Synthesize of 3D-conductive supramolecular gel and derived N-doped Fe-C as high-performance lithium-ion battery anodes
VACUUM(2021)
摘要
In this work, 3D-conductive supramolecular gel and derivates were synthesized and the performance of lithium storage were explored. In sol or gel state of iron-based metal-organic gel (Fe-MOG), the redox reaction of Fe3+ will generate free radicals which can catalyze the polymerization of pyrrole. Meanwhile, the conductive polymer could be more uniformly and closely coated on the internal structure of Fe-MOG. Systematic electrochemical analysis demonstrated that 3D-conductive supramolecular gel anode performed a stable reversible specific capacity of 1304.9 mAh g(-1) at the current density of 0.1 A g(-1), and outstanding cycle performance with high reversible specific capacity of 824.5 mAh g(-1) at the current density of 0.4 A g(-1) after 300 cycles and 422.3 mAh g(-1) at the current density of 2 A g(-1) after 100 cycles. At the same time, supramolecular gel-derived N-doped Fe-C could also show excellent performance of lithium storage as a novel carbon material, demonstrating that 3D-conductive supramolecular gel has a broad application prospect in the field of lithium-ion batteries (LIBs).
更多查看译文
关键词
In situ polymerization,Metal-organic gel,Lithium ion batteries,Electrochemical performance
AI 理解论文
溯源树
样例
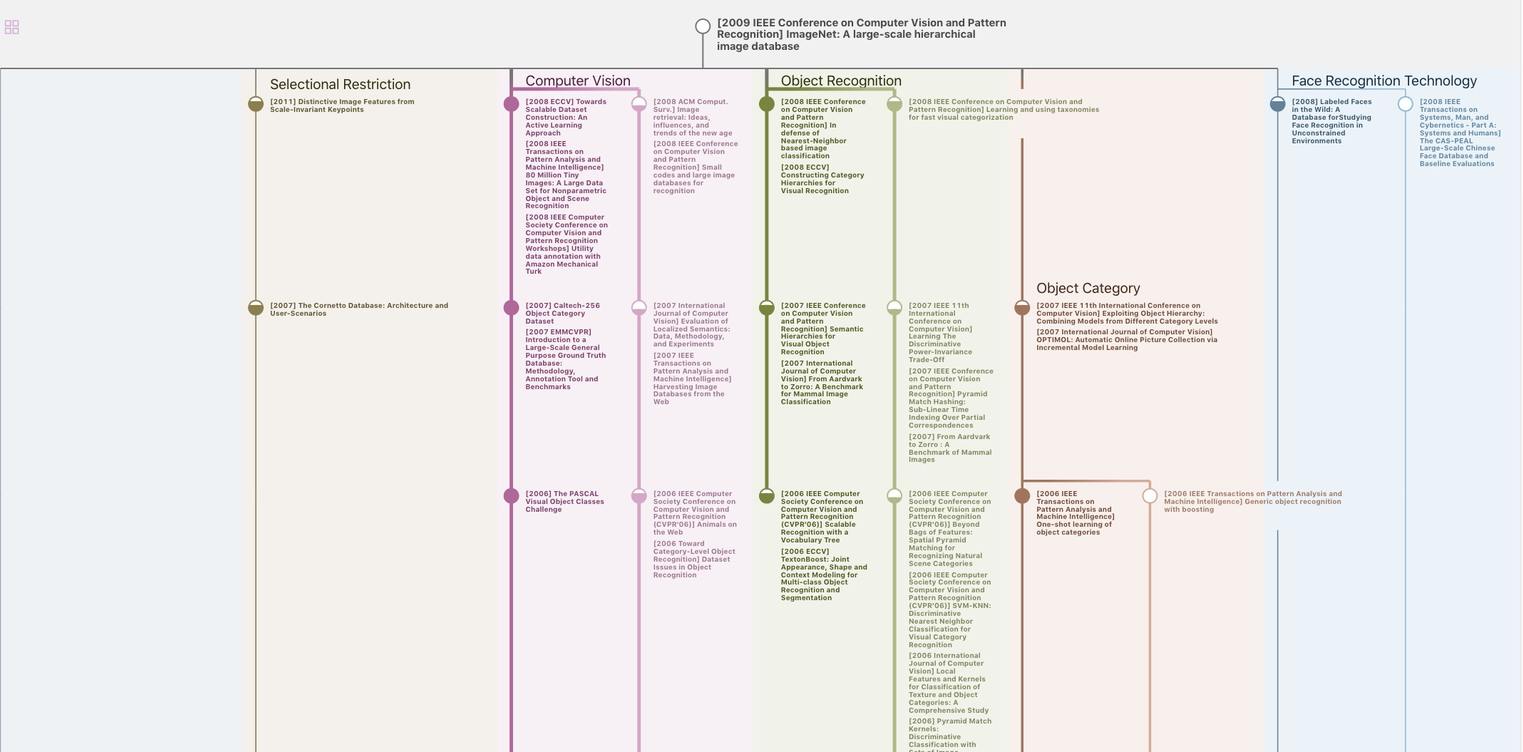
生成溯源树,研究论文发展脉络
Chat Paper
正在生成论文摘要