Kernel Operations on the GPU, with Autodiff, Without Memory Overflows
Journal of Machine Learning Research(2021)
摘要
The KeOps library provides a fast and memory-efficient GPU support for tensors whose entries are given by a mathematical formula, such as kernel and distance matrices. KeOps alleviates the major bottleneck of tensor-centric libraries for kernel and geometric applications: memory consumption. It also supports automatic differentiation and outperforms standard GPU baselines, including PyTorch CUDA tensors or the Halide and TVM libraries. KeOps combines optimized C++/CUDA schemes with binders for high-level languages: Python (Numpy and PyTorch), Matlab and GNU R. As a result, high-level "quadratic" codes can now scale up to large data sets with millions of samples processed in seconds. KeOps brings graphics-like performances for kernel methods and is freely available on standard repositories (PyPi, CRAN). To showcase its versatility, we provide tutorials in a wide range of settings online at \url{this http URL}.
更多查看译文
关键词
kernel methods,Gaussian processes,GPU,automatic differentiation
AI 理解论文
溯源树
样例
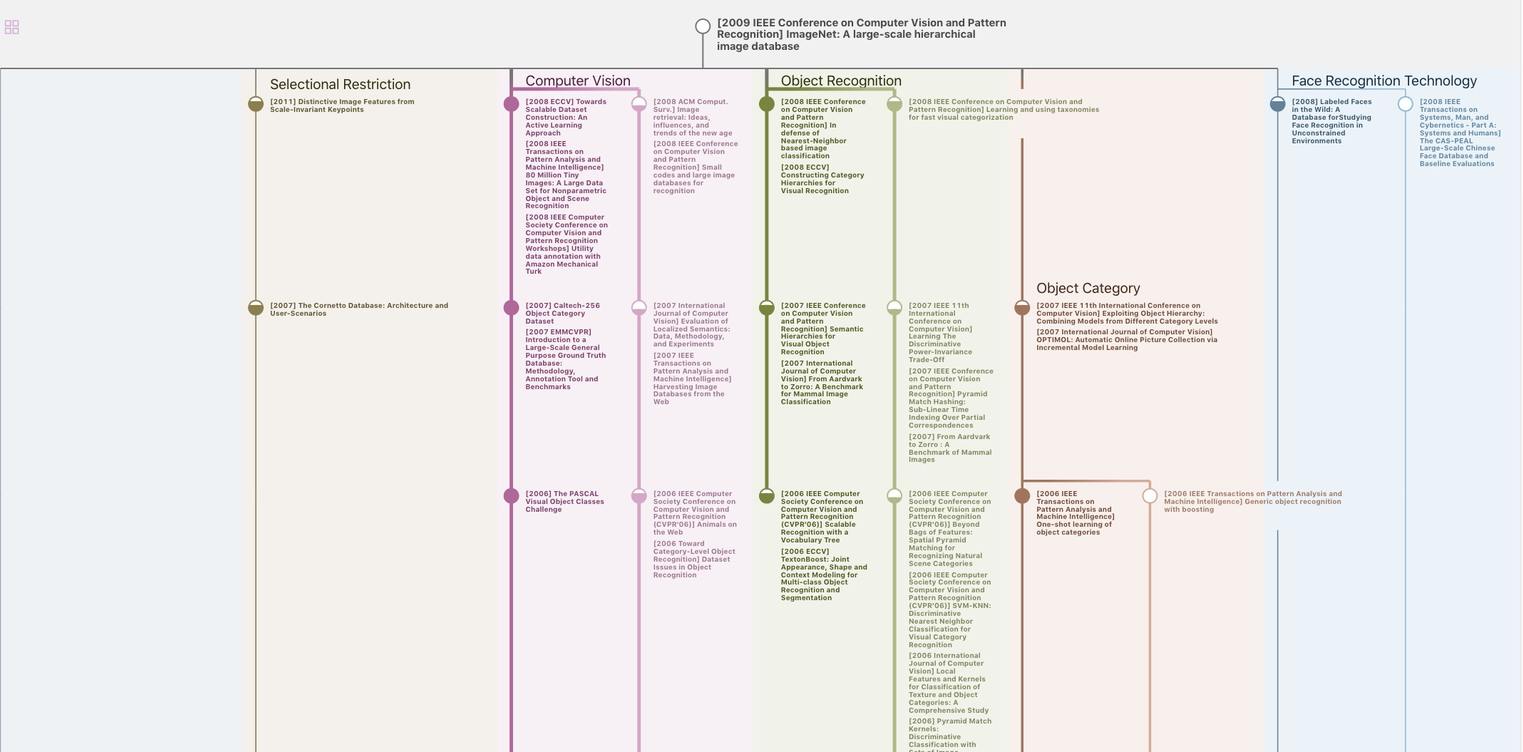
生成溯源树,研究论文发展脉络
Chat Paper
正在生成论文摘要