Dynamic malware attack dataset leveraging virtual machine monitor audit data for the detection of intrusions in cloud
TRANSACTIONS ON EMERGING TELECOMMUNICATIONS TECHNOLOGIES(2022)
摘要
In this new era of cloud computing, Intrusion Detection System (IDS) is very essential for the continual monitoring of computing resources for signs of compromise since the number of attack vectors and malware are in increase. Only few IDS datasets are publicly available and those available are outdated, lack cloud-specific attacks. This article presents a novel dataset based on Virtual Machine Introspected data for the implementation of IDS in cloud. The dataset was generated from the behavioral characteristics of malware and benign sample execution traces on virtual machines using Virtual Machine Introspection (VMI) technique. A vector space model based on system call approach is applied to analyze the behavioral characteristics for the generation of proposed dataset. The purpose of this study is to compare the proposed dataset with existing datasets and evaluate the effectiveness of these datasets by applying Machine Learning (ML) algorithms with 10-fold cross-validation. The ML algorithms used in the experiments are C4.5, Random Forest, JRip, NaiveBayes, K-Nearest Neighbors (KNN), and Support Vector Machine (SVM). The effectiveness of detecting intrusions using proposed dataset is promising compared with other datasets in-terms of intrusion detection accuracy, recall value, precision, and F1-score metrics. For example, the intrusion detection accuracy in proposed dataset is 0.11% improved than UNM dataset, 6.28% higher than ADFA dataset, and 1.88% higher than LID dataset with C4.5 algorithm. Therefore, the proposed dataset is best suitable for implementing IDS for cloud.
更多查看译文
AI 理解论文
溯源树
样例
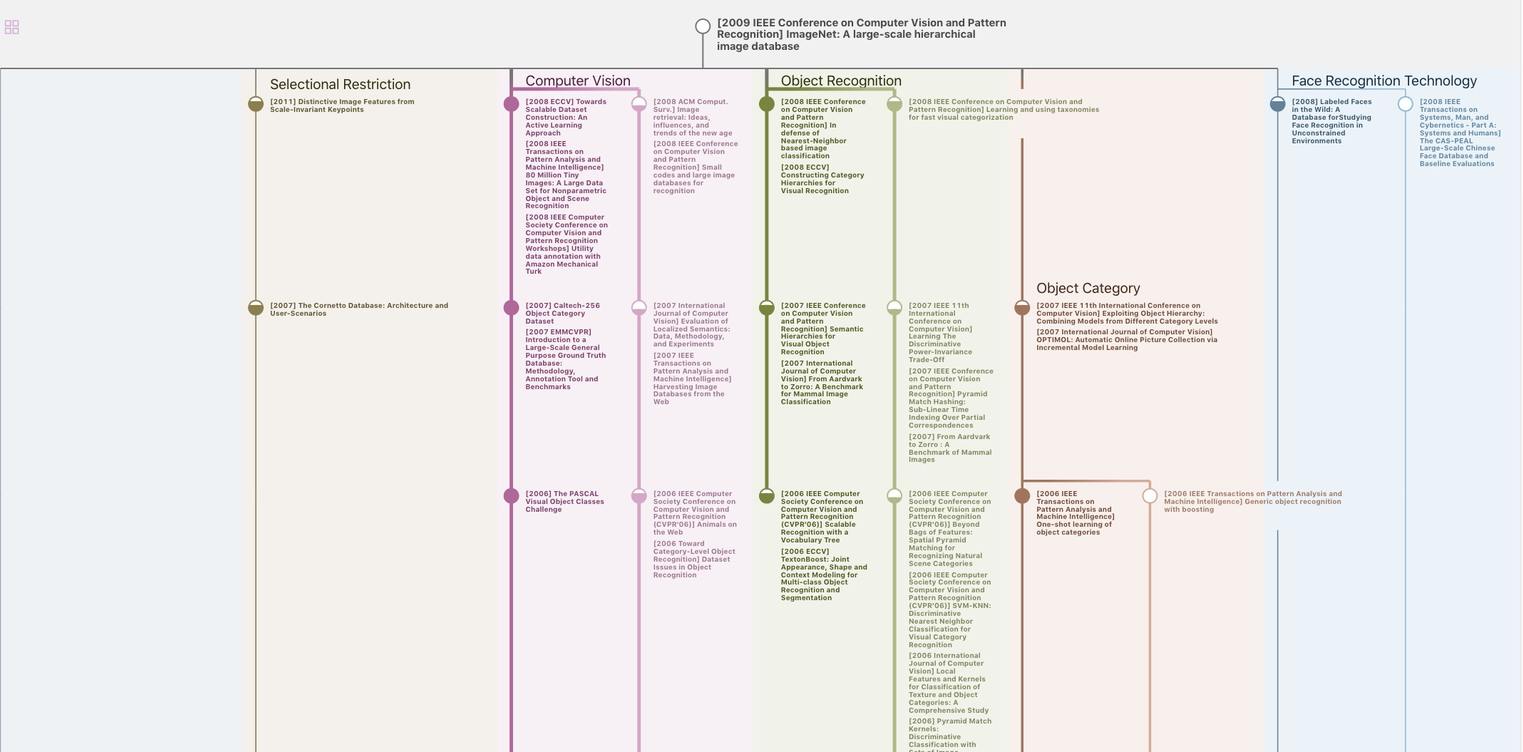
生成溯源树,研究论文发展脉络
Chat Paper
正在生成论文摘要