Enhancing Machine Learning Models for Path Loss Prediction Using Image Texture Techniques
IEEE antennas and wireless propagation letters/Antennas and wireless propagation letters(2021)
摘要
The performance of machine learning (ML)-based path loss models relies heavily on the data they use at their inputs. Feature engineering is, therefore, essential for the model's success. In this letter, at hand, we extract a new set of input features, based on image texture. The image that we use represents the footprint of the urban built-up area, where the gray scale values of the building blocks are analogue to their heights. We extract texture information by applying the segmentation-based fractal texture analysis algorithm on the orthogonal area that is bounded between the transmitter and the receiver. To the best of our knowledge this is the first time that such a technique is applied to a path loss modeling problem in electromagnetics. The algorithm, thus, delivers a new set of features, based on the image's texture, which eventually reveal the built-up profile of the area. These new features are injected to an already existing feature set. Comparative analysis shows that the addition of texture-based features leads to enhanced predictions, for a diverse set of transmitter heights, ML algorithms, and performance metrics.
更多查看译文
关键词
Feature extraction,Gray-scale,Buildings,Transmitters,Predictive models,Receivers,Data models,Image texture,machine learning,mobile communications,path loss prediction
AI 理解论文
溯源树
样例
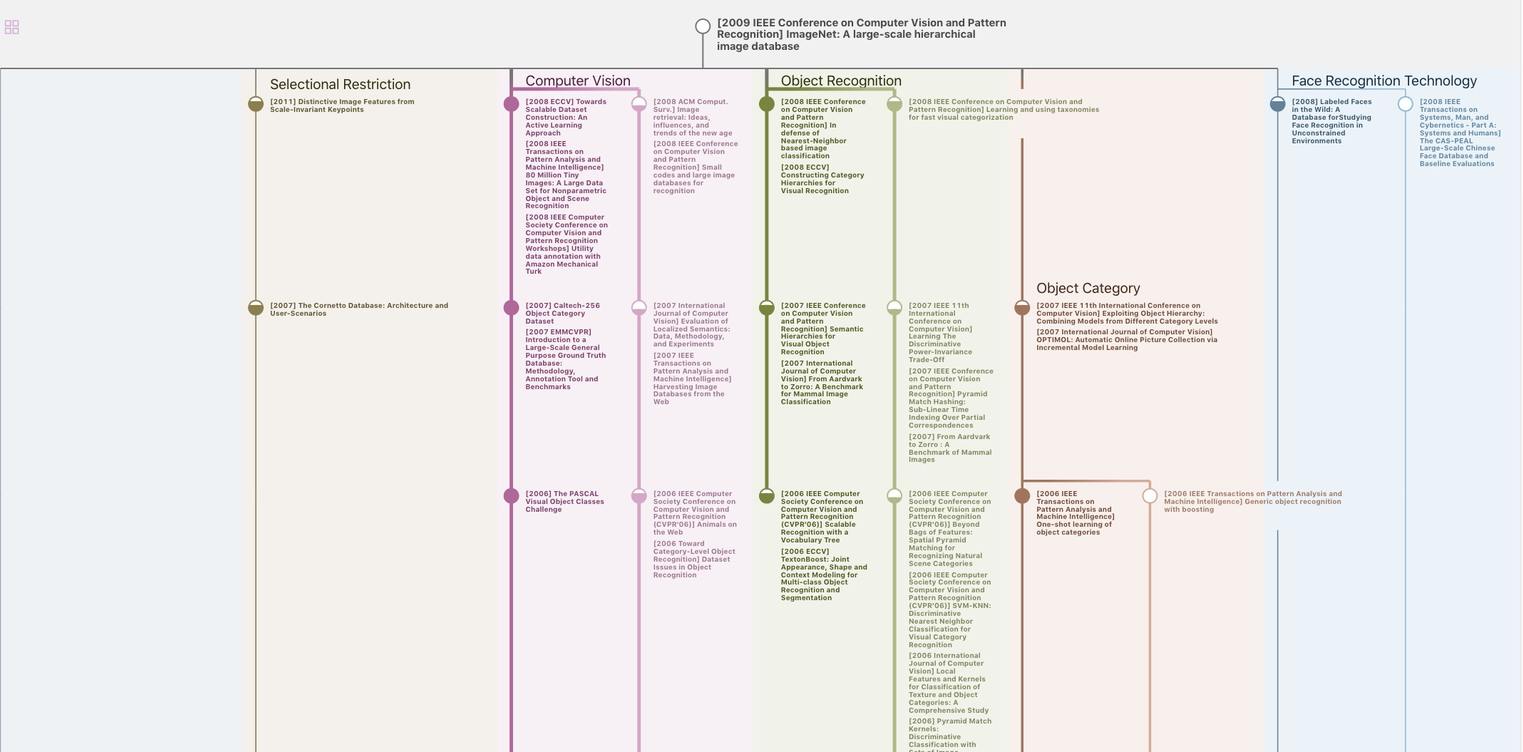
生成溯源树,研究论文发展脉络
Chat Paper
正在生成论文摘要