Prediction And Evaluation Method Of Tvb-N Values Distribution In Pork By Hyperspectral Imaging
INTERNATIONAL JOURNAL OF AGRICULTURAL AND BIOLOGICAL ENGINEERING(2021)
摘要
Hyperspectral imaging makes it possible to map the spatial distribution of quality-indicating attributes over biological targets. However, inconsistencies may arise between these newly available qualitymaps of the same piece of the biological target from different chemometric models. Therefore, such inconsistency of the spatial prediction of the freshness-indicating attribute of total volatile basic nitrogen (TVB-N) over pork loins (Longissimus dorsi) was investigated in this work from the perspective of the accuracy and variation of pixel-wise prediction over the top-surfaces. The partial least square regression (PLSR)-based chemometric modelling was performed on the characteristic spectra of a target extracted from regions-of-interest (ROIs) of both the whole meat and the lean part only, after spectral preprocessing and spatial filtering, and coupled with waveband selection using successive projections algorithm (SPA). Results showed that all PLSR models achieved good and stable predictions of the average TVB-N values of pork loins, despite the differences of data-preprocessing or numbers of wavebands, with R-p(2) being in the range from 0.832 to 0.889 for Meat-ROI and an even higher range from 0.846 to 0.912 if using Lean-ROIs, even with a reduced number of wavebands, accuracy remaining high in the range of R-p(2) from 0.722 to 0.889 for Meat-ROIs and from 0.704 to 0.900 for Lean-ROIs. In contrast, however, devastating differences emerged between the predicted TVB-N distributions over a top-surface. Results showed that excessive variation of pixel-wise predictions rendered utterly useless distribution maps resulted from a direct application of the PLSR or PLSR_SPA models from Meat or Lean ROIs, quantified by root-mean-error (RMSE) of pixel-wise predictions over 21.6 mg/100 g without PLSR, or even the worse with PLSR-SPA, shooting absurdly high about 55 mg/100 g. After appropriate spatial filtering, the excessive between-pixel variation was suppressed to below 8.5 mg/100 g in RMSE, presenting visually better distribution maps, but at a significant loss in accuracy, R-p(2) dropping from 0.79 to a barely enough 0.58 for Meat-ROI, and from 0.86 to a failing value of 0.42 for Lean-ROI. In conclusion, though good and reliable for the average TVB-N prediction of larger surfaces such as that of pork loins, hyperspectral imaging-based prediction of TVB-N distribution is more challenging. A compromise must be made between the accuracy and visual goodness of such quality maps and a meaningful variation of the pixel-wise values, possibly with appropriate spatial filtering. And never trust a quality-indicating map, even if a visually pleasing one, without going through the evaluation of its accuracy and pixel-wise variation.
更多查看译文
关键词
pork, TVB-N, hyperspectral imaging, prediction, evaluation, distribution, PLSR, SPA
AI 理解论文
溯源树
样例
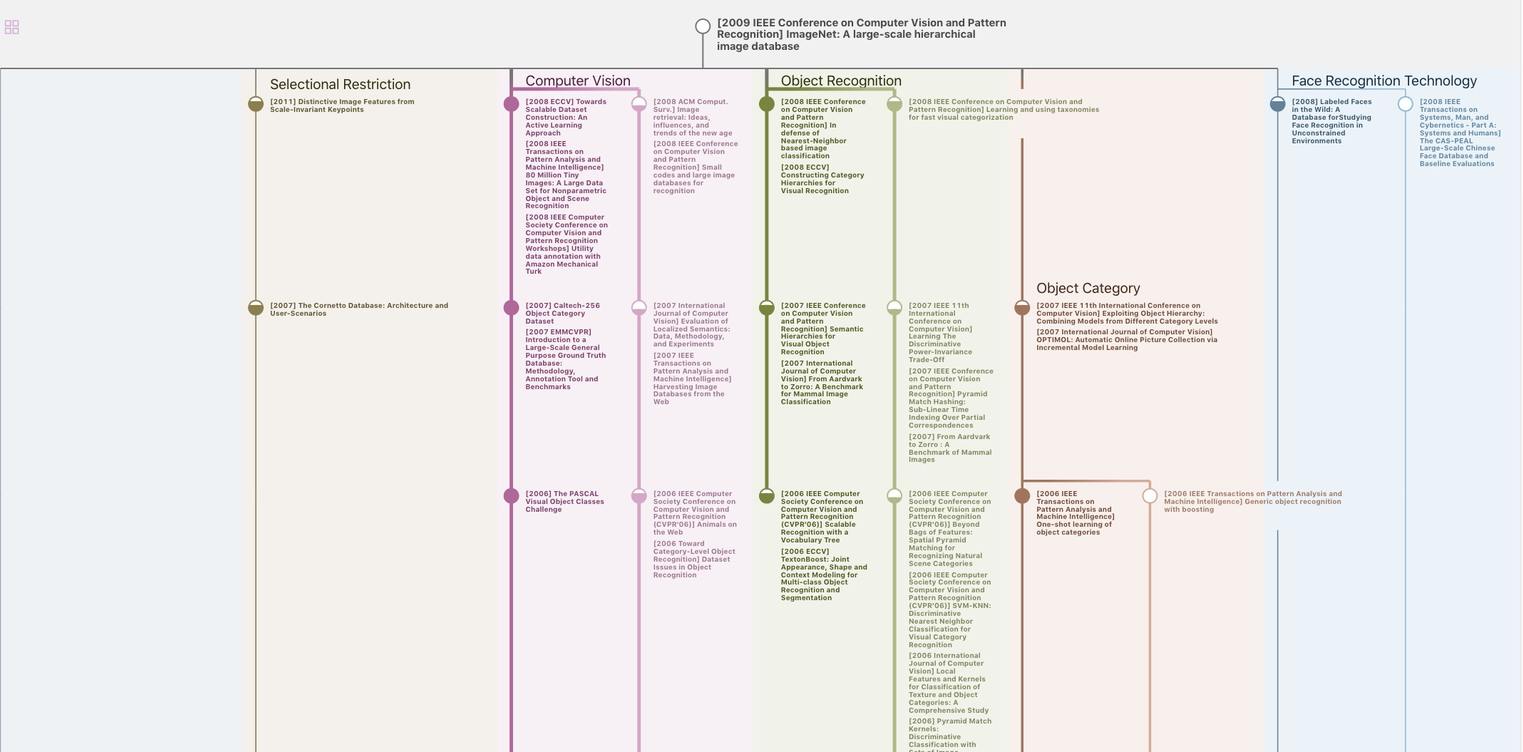
生成溯源树,研究论文发展脉络
Chat Paper
正在生成论文摘要