Comparison of Machine and Deep Learning Methods to Estimate Shrub Willow Biomass from UAS Imagery
CANADIAN JOURNAL OF REMOTE SENSING(2021)
Abstract
Shrub willow is considered an important dedicated energy crop in temperate climates for the production of bioenergy, biofuels, and bio-based products. A methodology to rapidly and accurately estimate above-ground biomass (AGB) is essential for understanding potential biomass supply, identifying potential growth limitations, and making management decisions. The main objective of this study was to investigate different statistical, machine learning, and deep learning models to estimate shrub willow AGB at a site in Camillus, NY using multi-spectral unmanned aerial system (UAS) imagery. The efficiency of the convolutional neural network (CNN) deep learning algorithm was compared to the well-known methods including linear regression, decision tree (DT), random forest (RF), and support vector regression (SVR). The RF model estimated the AGB with the root mean square error (RMSE) of 1.73 Mg/ha and R-2 of 0.95, and outperformed other methods. The next most effective method was CNN with the RMSE of 2.69 Mg/ha and R-2 of 0.89. Feature importance analysis indicated that normalized difference vegetation index (NDVI), ratio vegetation index (RVI), and difference vegetation index (DVI) had the greatest contribution to AGB estimation. This study compared shrub willow AGB estimation models using UAS imagery which will streamline bioenergy/biofuel development compared to the existing methods.
MoreTranslated text
Key words
Biomass Estimation,Tree Height Estimation,Vegetation Monitoring
AI Read Science
Must-Reading Tree
Example
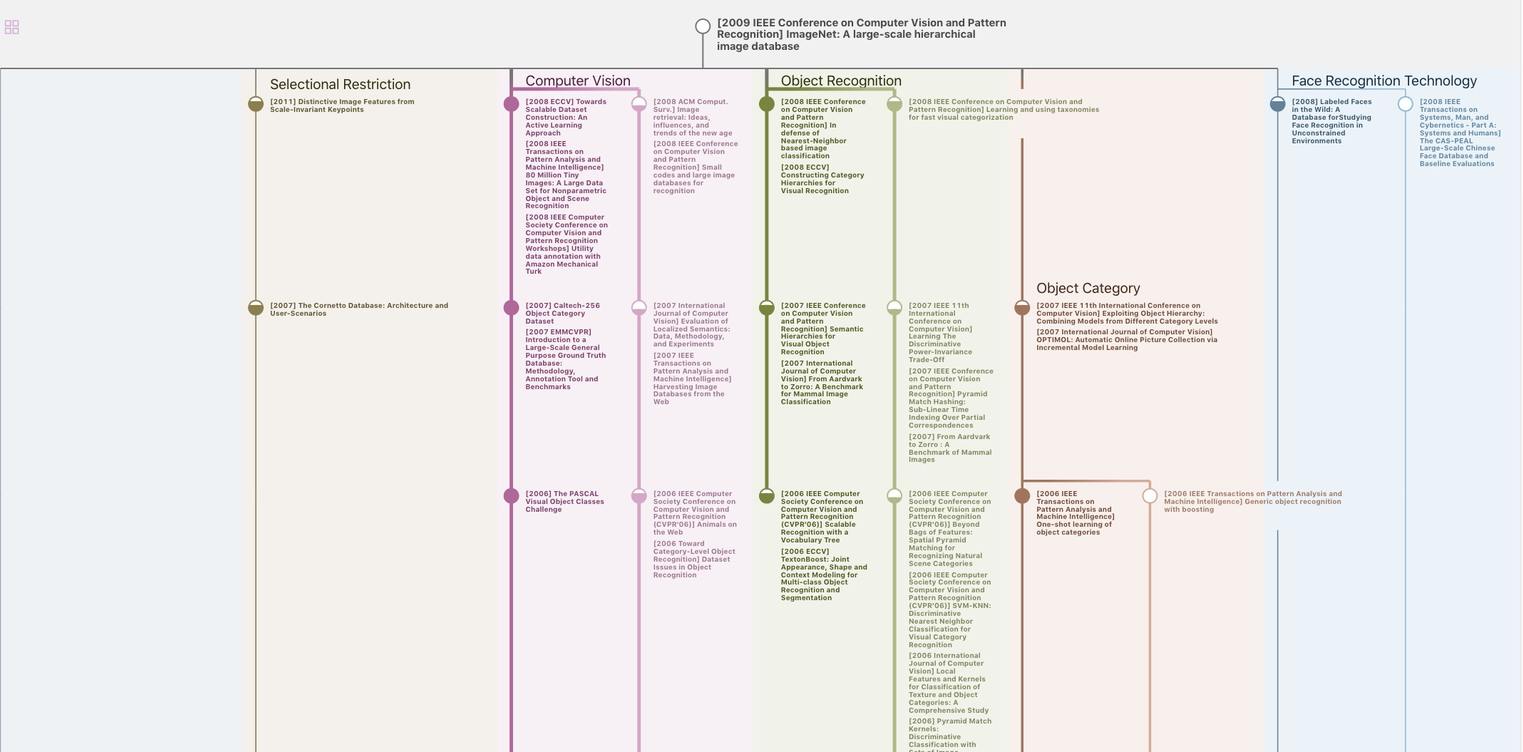
Generate MRT to find the research sequence of this paper
Chat Paper
Summary is being generated by the instructions you defined