Fluorescence Spectral Shape Analysis For Covid-19 Virus Identification: Machine Learning Approach
REMOTE SENSING FOR AGRICULTURE, ECOSYSTEMS, AND HYDROLOGY XXII(2020)
摘要
Fast and reliable tests for the new coronavirus are urgently needed. Current Polymerase Chain Reaction based virus detection approaches are typically time-consuming and expensive. Technologies capable of providing a fast, real-time and non-contact detection of virus contamination and real-time virus classification are not yet available. Here, we demonstrate the potential of a fluorescence detection technique along with machine-learning based classification for virus detection. The ultraviolet (UV) light irradiated virus emits a fluorescent signal with a characteristic spectrum, which is regarded as a fingerprint for the virus. We analyzed eight virus samples including a heat-inactivated SARS-CoV-2 (virus causing COVID-19) and collected a number of emission spectra. Machine learning techniques are applied to discriminate among the candidate viruses via classifying a number of spectra data collected. First, Principle Component Analysis (PCA) was applied to reduce spectra data dimensionality. Then support vector machine (SVM) with various kernel functions (kernel-SVM), k-nearest-neighbor (k-NN) and Artificial Neural Networks (ANN) methods were used to classify these viruses with dimension-reduced data from PCA. We found that dimension-reduced data in 3 principal components (PCs) space performs better than that in 2 PCs space in the machine learning algorithms mentioned above. Variance ratio analysis is able to explain nearly 95% of variance which allows nearly 100% accuracy of predictions for 25% data test set randomly chosen from the whole dataset. Finally, cross validation (CV) analysis is applied to kernel-SVM and k-NN methods.
更多查看译文
关键词
machine learning,fluorescence,biosensor,SARS-CoV-2,virus,virological analysis
AI 理解论文
溯源树
样例
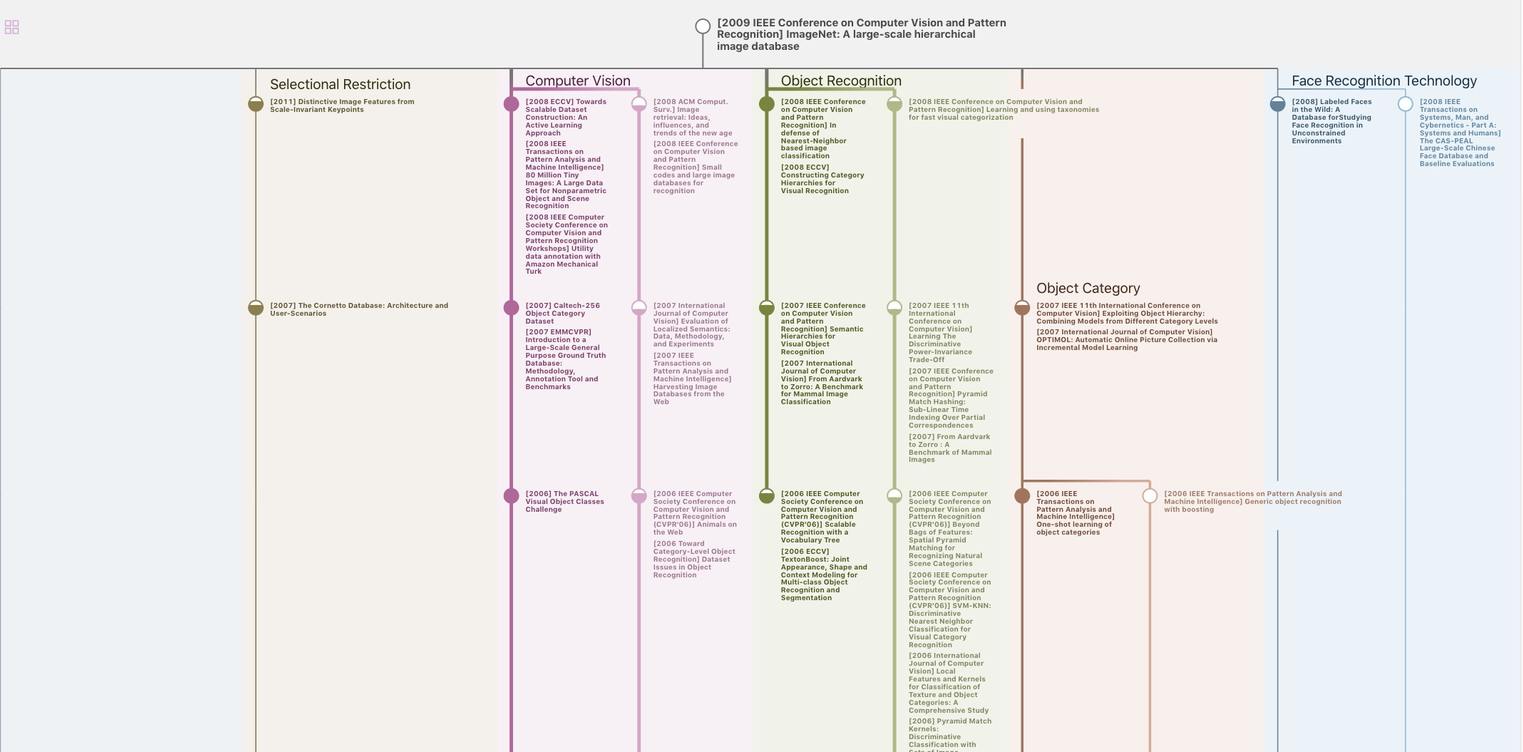
生成溯源树,研究论文发展脉络
Chat Paper
正在生成论文摘要