A General Fully Automated Deep-Learning Method to Detect Cardiomegaly in Chest X-Rays
MEDICAL IMAGING 2021 COMPUTER-AIDED DIAGNOSIS(2021)
摘要
Cardiomegaly is a medical condition that leads to an increase in cardiac size. It can be manually assessed using the cardiothoracic ratio from chest x-rays (CXRs). However, as that task can be challenging in such limited examinations, we propose the fully automated cardiomegaly detection in CXR. For this, we first trained convolutional networks (ConvNets) to classify the CXR as positive or negative to cardiomegaly and then evaluated the generalization potential of the trained ConvNets on independent cohorts. This work used frontal CXR images from a public dataset for training/testing and another public and one private dataset to test the models’ generalization externally. Training and testing were performed using images cropped with a previously developed U-Net model. Experiments were performed with five topologically different ConvNets, data augmentation techniques, and a 50-50 class-weighing strategy to improve performance and reduce the possibility of bias to the majority class. The receiver operating characteristic curve assessed the performance of the models. DenseNet yielded the highest area under the curve (AUC) on testing (0.818) and external validation (0.809) datasets. Moreover, DenseNet obtained the highest sensitivity overall, yielding up to 0.971 on the private dataset with patients from our hospital. Therefore, DenseNet had a statistically higher potential to identify cardiomegaly. The proposed models, especially those trained with DenseNet convolutional core, automatically detected cardiomegaly with high sensitivity. To the best of our knowledge, this was the first work to design a novel general model for classifying specific deep-learning patterns of cardiomegaly in CXRs.
更多查看译文
关键词
Cardiomegaly,radiography,convolutional neural network,medical imaging analysis,deep learning,radiomics
AI 理解论文
溯源树
样例
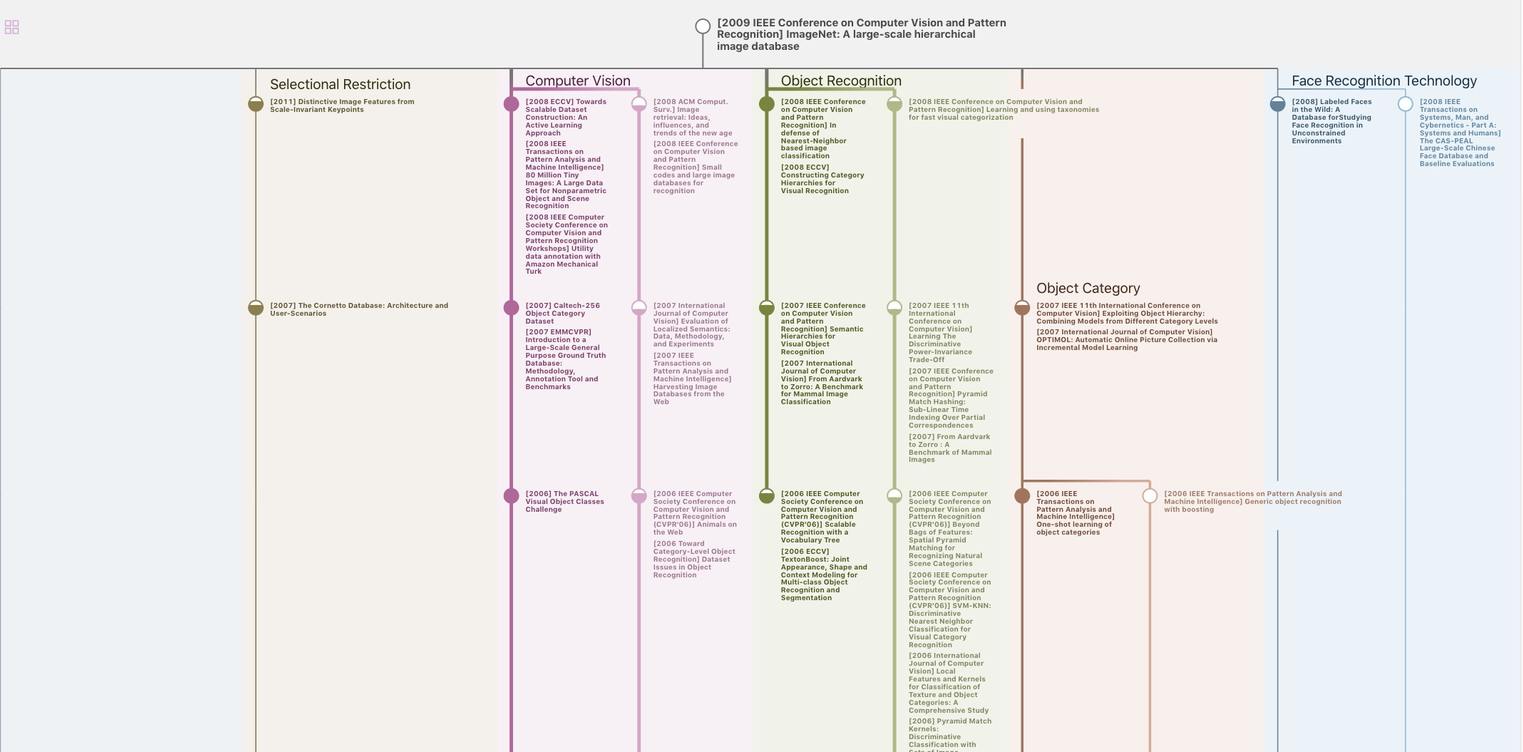
生成溯源树,研究论文发展脉络
Chat Paper
正在生成论文摘要