Readmission Prediction with Knowledge Graph Attention and RNN-Based Ordinary Differential Equations
KNOWLEDGE SCIENCE, ENGINEERING AND MANAGEMENT, PT III(2021)
摘要
Predicting the readmission risk within 30 days on the Electronic Health Record (EHR) has been proven crucial for predictive analytics in healthcare domain. Deep-learning-based models are recently utilized to address this task since those models can relatively improve prediction performance and work as decision aids, which helps reduce unnecessary readmission and recurrence risk. However, existing prediction models, limited by fuzzy relevance of patient data, are unable to get higher prediction accuracy due to data noise generated by patients with different disease types. To solve this problem, we propose an end-to-end model called GROM, which integrates knowledge graph to alleviate the interference of data noise generated in the processing of irregularity dynamic clinical data with neural ordinary differential equation (ODE). The experimental results show that our model achieved the highest average precision and proved that the graph attention mechanism is suitable to improve performance of predicting the risk of readmission within 30 days.
更多查看译文
关键词
Deep learning,Knowledge graph,Electronic health record,ICU Readmission Prediction
AI 理解论文
溯源树
样例
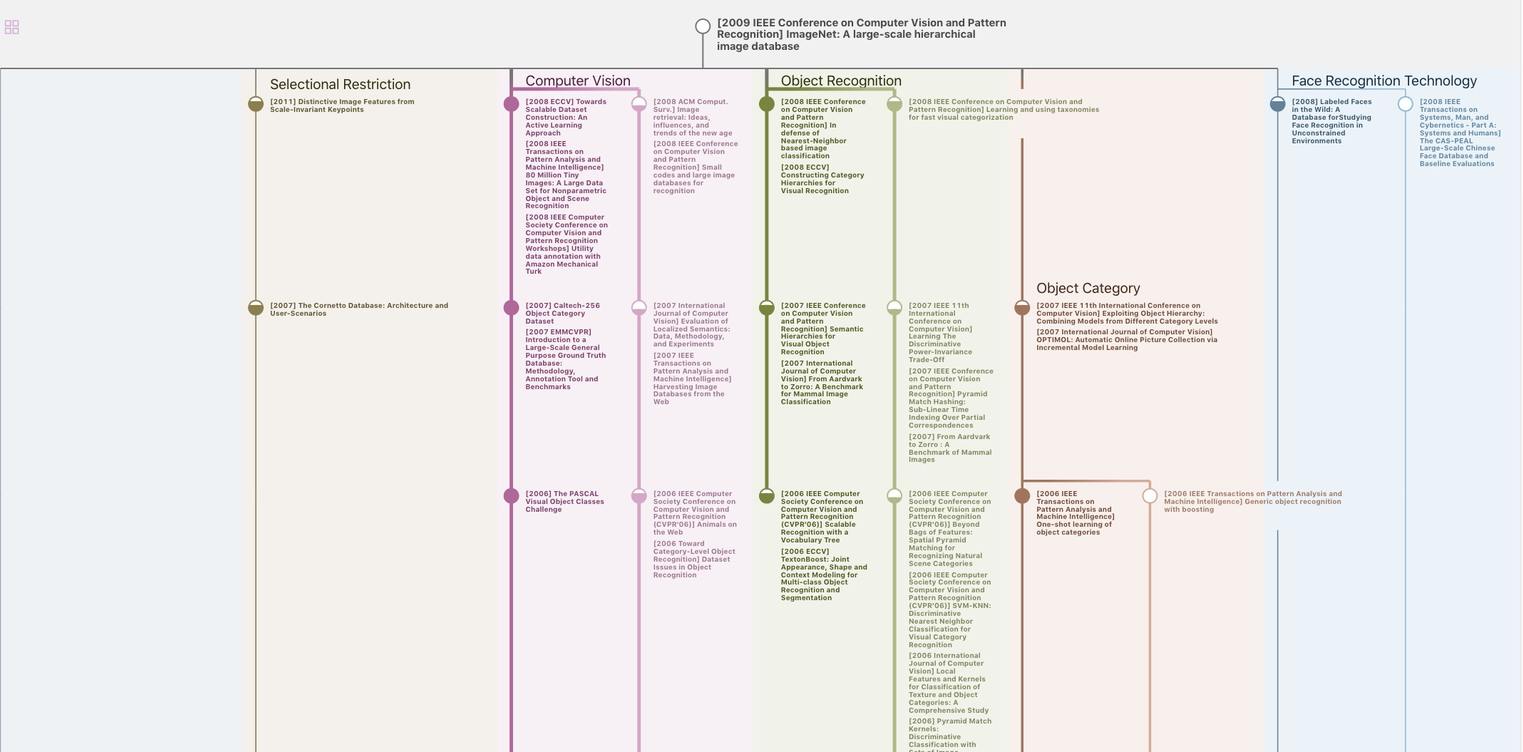
生成溯源树,研究论文发展脉络
Chat Paper
正在生成论文摘要