A Deep Learning Framework for Viable Tumor Burden Estimation
2020 6th Iranian Conference on Signal Processing and Intelligent Systems (ICSPIS)(2020)
摘要
Liver masses have become a common clinical challenge since they require to be defined and accurately categorized as neoplastic or nonneoplastic lesions. Hepatocellular carcinoma (HCC), the most common histologic type of primary liver malignancy, is a global health concern being the fifth most common cancer and the second cause of cancer mortality worldwide. Accurate diagnosis, which in some circumstances requires histopathology results, is necessary for appropriate management. Also, some tumor characteristics help in predicting tumor behavior and patient response to therapy. In this paper, we propose a deep learning framework for the segmentation of whole and viable tumor areas of liver cancer from whole-slide images (WSIs). To this end, we use Fast Segmentation Convolutional Neural Network (Fast-SCNN) as our network. We use the dataset from PAIP 2019 challenge. After data-augmentation on the training subset, we train the network with a multi-term loss function and SWA technique. Our model achieves 0.80 for the median of the Jaccard Index for the task of Viable Tumor Segmentation and 0.77 for the median of Weighted Absolute Accuracy for the task of Viable Tumor Burden Estimation on the whole-slide images of the test subset.
更多查看译文
关键词
Cancer Detection,Deep Convolutional Neural Networks,Pathology,Semantic Segmentation,Viable Tumor Burden Estimation
AI 理解论文
溯源树
样例
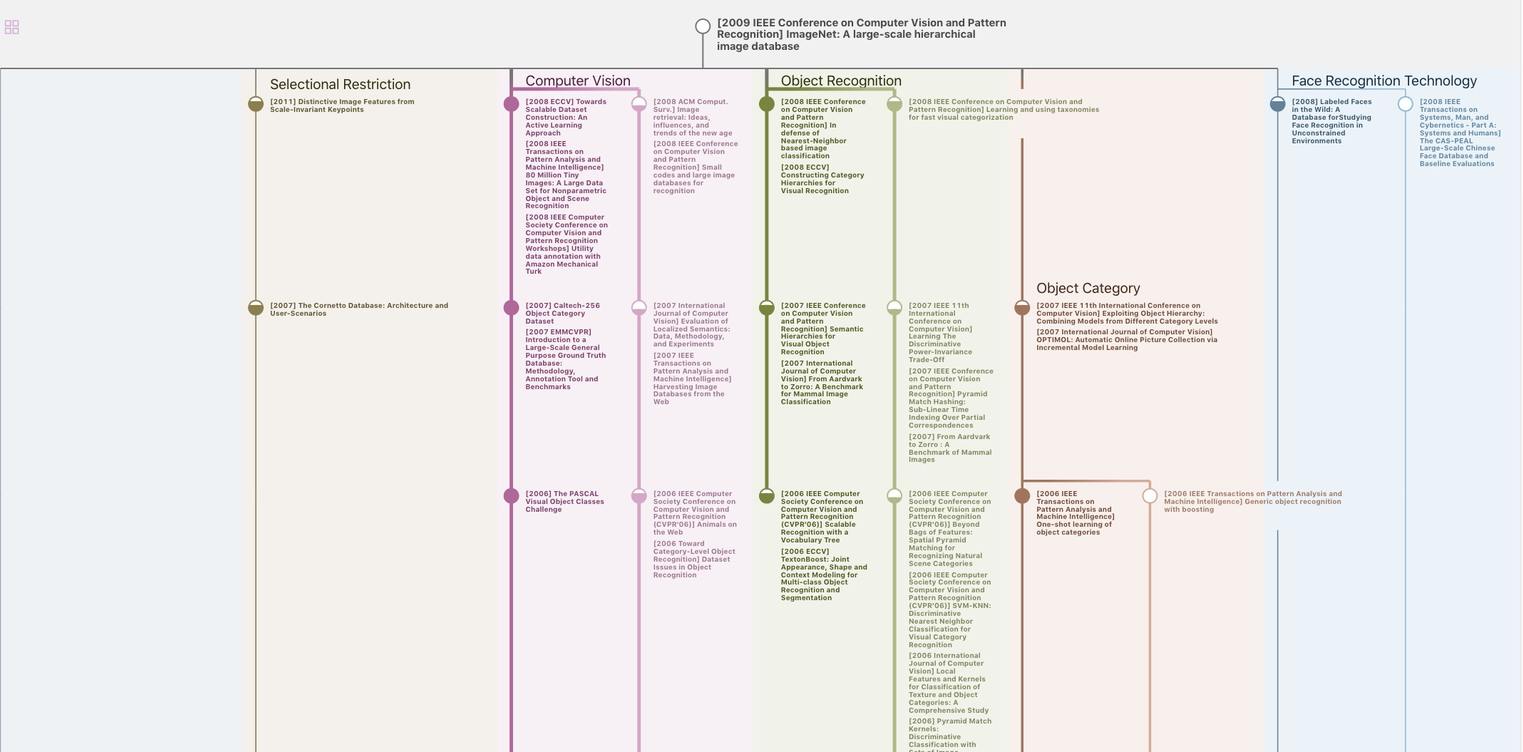
生成溯源树,研究论文发展脉络
Chat Paper
正在生成论文摘要