Metro-Environmental Data Approach For The Prediction Of Chemical Oxygen Demand In New Nicosia Wastewater Treatment Plant
DESALINATION AND WATER TREATMENT(2021)
摘要
This study aimed at employing three data-driven models, namely the Hammerstein-Weiner (HW) model, support vector machine (SVM), and feedforward back propagation neural network (FFBPNN) and traditional multi-linear regression, as well as two non-linear ensemble techniques viz: HW-ensemble and FFBPNN-ensemble, were employed to predict chemical oxygen demand (CODeff). For the prediction of the CODeff, two types of data were used, the first one being environmental data from the new Nicosia waste water treatment plant conductivity (Cond(inf)), including total nitrogen (TNinf), total phosphorus (TPinf) and one-effluent parameter CODeff as M1, where the second was meteorology data from the National Aeronautics and Space Administration (NASA) (at 2 m above the Earth's surface), such as relative humidity (R2H), maximum temperature (T2M_M) and mean temperature (T2M) as M2, in a hybrid model M3, which was a combination of both the meteorology and environmental data M1 and M2. According to the performance criteria RMSE and DC of the single models, values of HW-M1 (0.0308 and 0.9686), HW-M2 (0.0322 and 0.9093) and SVM-M3 (0.025 and 0.9486) were recorded. The ensemble technique improved the performance of the single models in the verification phase by 12% and 19% for HW-E and FFBPNN-E, respectively.
更多查看译文
关键词
Artificial intelligence, Nicosia, Wastewater, Chemical oxygen demand, Ensemble learning
AI 理解论文
溯源树
样例
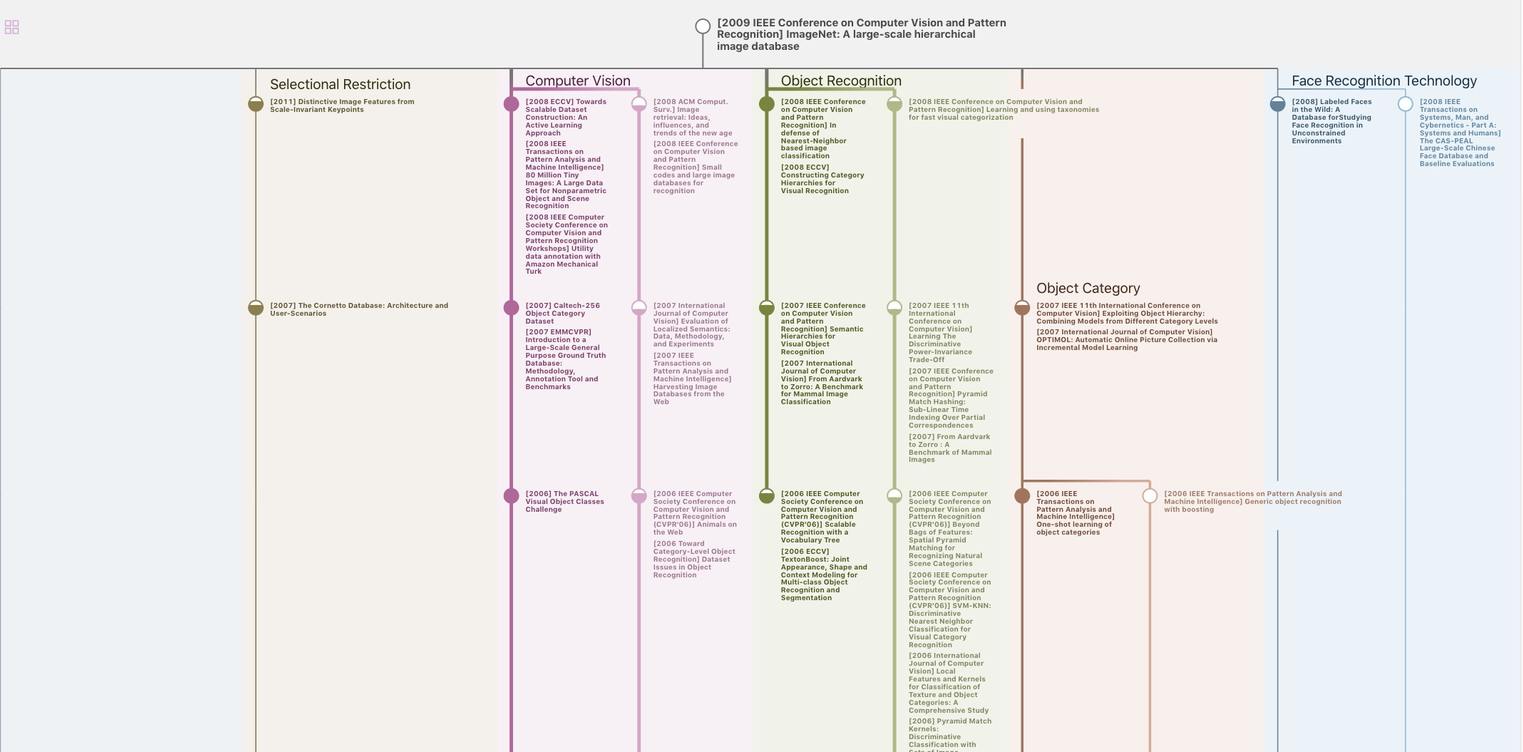
生成溯源树,研究论文发展脉络
Chat Paper
正在生成论文摘要