A Comparison of Supervised and Unsupervised Pre-Training of End-to-End Models.
Interspeech(2021)
摘要
In the absence of large-scale in-domain supervised training data, ASR models can achieve reasonable performance through pre-training on additional data that is unlabeled, mismatched or both. Given such data constraints, we compare pre-training end-to-end models on matched but unlabeled data (unsupervised) and on labeled but mismatched data (supervised), where the labeled data is mismatched in either domain or language. Across encoder architectures, pre-training methods and languages, our experiments indicate that both types of pre-training improve performance, with relative WER reductions of 15-30% in the domain mismatch case and up to 15% in the language mismatch condition. We further find that the advantage from unsupervised pre-training is most prominent when there is no matched and labeled fine-tuning data, provided that a sufficient amount of mismatched data is still available for supervised fine-tuning.
更多查看译文
关键词
Computer science,Machine learning,Training (meteorology),End-to-end principle,Artificial intelligence
AI 理解论文
溯源树
样例
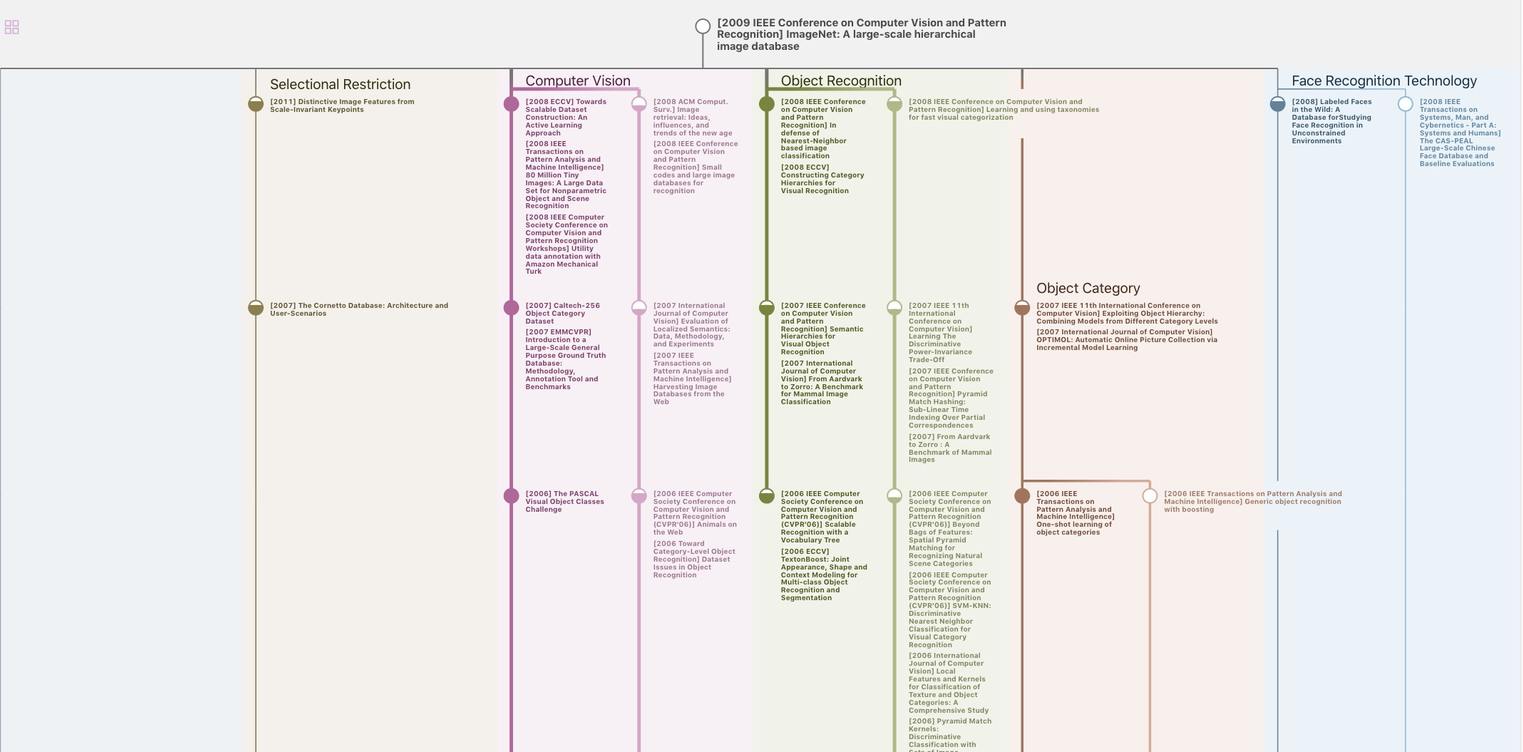
生成溯源树,研究论文发展脉络
Chat Paper
正在生成论文摘要