A Multi-Pass Sieve For Clinical Concept Normalization
TRAITEMENT AUTOMATIQUE DES LANGUES(2020)
摘要
Clinical concept normalization involves linking entity mentions in clinical narratives to their corresponding concepts in standardized medical terminologies. It can be used to determine the specific meaning of a mention, facilitating effective use and exchange of clinical information, and to support semantic cross-compatibility of texts. We present a rule-based multipass sieve approach incorporating both exact and approximate matching based on dictionaries, and experiment with back-translation as a means of data augmentation. The dictionaries are built from the UMLS Metathesaurus as well as MCN corpus training data. Additionally, we train a multi-class baseline based on BERT. Our multi-pass sieve approach achieves an accuracy of 82.0% on the MCN corpus, the highest for any rule-based method. A hybrid method combining these two achieves a slightly higher accuracy of 82.3%.
更多查看译文
关键词
Clinical concept normalization, Rule-based sieve, Back-translation, Neural classifier
AI 理解论文
溯源树
样例
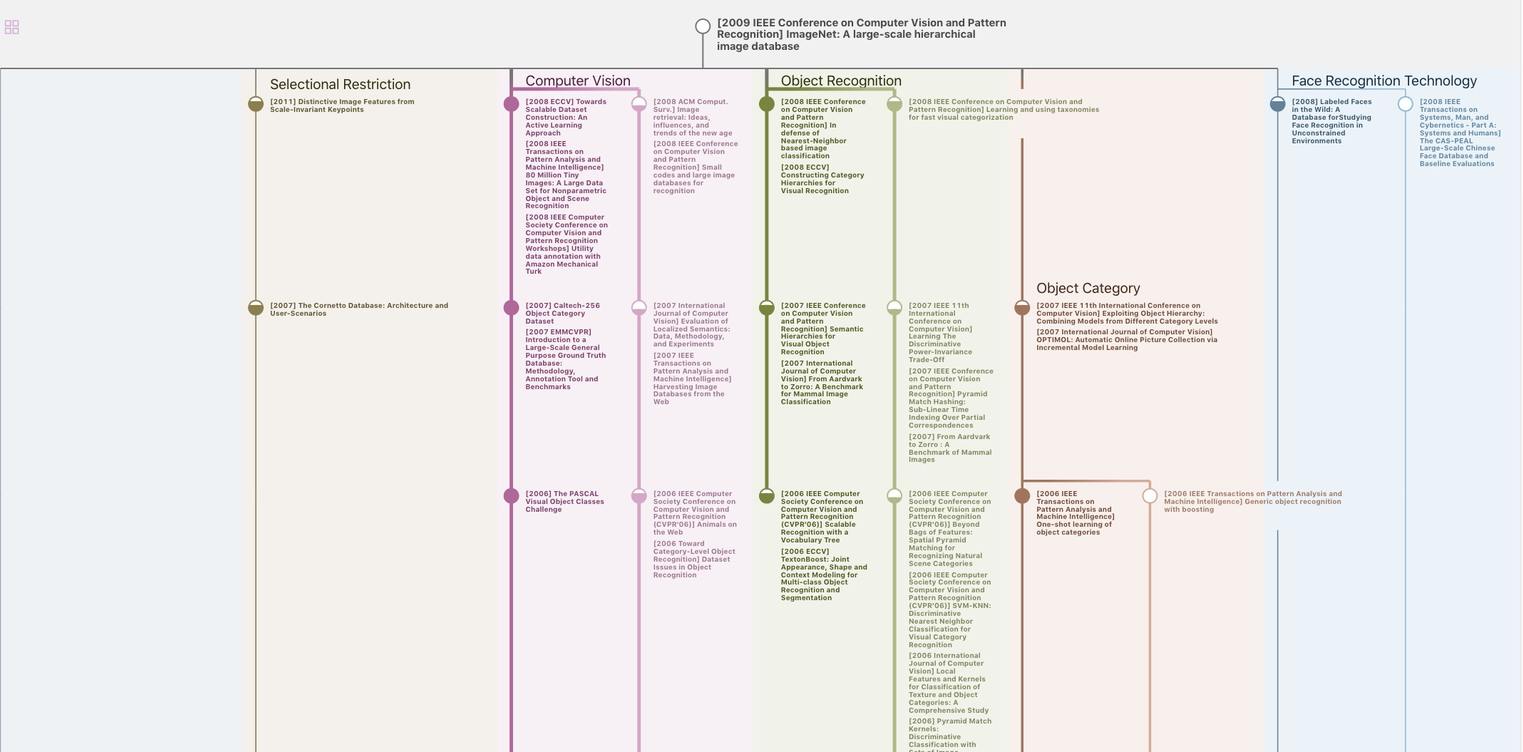
生成溯源树,研究论文发展脉络
Chat Paper
正在生成论文摘要