Deep-Reinforcement Learning For Fair Distributed Dynamic Spectrum Access In Priority Buffered Heterogeneous Wireless Networks
IET COMMUNICATIONS(2021)
摘要
In this paper, distributed heterogeneous wireless networks are studied and a spectrum access scheme that fairly allocates channels among users based on their request levels is investigated. To be closer to what happens in practice, users' request levels to have different priorities are also considered, i.e. some packets are more important for the user to be transmitted than the others. Due to the distributed nature of the problem and since users are not able to coordinate with each other before transmission, a scheme based on reinforcement learning is proposed in which the network state is estimated based on the previous successful transmissions of packets in the network. The reinforcement learning method's adaptive nature lets our method work in heterogeneous settings where there exist users with other medium access control protocols. The performance of the proposed scheme is evaluated in different network settings, and it is shown that it tries to implement a fair spectrum access policy (considering the packets' priorities) while maximising the total throughput of the network.
更多查看译文
关键词
dynamic spectrum access,deep‐reinforcement,networks
AI 理解论文
溯源树
样例
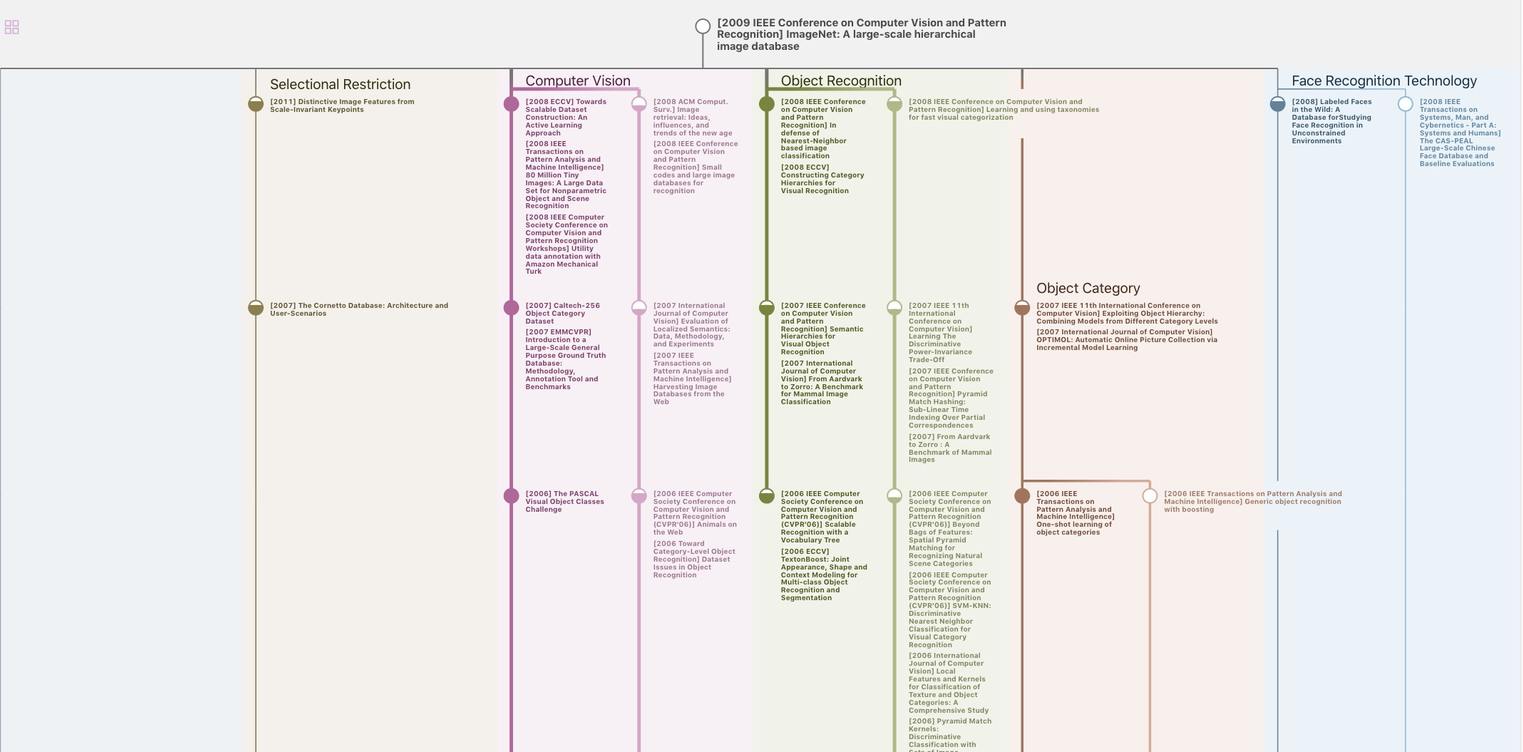
生成溯源树,研究论文发展脉络
Chat Paper
正在生成论文摘要