A Model of Artificial Biological Time Series Generation
Journal of the Korean Physical Society(2021)
摘要
This paper presents a novel model of artificial biological time series generation. We employed a cellular automata (CA) system that comprises self-replicating and mutually competing loops. Our self-replicating system is an advanced version of Chou & Reggia’s system (Physica D 110, 252–276). The complex self-replicating CA loops were developed using a construction scheme in which we introduce a unique measure of the CA to quantify the activity of the loops. By summing the loop activities globally and observing the time evolution of the sum, we obtain an artificial time series. The power spectrum of the resulting time series exhibits $${1 \mathord{\left/ {\vphantom {1 {f^{\alpha } }}} \right. \kern-\nulldelimiterspace} {f^{\alpha } }}$$ scaling behavior. The exponent $$\alpha$$ in the $${1 \mathord{\left/ {\vphantom {1 {f^{\alpha } }}} \right. \kern-\nulldelimiterspace} {f^{\alpha } }}$$ power spectrum of the unconstrained self-replicating system is $$\alpha \approx 1.5$$ . Constraints on loop dynamics, leading to larger-scale death in the loop colonies, are implemented in our CA. With the application of the constraints, the value of $$\alpha$$ is lowered to nearly 1. The results show that self-replication and death in the CA space play the roles of excitatory and inhibitory synaptic functions, respectively, in a neuronal network. The self-replication/death balance control in our study could modulate the scaling exponent $$\alpha$$ in the power spectrum, similar to the activation/inhibition balance control in neural networks and local field potential (LFP) simulations in recent studies. The calculation of the largest Lyapunov exponent, L1, shows the chaotic nature of the time series. The form of our CA system resembles that of neuronal potential transmission and replication to nearby neurons. The results of this study suggest another feasible concept for the origin of biological signals. Executable codes associated with this study are freely accessible.
更多查看译文
关键词
Biological signal,Time series,Self-replication,1/f noise,Cellular automata
AI 理解论文
溯源树
样例
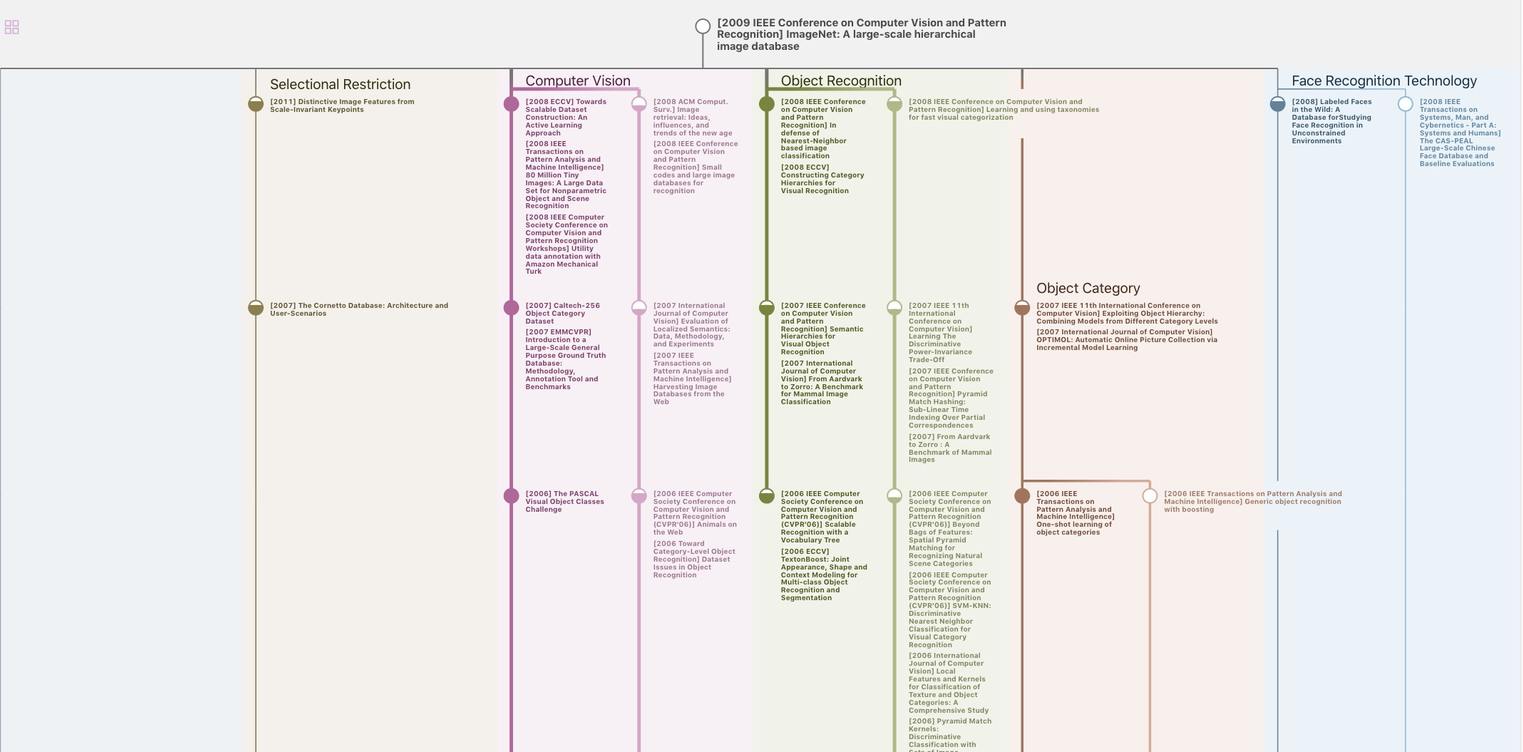
生成溯源树,研究论文发展脉络
Chat Paper
正在生成论文摘要