New Diversity Measures Based on the Coverage and Similarity of the Classification.
Computación y sistemas(2021)
摘要
In supervised pattern classification, it often happens that a single individual classifier is not able to meet the requirements of the problem. This is the main reason that leads to the successful use of systems composed of several classifiers (classifier ensembles) looking to obtain better results than a single classifier. The selection of the classifiers to be used is difficult due to its great variety and to the presence of necessary conditions such as the diversity between them to obtain the best possible results. Specifically, the diversity among the classifiers continues being a crucial factor in these systems and the way of measuring it in an effective way is still an open field. There are different measures in literature that help to decide if a group of classifiers is diverse. Some methods explicitly use these measures to obtain more effective classifier ensembles. The obtained results show it is possible to find combinations of classifiers that assure a superior accuracy compare to the best individual accuracy. In this paper, we propose two new diversity measures based on the coverage and similarity of the classification. Our objective is to measure the diversity in a different way in search of better results. We show several experiments where the behavior of the proposed diversity measures is analyzed. Also, we present the correlation that exists among the propose measures and other measures, including the classifier ensemble accuracy.
更多查看译文
关键词
Diversity measures,classifier ensemble,classifiers
AI 理解论文
溯源树
样例
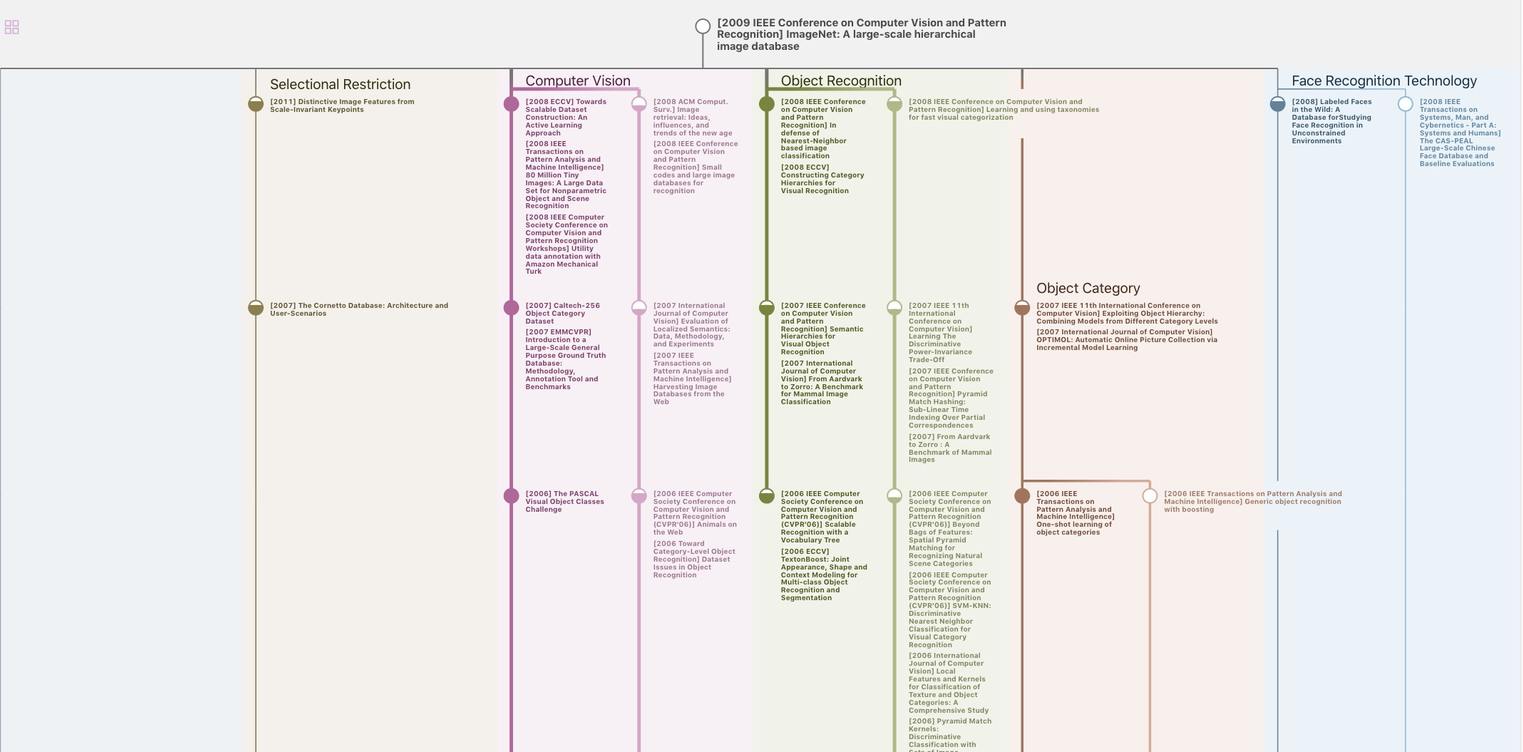
生成溯源树,研究论文发展脉络
Chat Paper
正在生成论文摘要