Optimization of Graph Neural Networks with Natural Gradient Descent
2020 IEEE International Conference on Big Data (Big Data)(2020)
摘要
In this work, we propose to employ information-geometric tools to optimize a graph neural network architecture such as the graph convolutional networks. More specifically, we develop optimization algorithms for the graph-based semi-supervised learning by employing the natural gradient information in the optimization process. This allows us to efficiently exploit the geometry of the underlying statistical model or parameter space for optimization and inference. To the best of our knowledge, this is the first work that has utilized the natural gradient for the optimization of graph neural networks that can be extended to other semi-supervised problems. Efficient computations algorithms are developed and extensive numerical studies are conducted to demonstrate the superior performance of our algorithms over existing algorithms such as ADAM and SGD.
更多查看译文
关键词
Graph neural network,Fisher information,natural gradient descent,network data
AI 理解论文
溯源树
样例
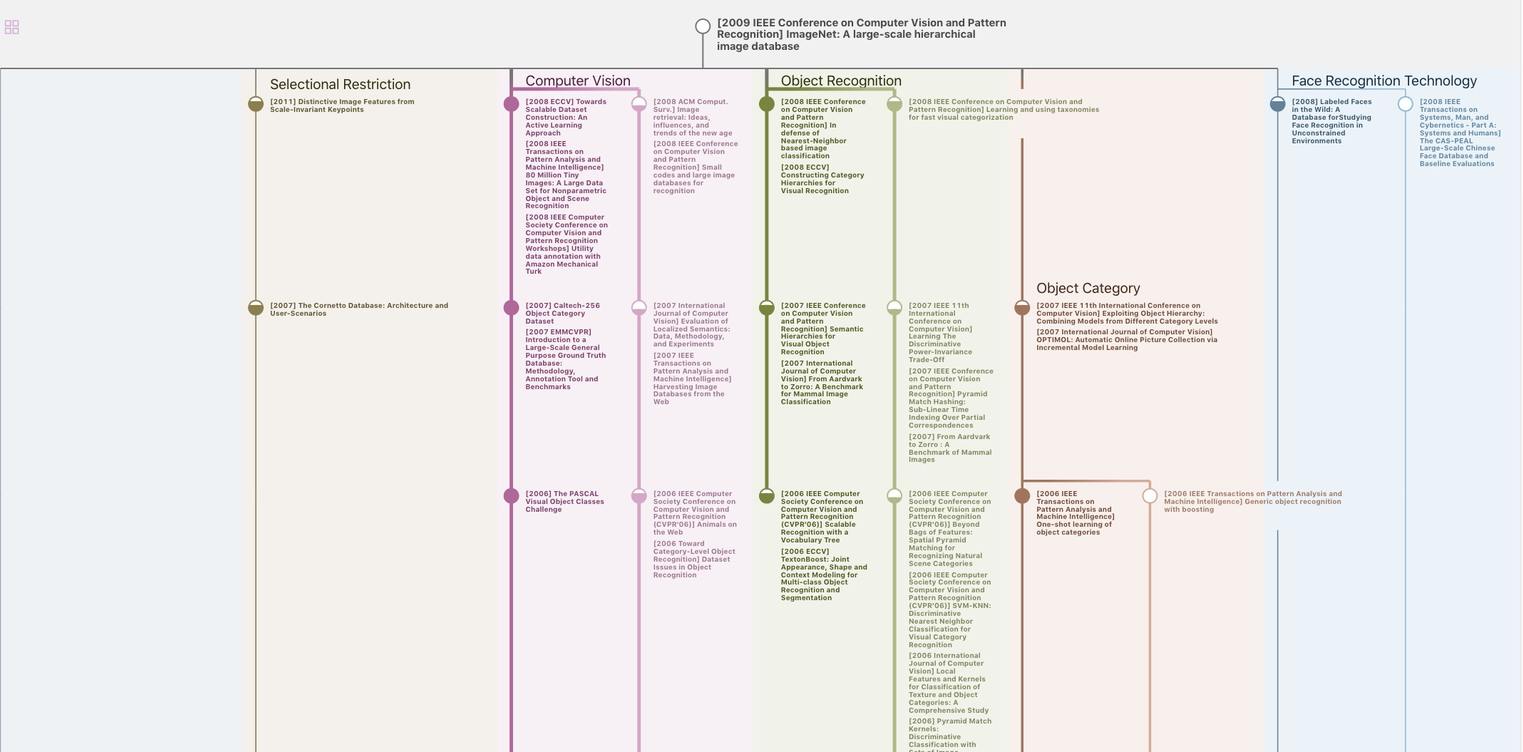
生成溯源树,研究论文发展脉络
Chat Paper
正在生成论文摘要