Hierarchical Relation Networks: Exploiting Categorical Structure in Neural Relational Reasoning
PROCEEDINGS OF THE 13TH INTERNATIONAL JOINT CONFERENCE ON COMPUTATIONAL INTELLIGENCE (IJCCI)(2021)
摘要
Organizing objects in the world into conceptual hierarchies is a key part of human cognition and general intelligence. It allows us to efficiently reason about complex and novel situations relying on relationships between object categories and hierarchies. Learning relationships among sets of objects from data is known as relation learning. Recent developments in this area using neural networks have enabled answering complex questions posed on sets of objects. Previous approaches operate directly on objects - instead of categories of objects. In this position paper, we make the case for reasoning at the level of object categories, and we propose the Hierarchical Relation Network (HRN) framework. HRNs first infer a category for each object to drastically decrease the number of relationships that need to be learned. An HRN consists of a number of distinct modules, each of which can be initialized as a simple arithmetic operation, a supervised or unsupervised model, or as part of a fully differentiable network. This approach demonstrates that categories in relational reasoning can allow for major reductions in training time, increased data efficiency. and better interpretability of the network's reasoning process.
更多查看译文
关键词
Relational Reasoning,Categorization,Hierarchy Learning
AI 理解论文
溯源树
样例
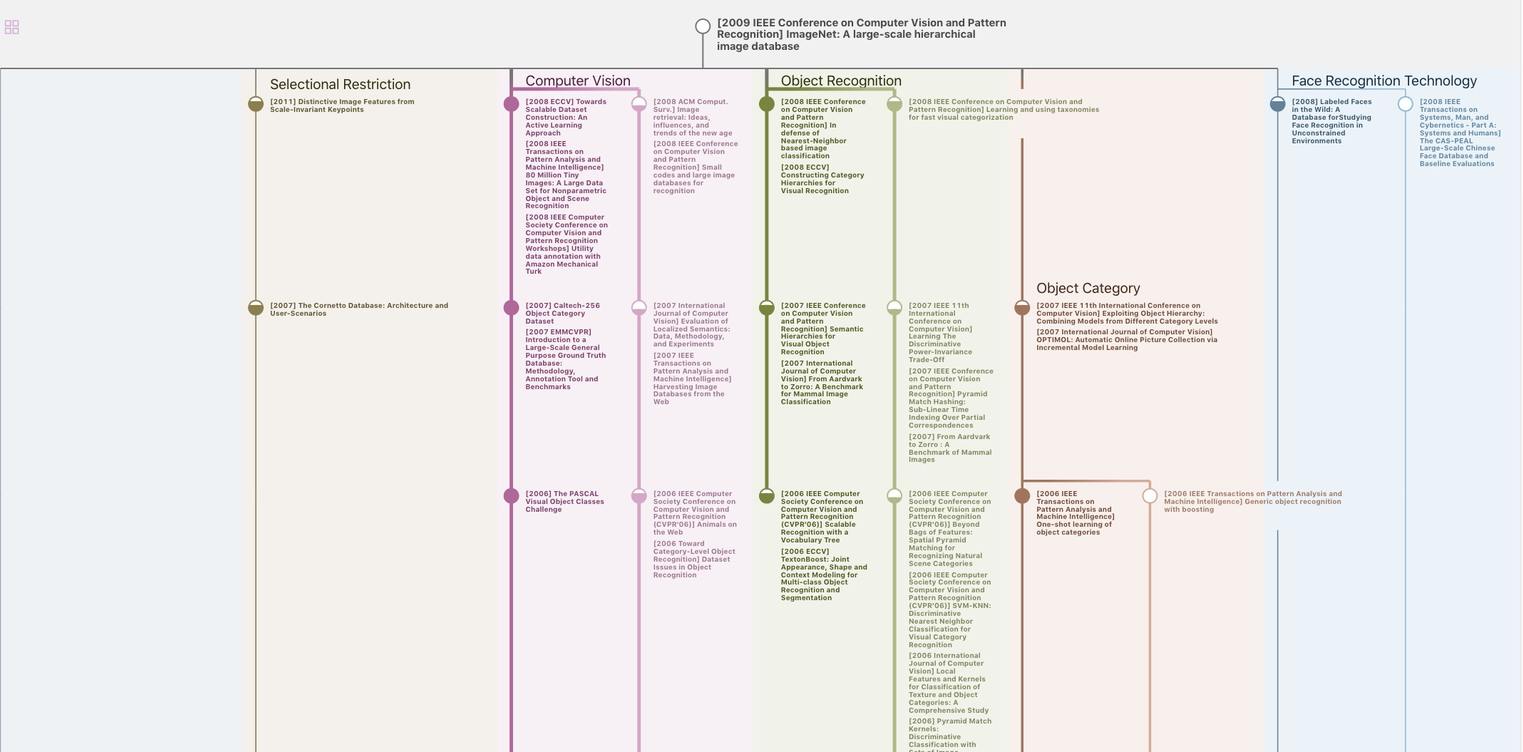
生成溯源树,研究论文发展脉络
Chat Paper
正在生成论文摘要