A Decision-Making Method for Machinery Abnormalities Based on Neural Network Prediction and Bayesian Hypothesis Testing
Electronics(2021)
摘要
For anomaly identification of predicted data in machinery condition monitoring, traditional threshold methods have problems during residual testing. It is difficult to make decisions when the residuals are close to the threshold and fluctuate. This paper proposes a Bayesian dynamic thresholding method that combines Bayesian inference with neural network signal prediction. The method makes full use of historical prior data to build an anomaly identification and warning model applicable under single variable or multidimensional variables. A long short-term memory signal prediction model is established, and then a Bayesian hypothesis testing-based anomaly identification strategy is presented to quantify the probability of anomaly occurrence and issue early warnings for anomalies beyond a certain probability. The model was applied to open data sets of a pumping station and actual operating data of a nuclear power turbine. The results indicate that the model successfully predicts the failure probability and failure time. The effectiveness of the proposed method is verified.
更多查看译文
关键词
Bayesian hypothesis testing,abnormality identification,long short-term memory,nuclear power turbine
AI 理解论文
溯源树
样例
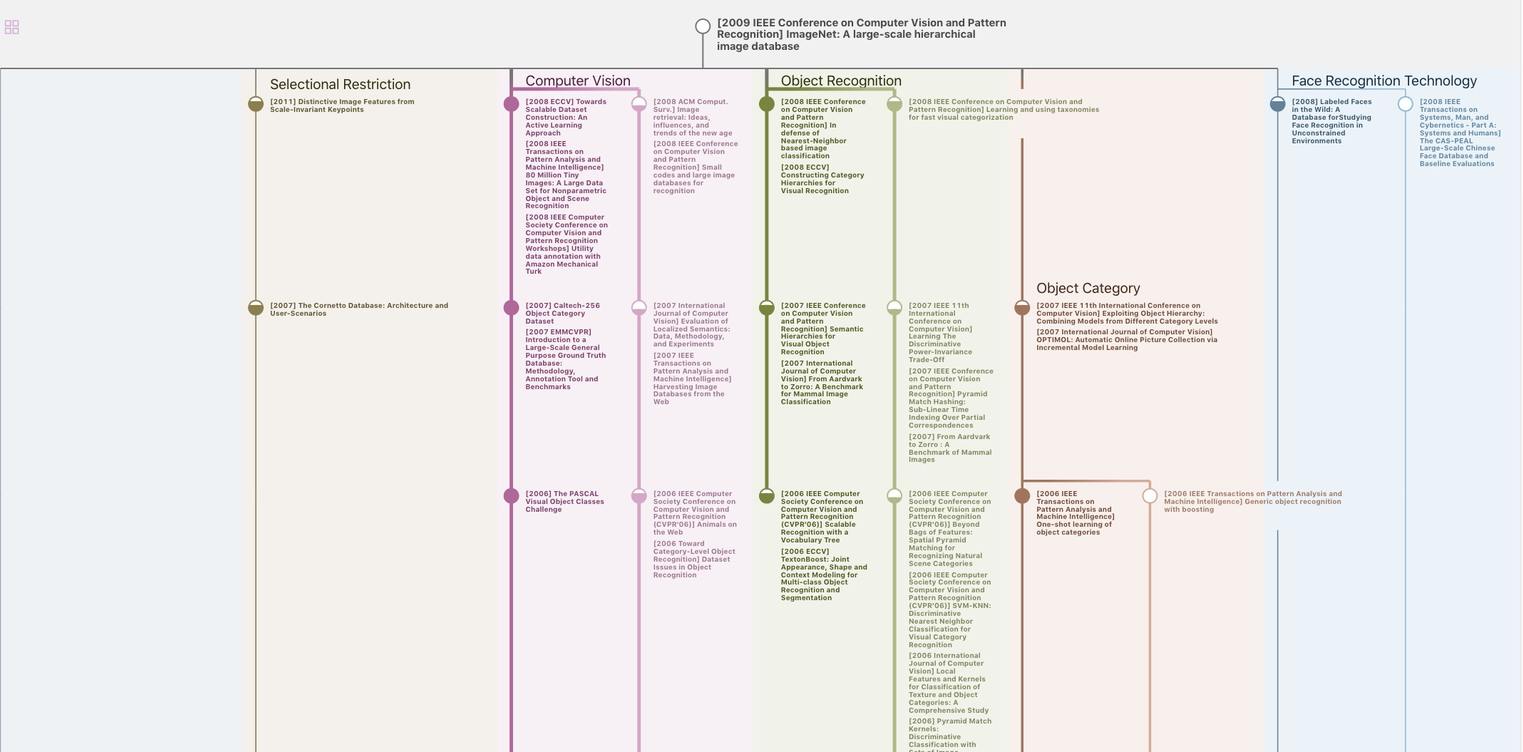
生成溯源树,研究论文发展脉络
Chat Paper
正在生成论文摘要