Putting The Pieces Together
EXPLORING THE STRATEGY SPACE OF NEGOTIATING AGENTS: A FRAMEWORK FOR BIDDING, LEARNING AND ACCEPTING IN AUTOMATED NEGOTIATION(2016)
摘要
In the previous chapters, we performed extensive research into three different aspects of an automated negotiator: bidding (Chaps. 8 and 9), learning (Chaps. 6 and 7), and accepting (Chaps. 4 and 5). We have found novel ways to handle each component, sometimes even in optimal ways for particular circumstances. The natural question then arises: how do the pieces fit together? Or, more specifically: how do the components of a negotiating agent influence its overall performance, and which components are the most important for the end result of an agent? In this chapter, we provide an answer to this question in a quantitative way. In doing so, we show that the BOA framework not only provides a useful basis for developing and evaluating agent components, but also provides a powerful agent design tool. Furthermore, we demonstrate that combining effective key components from different agents improves an agent's overall performance. This validates the analytical approach of the BOA framework towards optimizing the individual components of a negotiating agent. By combining agent components in varying ways, we are able to demonstrate the contribution of each component to the overall negotiation result, and thus determine the key contributing components. Moreover, we study the interaction between components and present detailed interaction effects. We find that the bidding strategy in particular is of critical importance to the negotiator's success and far exceeds the importance of opponent preference modeling techniques. Our results contribute to the shaping of a research agenda for negotiating agent design by providing guidelines on how agent developers can spend their time most effectively.
更多查看译文
AI 理解论文
溯源树
样例
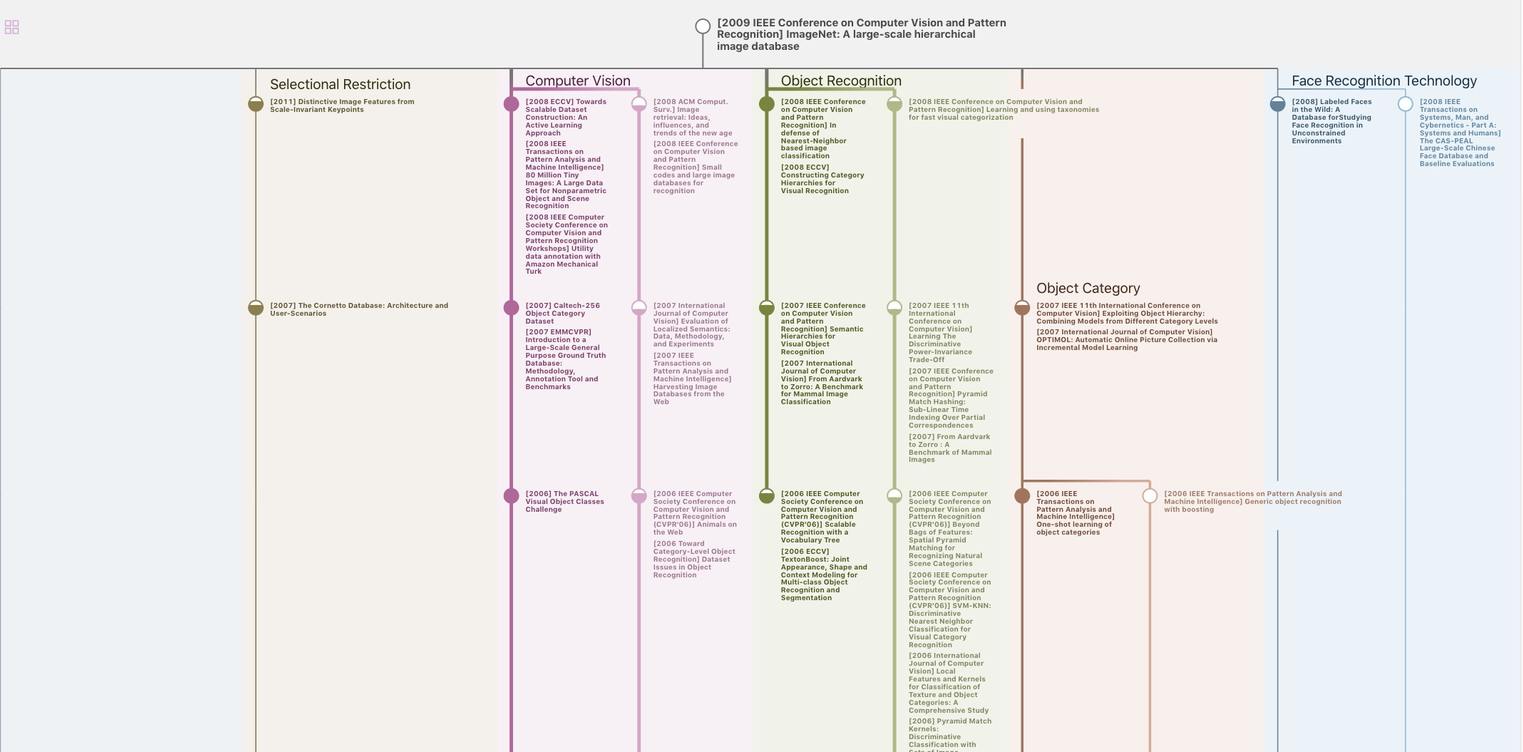
生成溯源树,研究论文发展脉络
Chat Paper
正在生成论文摘要