Firefly Optimization Based Improved Fuzzy Clustering For Ct/Mr Image Segmentation
NATURE INSPIRED OPTIMIZATION TECHNIQUES FOR IMAGE PROCESSING APPLICATIONS(2019)
摘要
The segmentation is the process of extraction of the desired region of interest. In medical images, the anatomical organs and anomalies like a tumor, cysts, etc. are of importance for the diagnosis of diseases by physicians for telemedicine applications. The thresholding, region growing, and edge detection are termed as classical segmentation algorithms. Clustering is an unsupervised learning technique to group similar data points and fuzzy partitioning merges similar pixels based on the fuzzy membership value. The classical FCM algorithm lacks sensitivity in the cluster centroid initialization and often gets trapped in local minima. The optimization algorithm gains its importance in cluster centroids initialization, thereby improving the efficiency of FCM algorithm. In this work, firefly optimization is coupled with FCM algorithm for CT/ MR medical image segmentation. Fireflies are insects having a natural capacity to illumine in dark with glowing and flickering lights and firefly optimization algorithm was modeled based on its biological traits. The preprocessing stage comprises of artifacts removal and denoising by Nonlinear Tensor Diffusion (NLTD) filter. The computation time was minimized by reducing the total pixels count for the processing. The Firefly optimization, when coupled with FCM, generates satisfactory results inconsistent with FCM when coupled with Cuckoo, Artificial Bee Colony, and Simulated annealing algorithms. The cluster validity performance metrics are used for the determination of optimum number of clusters. The algorithms are developed in Matlab 2010a and tested on real-time abdomen datasets.
更多查看译文
关键词
Unsupervised learning, Clustering, Fuzzy C means, FCM-firefly algorithm, FCM-artificial bee colony algorithm, FCM-cuckoo algorithm
AI 理解论文
溯源树
样例
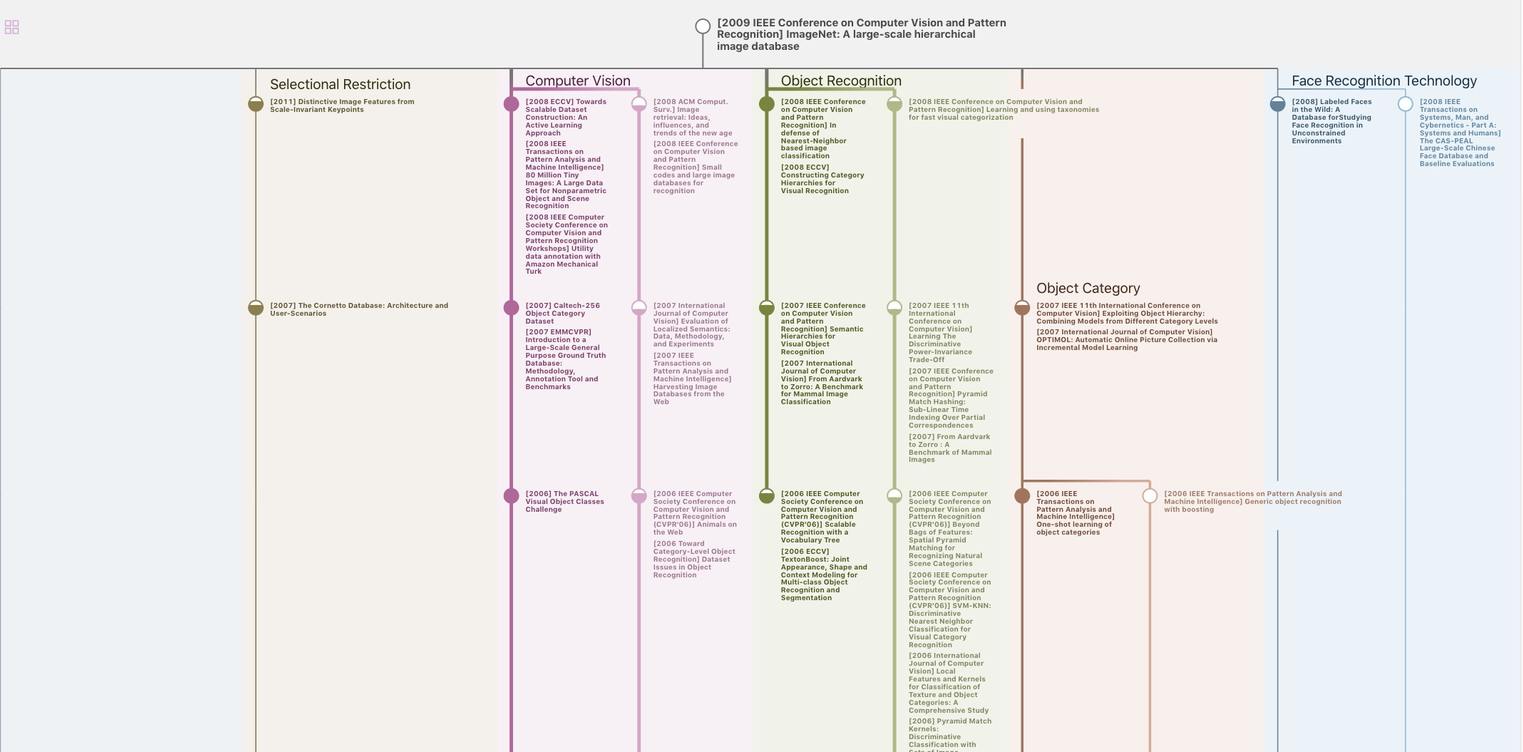
生成溯源树,研究论文发展脉络
Chat Paper
正在生成论文摘要