An Optimal Model Identification for Solid Oxide Fuel Cell Based on Extreme Learning Machines Optimized by Improved Red Fox Optimization Algorithm
International Journal of Hydrogen Energy(2021)
关键词
Extreme learning machines,Model identification,Output voltage,Solid oxide fuel cell,Improved red fox optimization algorithm
AI 理解论文
溯源树
样例
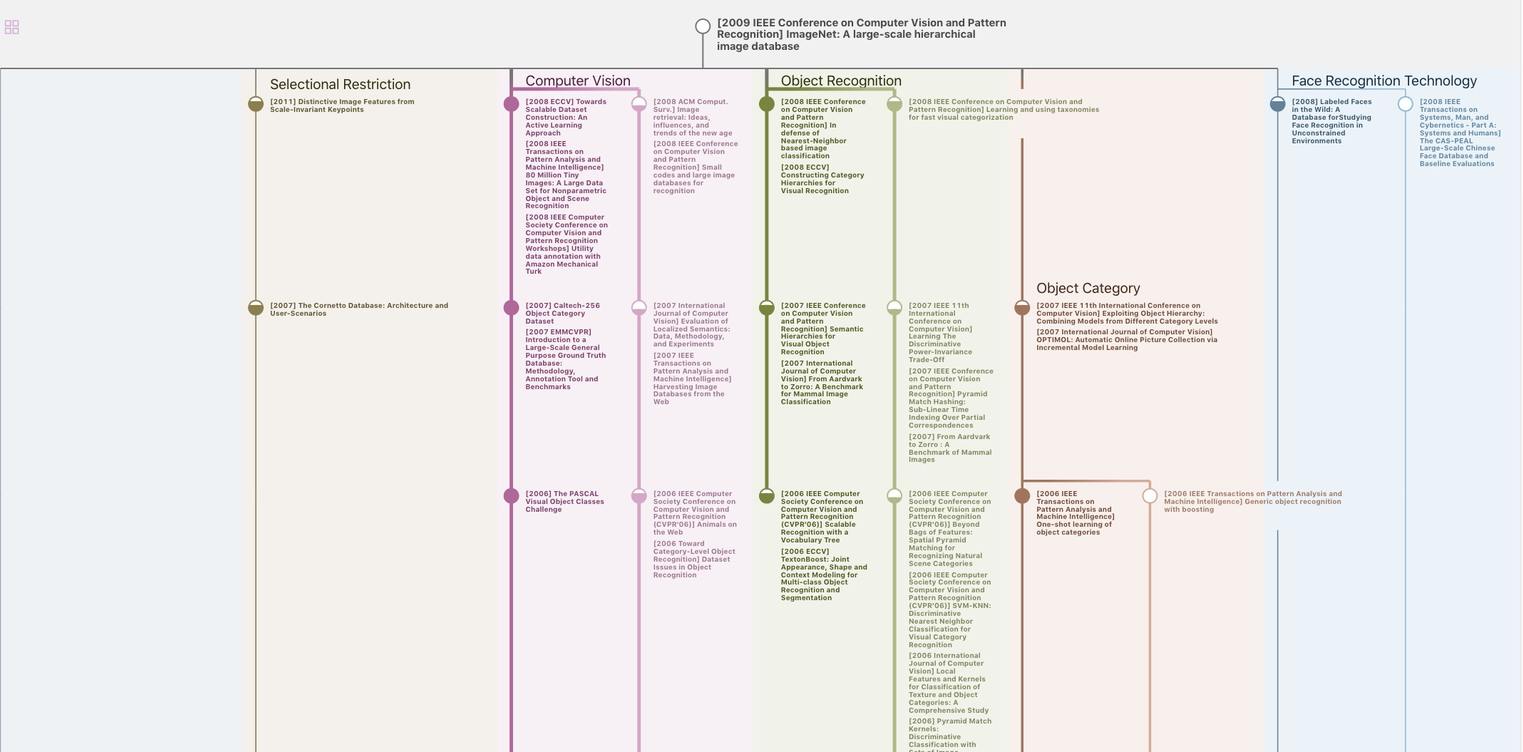
生成溯源树,研究论文发展脉络
Chat Paper
正在生成论文摘要