Model Selection for Data Analysis in Encrypted Domain: Application to Simple Linear Regression
INFORMATION SECURITY APPLICATIONS, WISA 2019(2020)
Abstract
In the big data era, data scientists explore machine learning methods for observed data to predict or classify. For machine learining to be effective, it requires access to raw data which is often privacy sensitive. In addition, whatever data and fitting procedures are employed, a crucial step is to select the most appropriate model from the given dataset. Model selection is a key ingredient in data analysis for reliable and reproducible statistical inference or prediction. To address this issue, we develop new techniques to provide solutions for running model selection over encrypted data. Our approach provides the best approximation of the relationship between the dependent and independent variable through cross validation. After performing 4-fold cross validation, 4 different estimates of our model's errors are calculated. And then we use bias and variance extracted from these errors to find the best model. We perform an experiment on a dataset extracted from Kaggle and show that our approach can homomorphically regress a given encrypted data without decrypting it.
MoreTranslated text
Key words
Fully Homomorphic Encryption,TFHE,Model selection
AI Read Science
Must-Reading Tree
Example
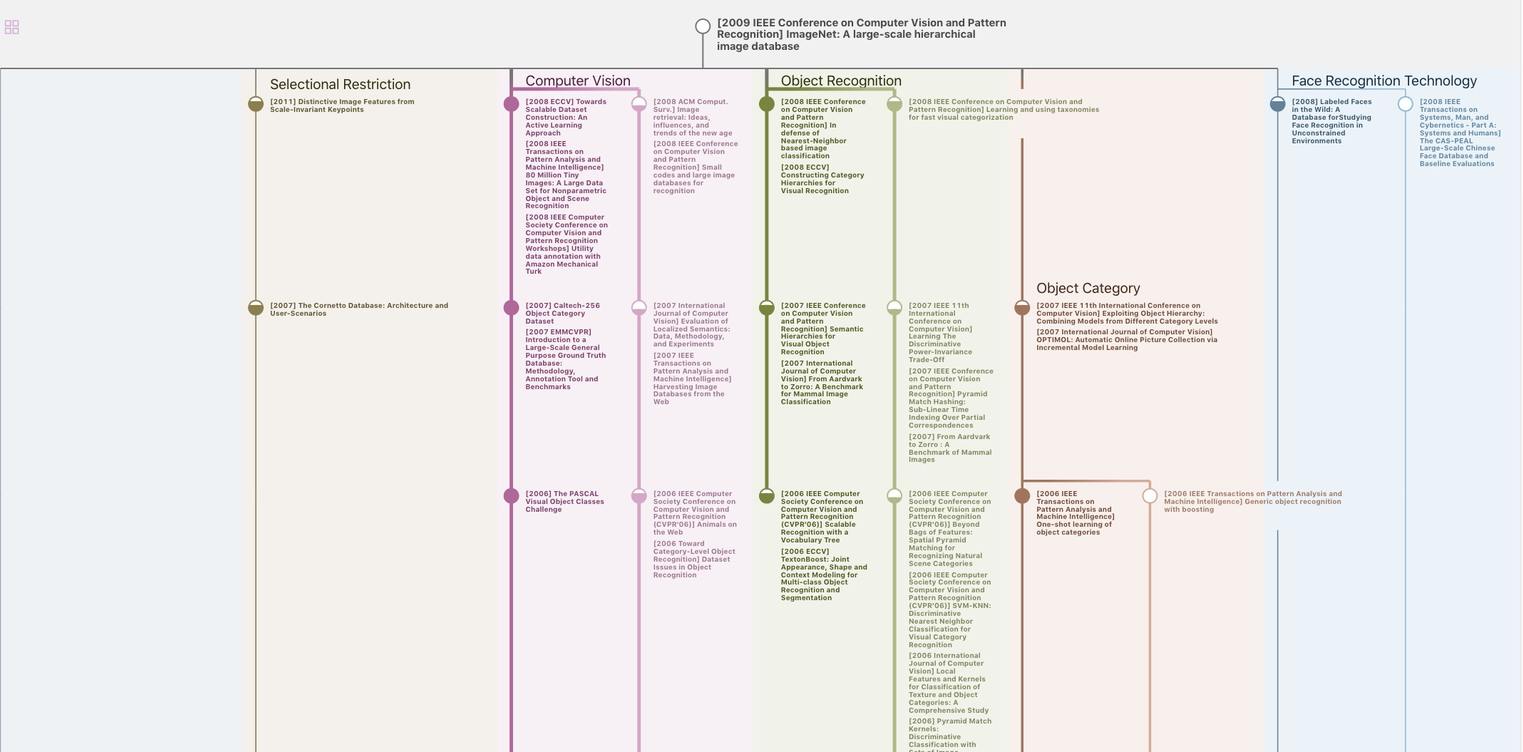
Generate MRT to find the research sequence of this paper
Chat Paper
Summary is being generated by the instructions you defined