Multichannel convolutional neural network-based fuzzy active contour model for medical image segmentation
Evolving Systems(2021)
摘要
Anatomical maps depicted by medical images reflect the health of the human body. Understanding the detailed division of the regions in the anatomical maps is helpful for diagnosis and further treatment. For example, the lung window contains the lung parenchyma, mediastinum, and diseased tumor. Accurate tumor segmentation can be more comprehensive and accurate to determine the disease condition to select the appropriate radiotherapy means to improve the success rate of treatment. However, the traditional manual segmentation task is large, and the segmentation results vary with the experience level of the observer. Therefore, the development of automatic and reliable segmentation methods is a feasible and important task in clinical practice. Therefore, this paper proposes a multichannel convolutional neural network (CNN)-based fuzzy active contour model for medical image segmentation. Medical images are gridded and labeled using the superpixel SLIC method. The gridded images are used as data sets to train the multichannel CNN network to segment superpixels of organ boundaries, and the seed points of these superpixels are connected to form rough segmentation boundaries. The rough segmentation boundary is used as the initial contour, and the organ boundary in the medical image is obtained by fuzzy active contour segmentation. Finally, we conduct comparison experiments with the state-of-the-art segmentation methods, the results of the subjective evaluation and objective evaluation show that the proposed method has a better segmentation effect and robustness.
更多查看译文
关键词
Medical image segmentation, Multichannel CNN, SLIC, Fuzzy active contour
AI 理解论文
溯源树
样例
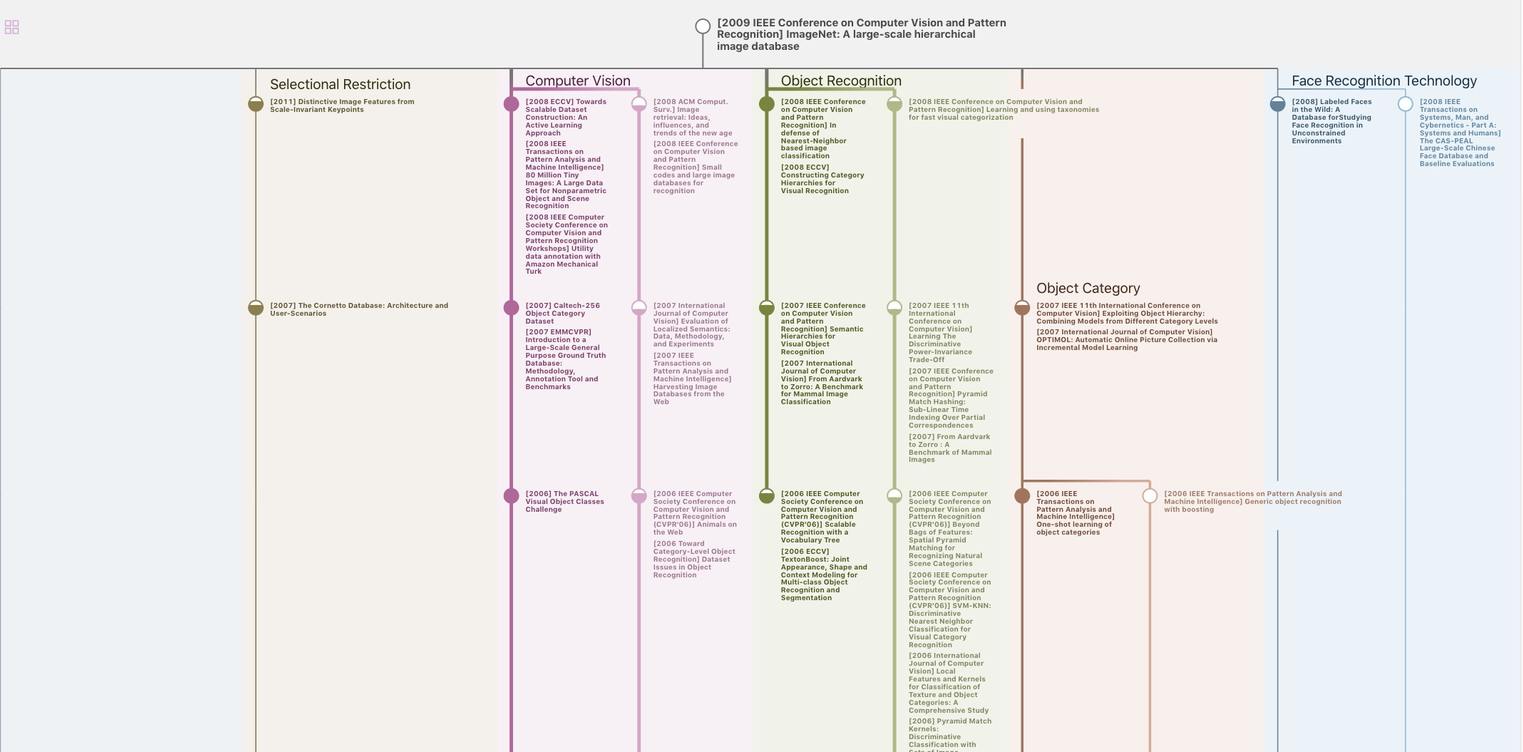
生成溯源树,研究论文发展脉络
Chat Paper
正在生成论文摘要