Joint distribution between rankings (leader and metric based) and metric values
user-607cde9d4c775e0497f57189(2019)
关键词
Metric (mathematics),Joint probability distribution,Mathematical optimization,Mathematics
AI 理解论文
溯源树
样例
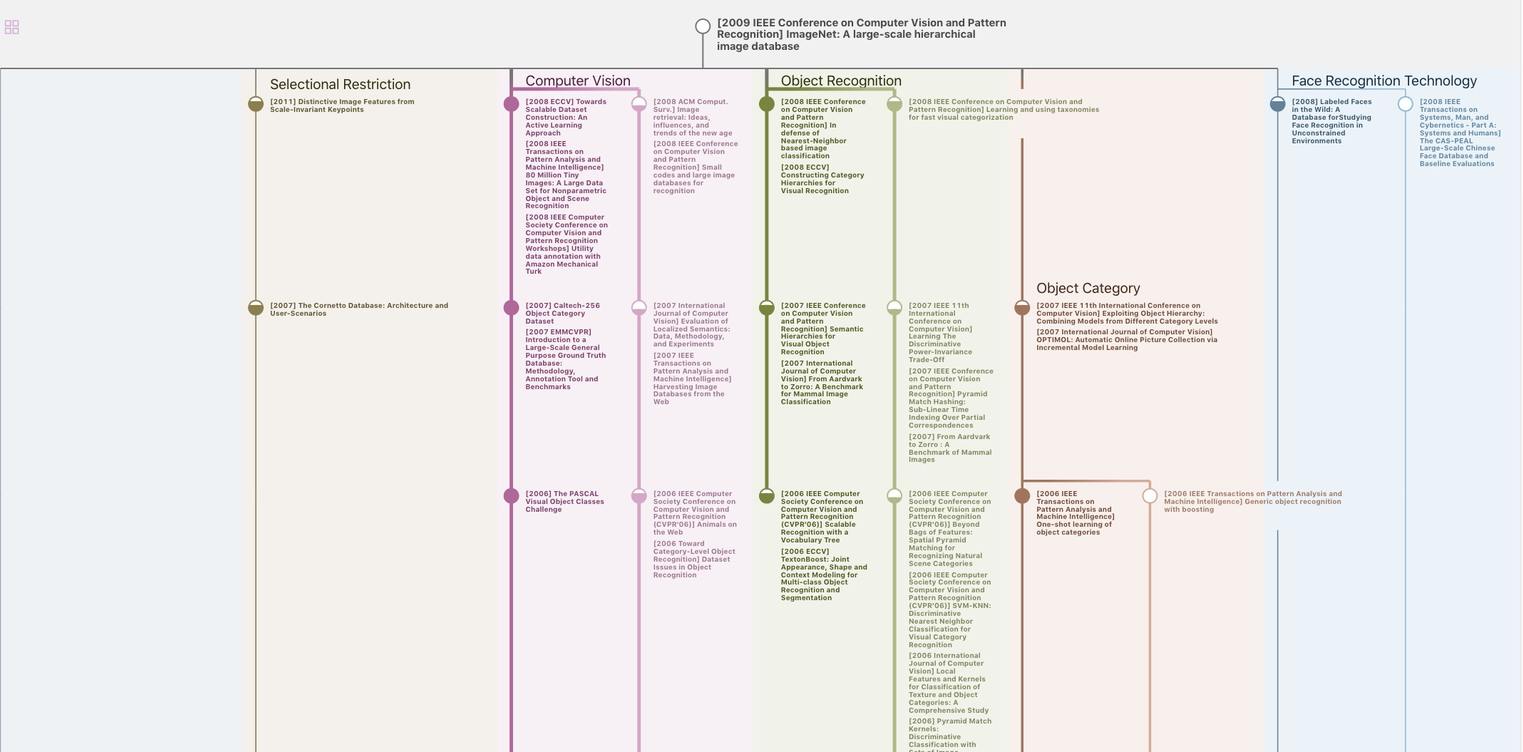
生成溯源树,研究论文发展脉络
Chat Paper
正在生成论文摘要