Towards Risk-aware Machine Learning Supported Model Predictive Control and Open-loop Optimization for Repetitive Processes
IFAC-PapersOnLine(2021)
摘要
Many processes operate repetitively, for example batch processes in biotechnology or chemical engineering. We propose a method for risk-aware run-to-run optimization and model predictive control of repetitive processes with uncertain models. The goal is to increase the performance as the number of runs increases by improving the model despite limited measurements while considering model uncertainty and avoiding uncertain areas. The method uses a gray-box model, i.e. a model formed by a first principle and a machine learning component, in this case an artificial neural network. The model uncertainty might be large, particularly in the first runs, where only a few measurements are available. We propose to quantify this uncertainty using Bayesian inference. This is in turn reflected by a risk measure entering an open-loop optimal control problem and a shrinking-horizon Model Predictive Controller as a constraint to limit control and exploitation in high risk areas. We show that using this risk measure we are able to efficiently reach high process performance. The proposed method is tested in simulations on two biotechnological fed-batch processes.
更多查看译文
关键词
Optimal control,machine learning,Gaussian process,uncertain process,batch-to-batch,run-to-run,biotechnology,predictive control,neural networks
AI 理解论文
溯源树
样例
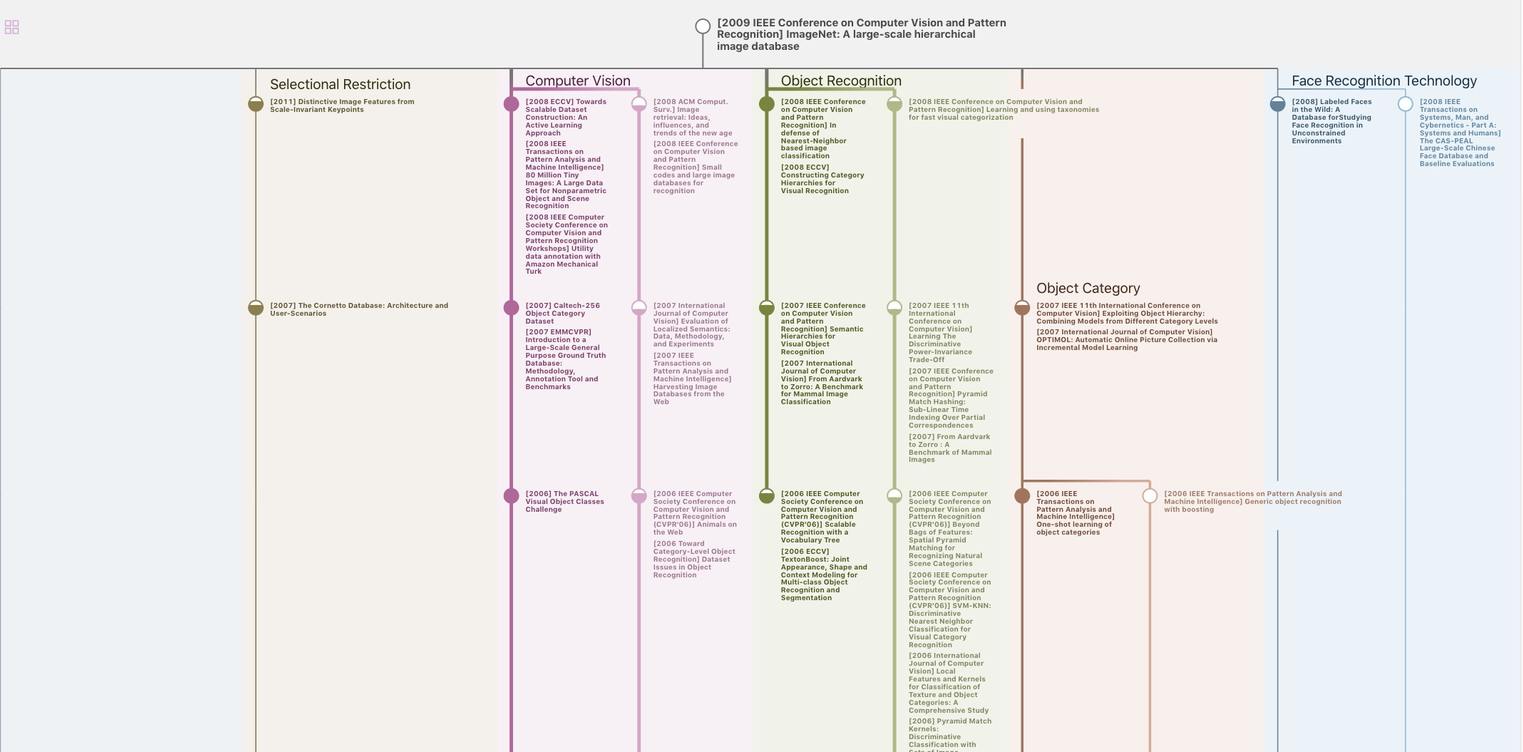
生成溯源树,研究论文发展脉络
Chat Paper
正在生成论文摘要