Probabilistic Spatiotemporal Solar Irradiation Forecasting Using Deep Ensembles Convolutional Shared Weight Long Short-Term Memory Network
APPLIED ENERGY(2021)
摘要
High-quality and reliable solar radiation spatiotemporal probabilistic prediction is very significant for the planning and management of solar energy. In this paper, convolutional shared weight long short-term memory network is proposed to deal with solar radiation spatiotemporal prediction problems. The model can minimize the training time of model without significantly reducing prediction accuracy. Furthermore, a spatiotemporal probabilistic prediction model that combines convolutional shared weight long short-term memory network and deep ensemble method is proposed to deal with the solar radiation probabilistic prediction problem. The model obtains the uncertainty estimation of predictands by adjusting the network structure and optimizes the uncertainty by employing a proper scoring rule. In addition, a combination of ensembles are used in the model to improve the robustness of probabilistic prediction. The new spatiotemporal probabilistic prediction model is applied to predict solar radiation in a real area of the United States. Five state-of-the-art models and seven evaluation indicators are used for comparation. The comparation results show the proposed model is able to provide accurate point prediction, reasonable prediction interval and reliable probabilistic prediction results for a whole area.
更多查看译文
关键词
Deep ensemble, Deep learning, Probabilistic prediction, Spatiotemporal feature, Solar energy
AI 理解论文
溯源树
样例
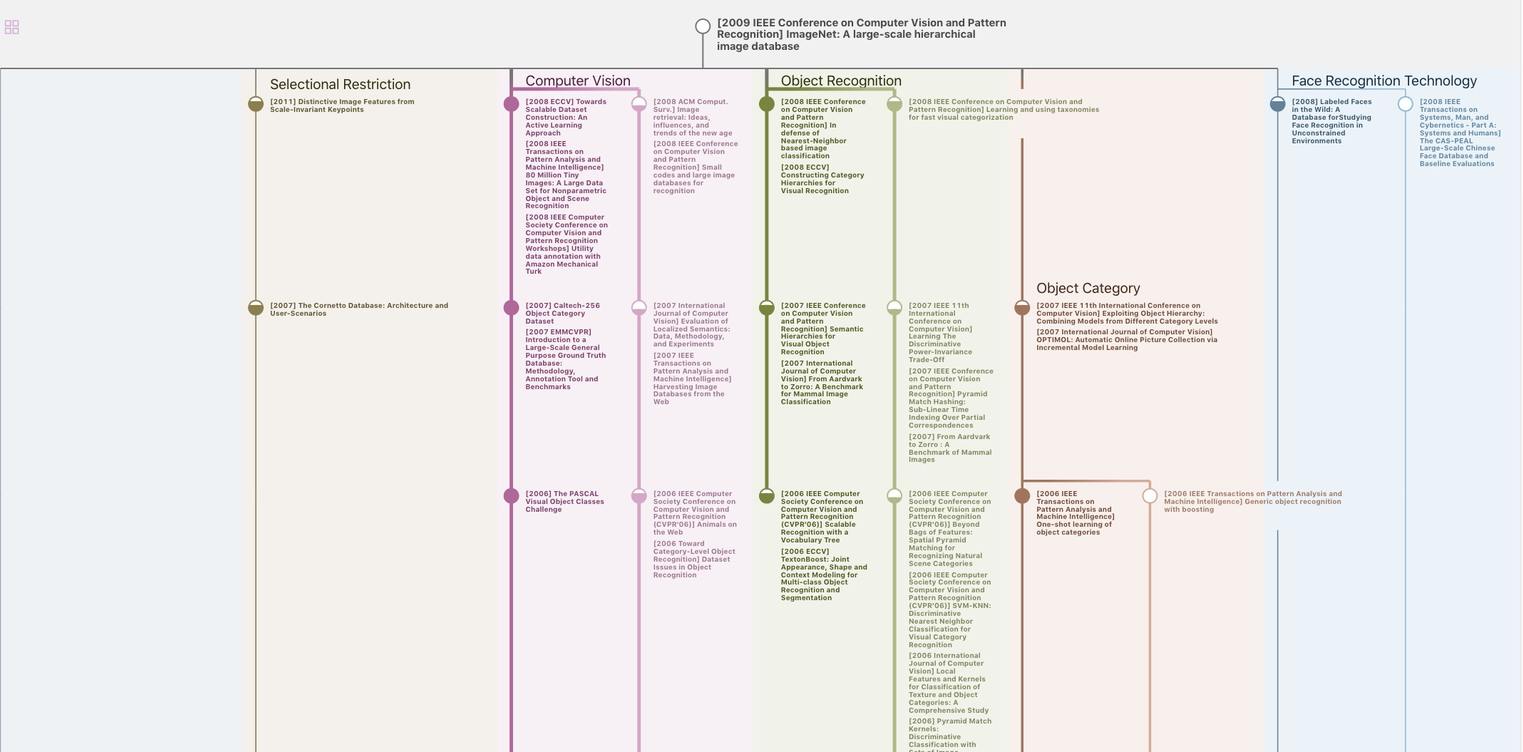
生成溯源树,研究论文发展脉络
Chat Paper
正在生成论文摘要