Distilling the Knowledge in Data Pruning
arXiv (Cornell University)(2024)
摘要
With the increasing size of datasets used for training neural networks, datapruning becomes an attractive field of research. However, most current datapruning algorithms are limited in their ability to preserve accuracy comparedto models trained on the full data, especially in high pruning regimes. In thispaper we explore the application of data pruning while incorporating knowledgedistillation (KD) when training on a pruned subset. That is, rather thanrelying solely on ground-truth labels, we also use the soft predictions from ateacher network pre-trained on the complete data. By integrating KD intotraining, we demonstrate significant improvement across datasets, pruningmethods, and on all pruning fractions. We first establish a theoreticalmotivation for employing self-distillation to improve training on pruned data.Then, we empirically make a compelling and highly practical observation: usingKD, simple random pruning is comparable or superior to sophisticated pruningmethods across all pruning regimes. On ImageNet for example, we achievesuperior accuracy despite training on a random subset of only 50Additionally, we demonstrate a crucial connection between the pruning factorand the optimal knowledge distillation weight. This helps mitigate the impactof samples with noisy labels and low-quality images retained by typical pruningalgorithms. Finally, we make an intriguing observation: when using lowerpruning fractions, larger teachers lead to accuracy degradation, whilesurprisingly, employing teachers with a smaller capacity than the student's mayimprove results. Our code will be made available.
更多查看译文
关键词
Knowledge Discovery
AI 理解论文
溯源树
样例
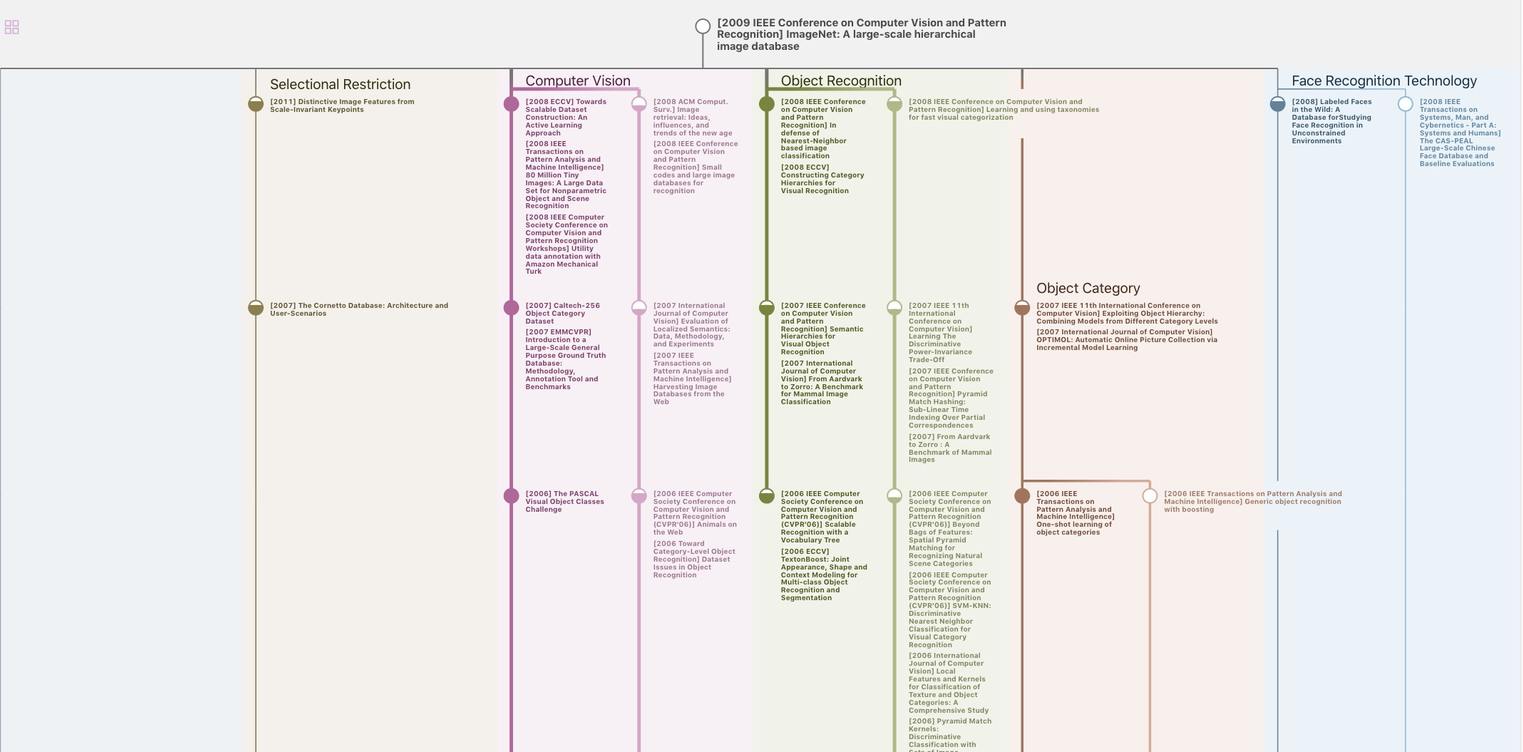
生成溯源树,研究论文发展脉络
Chat Paper
正在生成论文摘要