Scene Representation Transformer: Geometry-Free Novel View Synthesis Through Set-Latent Scene Representations
IEEE Conference on Computer Vision and Pattern Recognition(2022)
摘要
A classical problem in computer vision is to infer a 3D scene representation from few images that can be used to render novel views at interactive rates. Previous work focuses on reconstructing pre-defined 3D representations, e.g. textured meshes, or implicit representations, e.g. radiance fields, and often requires input images with precise camera poses and long processing times for each novel scene. In this work, we propose the Scene Representation Transformer (SRT), a method which processes posed or unposed RGB images of a new area, infers a “set-latent scene representation ”, and synthesises novel views, all in a single feed-forward pass. To calculate the scene representation, we propose a generalization of the Vision Transformer to sets of images, enabling global information integration, and hence 3D reasoning. An efficient decoder transformer parameterizes the light field by attending into the scene representation to render novel views. Learning is supervised end-to-end by minimizing a novel-view reconstruction error. We show that this method outperforms recent baselines in terms of PSNR and speed on synthetic datasets, including a new dataset created for the paper. Further, we demonstrate that SRT scales to support interactive visualization and semantic segmentation of real-world outdoor environments using Street View imagery.
更多查看译文
关键词
3D from multi-view and sensors, 3D from single images, Deep learning architectures and techniques, Machine learning
AI 理解论文
溯源树
样例
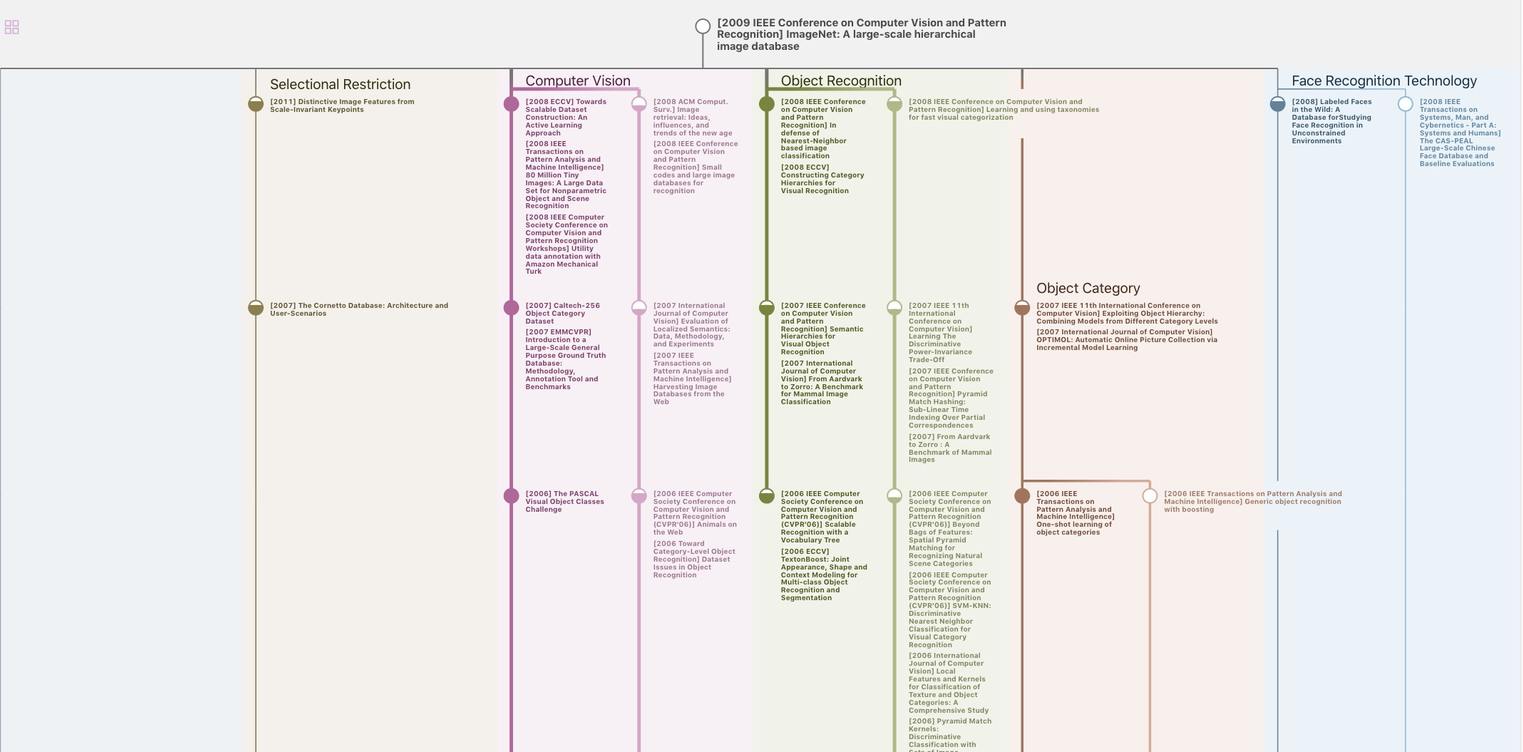
生成溯源树,研究论文发展脉络
Chat Paper
正在生成论文摘要