Quantile Regression-Based Multiple Imputation of Missing Values — an Evaluation and Application to Corporal Punishment Data
Methodology(2021)
摘要
Quantile regression (QR) is a valuable tool for data analysis and multiple imputation (MI) of missing values – especially when standard parametric modelling assumptions are violated. Yet, Monte Carlo simulations that systematically evaluate QR-based MI in a variety of different practically relevant settings are still scarce. In this paper, we evaluate the method regarding the imputation of ordinal data and compare the results with other standard and robust imputation methods. We then apply QR-based MI to an empirical dataset, where we seek to identify risk factors for corporal punishment of children by their fathers. We compare the modelling results with previously published findings based on complete cases. Our Monte Carlo results highlight the advantages of QR-based MI over fully parametric imputation models: QR-based MI yields unbiased statistical inferences across large parts of the conditional distribution, when parametric modelling assumptions, such as normal and homoscedastic error terms, are violated. Regarding risk factors for corporal punishment, our MI results support previously published findings based on complete cases. Our empirical results indicate that the identified “missing at random” processes in the investigated dataset are negligible.
更多查看译文
关键词
missing values,multiple imputation,quantile regression,random forest,corporal punishment,parenting behavior
AI 理解论文
溯源树
样例
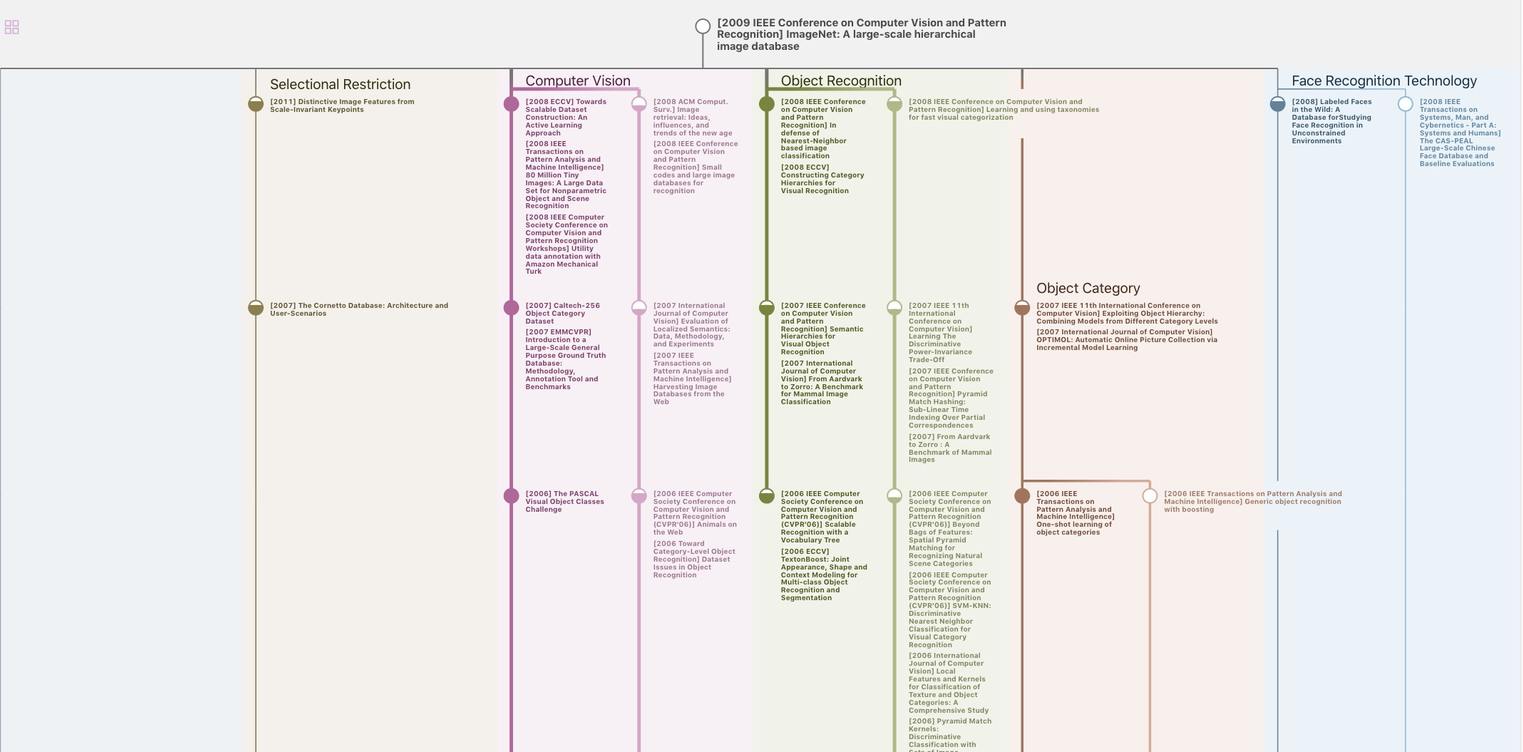
生成溯源树,研究论文发展脉络
Chat Paper
正在生成论文摘要