Machine Learning Can Assign Geologic Basin to Produced Water Samples Using Major Ion Geochemistry
NATURAL RESOURCES RESEARCH(2021)
摘要
Understanding the geochemistry of waters produced during petroleum extraction is essential to informing the best treatment and reuse options, which can potentially be optimized for a given geologic basin. Here, we used the US Geological Survey’s National Produced Waters Geochemical Database (PWGD) to determine if major ion chemistry could be used to classify accurately a produced water sample to a given geologic basin based on similarities to a given training dataset. Two datasets were derived from the PWGD: one with seven features but more samples (PWGD7), and another with nine features but fewer samples (PWGD9). The seven-feature dataset, prior to randomly generating a training and testing (i.e., validation) dataset, had 58,541 samples, 20 basins, and was classified based on total dissolved solids (TDS), bicarbonate (HCO 3 ), Ca, Na, Cl, Mg, and sulfate (SO 4 ). The nine-feature dataset, prior to randomly splitting into a training and testing (i.e., validation) dataset, contained 33,271 samples, 19 basins, and was classified based on TDS, HCO 3 , Ca, Na, Cl, Mg, SO 4 , pH, and specific gravity. Three supervised machine learning algorithms—Random Forest, k-Nearest Neighbors, and Naïve Bayes—were used to develop multi-class classification models to predict a basin of origin for produced waters using major ion chemistry. After training, the models were tested on three different datasets: Validation7, Validation9, and one based on data absent from the PWGD. Prediction accuracies across the models ranged from 23.5 to 73.5% when tested on the two PWGD-based datasets. A model using the Random Forest algorithm predicted most accurately compared to all other models tested. The models generally predicted basin of origin more accurately on the PWGD7-based dataset than on the PWGD9-based dataset. An additional dataset, which contained data not in the PWGD, was used to test the most accurate model; results suggest that some basins may lack geochemical diversity or may not be well described, while others may be geochemically diverse or are well described. A compelling result of this work is that a produced water basin of origin can be determined using major ions alone and, therefore, deep basinal fluid compositions may not be as variable within a given basin as previously thought. Applications include predicting the geochemistry of produced fluid prior to drilling at different intervals and assigning historical produced water data to a producing basin.
更多查看译文
关键词
Machine learning,Produced water,Chemistry,Random Forest,Basinal brines
AI 理解论文
溯源树
样例
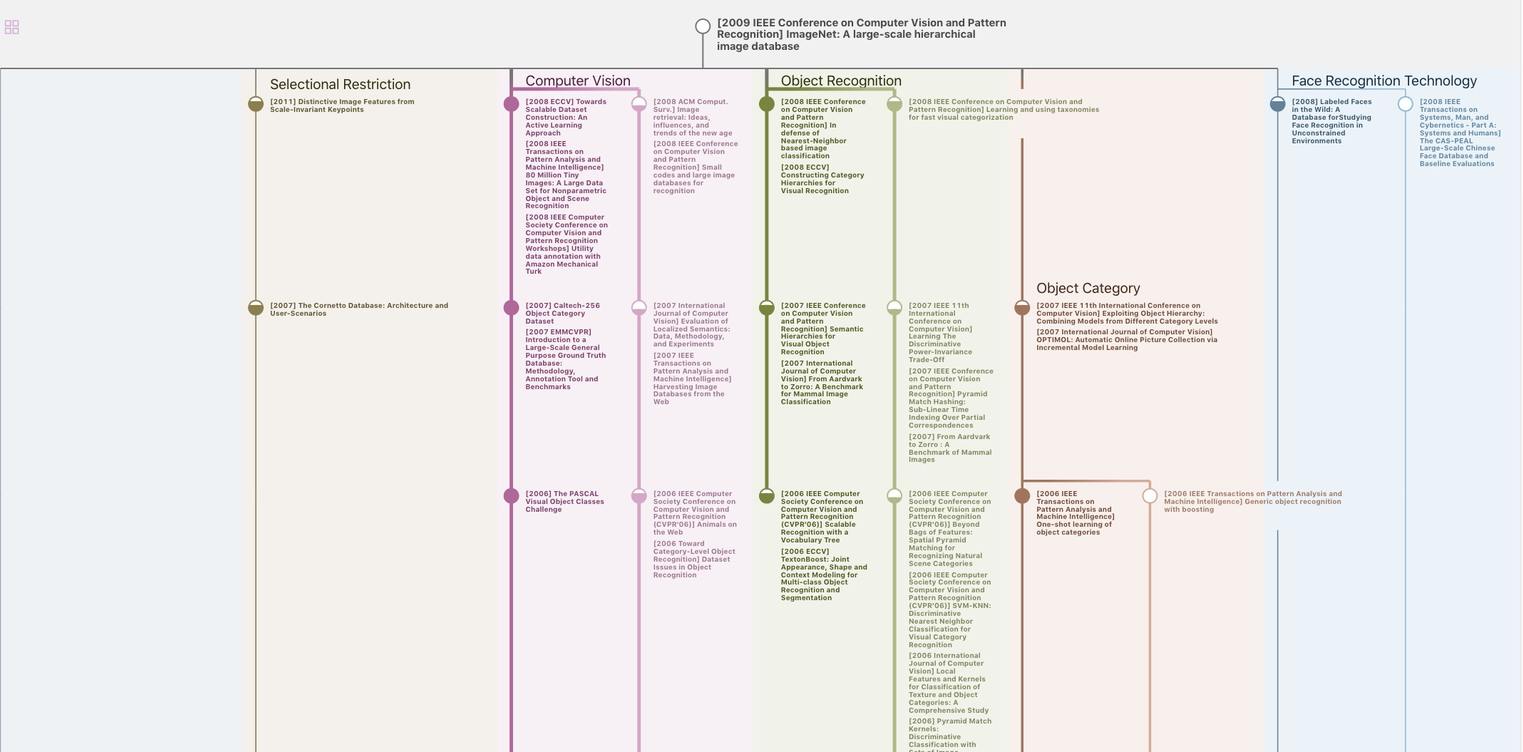
生成溯源树,研究论文发展脉络
Chat Paper
正在生成论文摘要