Can We Predict Psychiatric Disorders at the Adolescence Period in Toddlers? A Machine Learning Approach
Psychiatry and behavioral sciences (Online)(2020)
摘要
Objective: Recent studies show emotional and behavioral problems in toddler hood affecting later stages of development. However, the predictive factors for psychiatric disorders were not studied with machine learning methods. We aimed to examine the predictors of outcome with machine learning methods, which are novel computational methods including statistical estimation, information theories, and mathematical learning automatically discovering useful patterns in large amounts of data. Method: The study group comprised 116 children (mean age: 27.4 +/- 4.4 months) who are evaluated between 2006-2007 years in a clinical setting. Emotional and behavioral problems were assessed by the Brief Infant-Toddler Social Emotional Assessment and Child Behavior Checklist/2-3.Child psychiatry residents made follow-up evaluations with telephone calls in 2018. We tested the performance of machine learning algorithms (Decision tree, Support Vector Machine, Random Forest, Naive Bayes, Logistic Regression) on our data, including the 254 items in the baseline forms to predict psychiatric disorders in adolescence period. Results: 26.7% (n: 31) of the cases had diagnosed with a psychiatric disorder in adolescence period. In machine learning methods Random Forest outperforms other models, which had reached an accuracy of 85.2%, AUC: 0.79. Our model showed BITSEA item 20, 13, and CBCL total external problems scores filled by mother at the age of 12-36 months are the most potent factors for a psychiatric disorder in adolescence. Conclusion: We found very early behavioral and emotional problems with sociodemographic data predicted outcome significantly accurately. In the future, the machine learning models may reveal several others are more important in terms of prognostic information and also planning treatment of toddlers.
更多查看译文
关键词
Child Psychiatry,Artificial Intelligence,Prediction,Supervised Learning,Classification
AI 理解论文
溯源树
样例
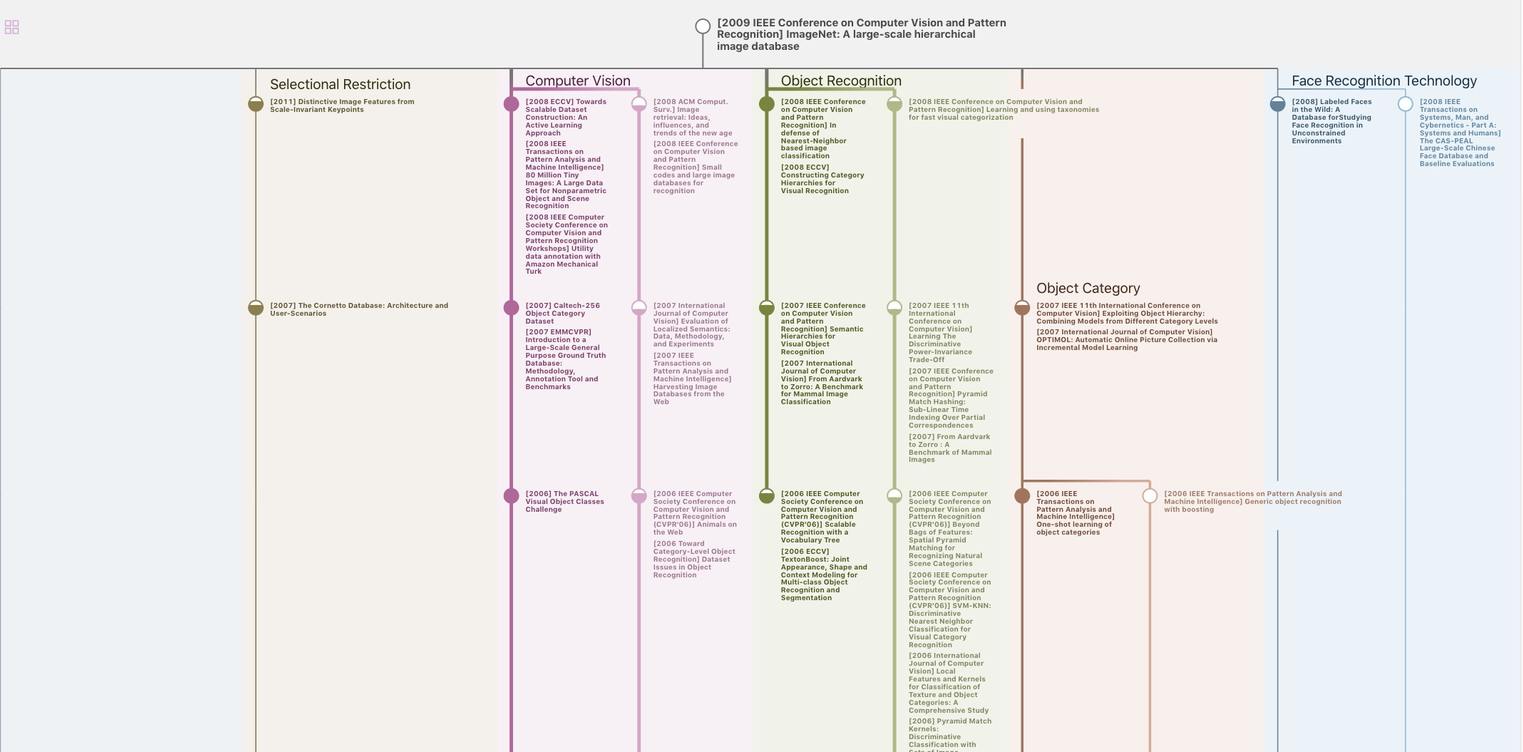
生成溯源树,研究论文发展脉络
Chat Paper
正在生成论文摘要