研究论文发展脉络
Chat Paper
正在生成论文摘要