floodGAN: Using Deep Adversarial Learning to Predict Pluvial Flooding in Real Time
WATER(2021)
摘要
Using machine learning for pluvial flood prediction tasks has gained growing attention in the past years. In particular, data-driven models using artificial neuronal networks show promising results, shortening the computation times of physically based simulations. However, recent approaches have used mainly conventional fully connected neural networks which were (a) restricted to spatially uniform precipitation events and (b) limited to a small amount of input data. In this work, a deep convolutional generative adversarial network has been developed to predict pluvial flooding caused by nonlinear spatial heterogeny rainfall events. The model developed, floodGAN, is based on an image-to-image translation approach whereby the model learns to generate 2D inundation predictions conditioned by heterogenous rainfall distributions-through the minimax game of two adversarial networks. The training data for the floodGAN model was generated using a physically based hydrodynamic model. To evaluate the performance and accuracy of the floodGAN, model multiple tests were conducted using both synthetic events and a historic rainfall event. The results demonstrate that the proposed floodGAN model is up to 10(6) times faster than the hydrodynamic model and promising in terms of accuracy and generalizability. Therefore, it bridges the gap between detailed flood modelling and real-time applications such as end-to-end early warning systems.
更多查看译文
关键词
flood modelling,machine learning,deep learning,generative adversarial networks,real-time flood forecasting
AI 理解论文
溯源树
样例
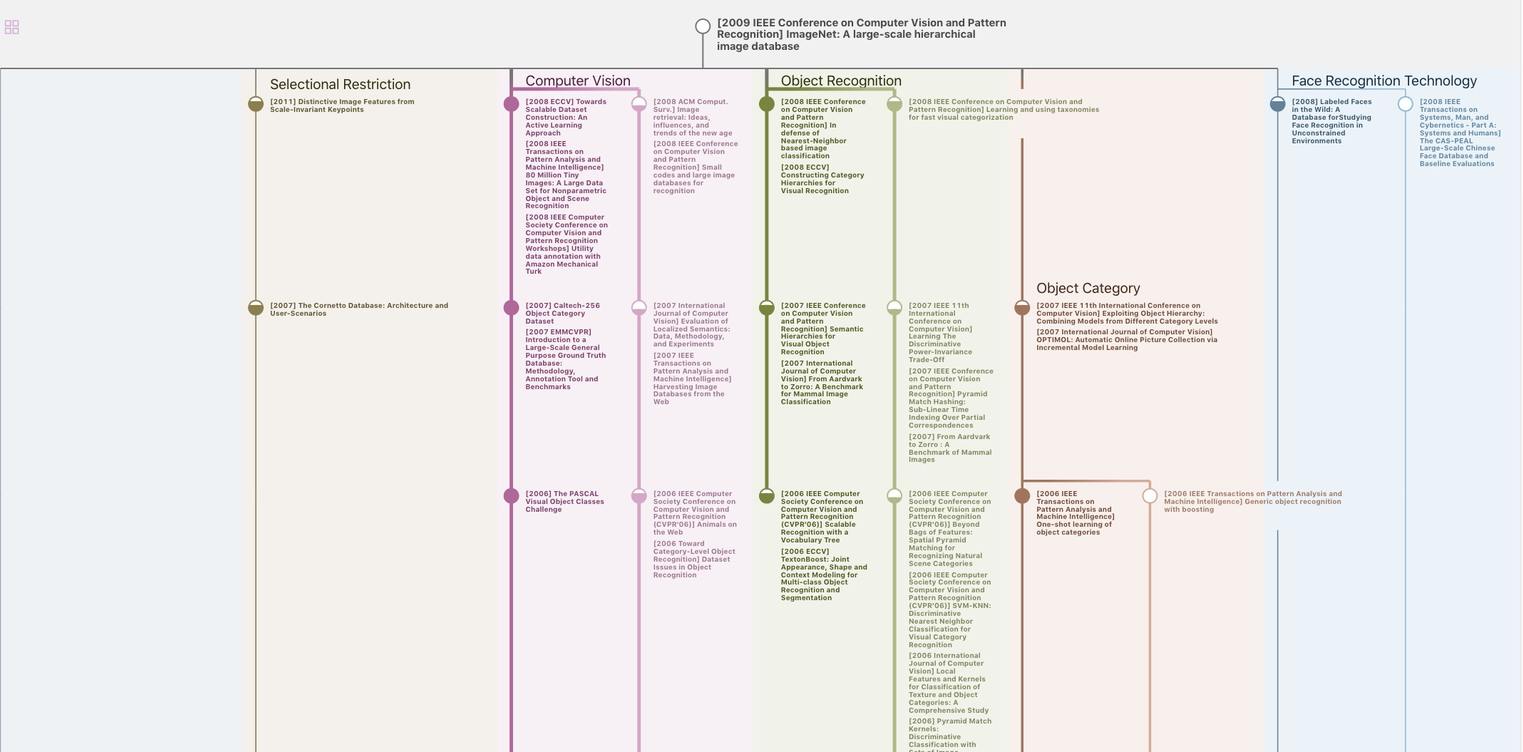
生成溯源树,研究论文发展脉络
Chat Paper
正在生成论文摘要