Supervised Sequence Labelling with Recurrent Neural Networks
Studies in Computational Intelligence(2012)
- Pretraining has recently greatly promoted the development of natural language processing (NLP)
- We show that M6 outperforms the baselines in multimodal downstream tasks, and the large M6 with 10 parameters can reach a better performance
- We propose a method called M6 that is able to process information of multiple modalities and perform both single-modal and cross-modal understanding and generation
- The model is scaled to large model with 10 billion parameters with sophisticated deployment, and the 10 -parameter M6-large is the largest pretrained model in Chinese
- Experimental results show that our proposed M6 outperforms the baseline in a number of downstream tasks concerning both single modality and multiple modalities We will continue the pretraining of extremely large models by increasing data to explore the limit of its performance
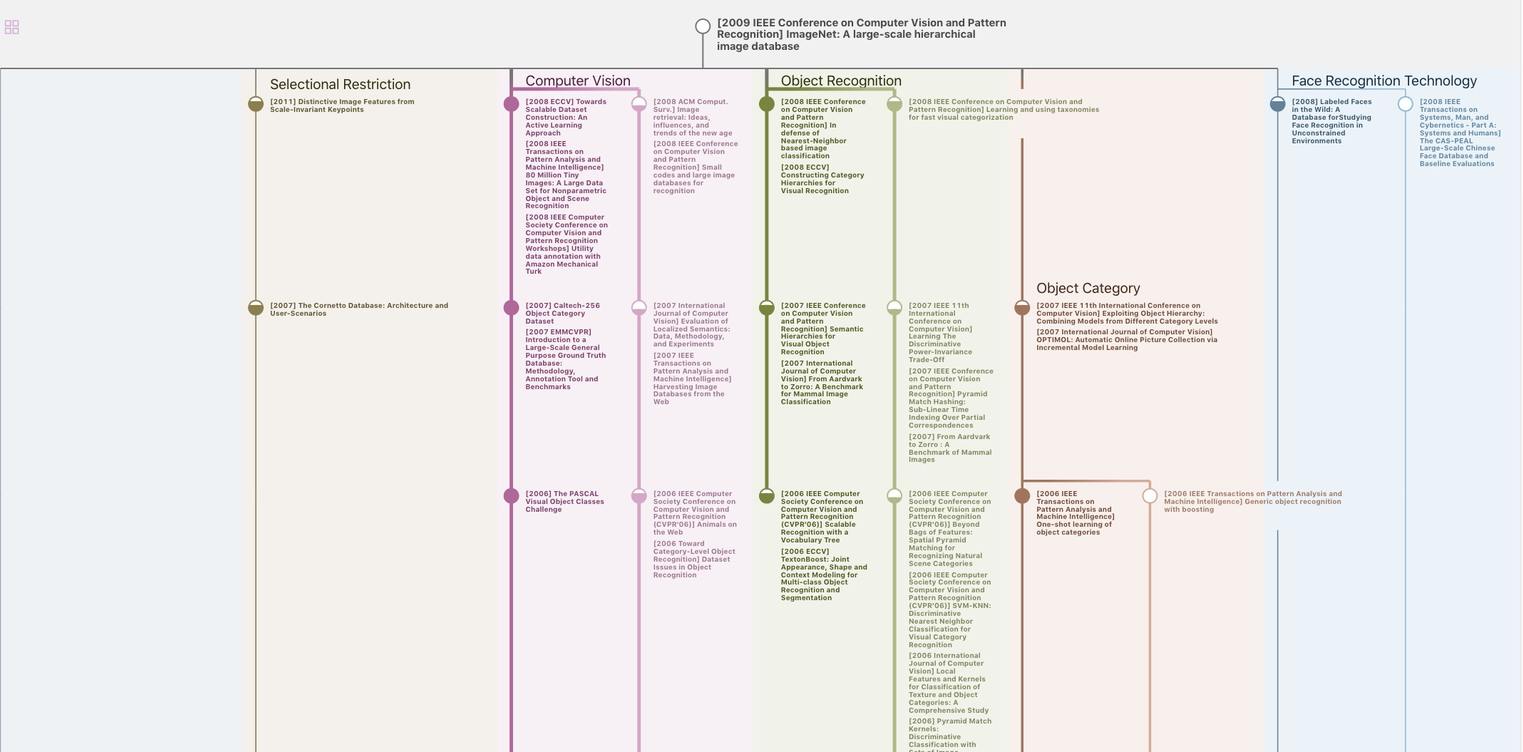
Local Feature Based Online Mode Detection with Recurrent Neural Networks
被引用32
Stabilize Sequence Learning with Recurrent Neural Networks by Forced Alignment
被引用10
Temporal and Situational Context Modeling for Improved Dominance Recognition in Meetings
被引用24
Multi View Facial Action Unit Detection Based on CNN and BLSTM-RNN.
被引用52
Multi-Granularity Neural Sentence Model for Measuring Short Text Similarity
被引用18
被引用3
Using Artificial Intelligence to Create Value in Insurance
被引用126
被引用69
Research on Construction Method of Dynamic Sentiment Dictionary Based on Bidirectional LSTM
被引用10
Factor Decomposition and Prediction of Solar Energy Consumption in the United States
被引用29
Aspect-Based Opinion Mining on Student’s Feedback for Faculty Teaching Performance Evaluation
被引用81
被引用89
被引用23
Attention-based Sentiment Reasoner for Aspect-Based Sentiment Analysis.
被引用54
Structured Sparsification of Gated Recurrent Neural Networks.
被引用9
Spatiotemporal Feature Descriptor for Micro-Expression Recognition Using Local Cube Binary Pattern
被引用13
Optimizing Geo-Distributed Data Analytics with Coordinated Task Scheduling and Routing
被引用28
BlockQNN: Efficient Block-wise Neural Network Architecture Generation
被引用132
Efficiently Solving the Practical Vehicle Routing Problem: A Novel Joint Learning Approach
被引用100
Stock Market Uncertainty Determination with News Headlines: A Digital Twin Approach
被引用0
被引用3
被引用1
被引用2
被引用1
A Fault Diagnosis Method for Flexible Converter Valve Equipment Based on DSC-BIGRU-MA
被引用0