Heat Prediction Of High Energy Physical Data Based On Lstm Recurrent Neural Network
24TH INTERNATIONAL CONFERENCE ON COMPUTING IN HIGH ENERGY AND NUCLEAR PHYSICS (CHEP 2019)(2020)
摘要
High-energy physics computing is a typical data-intensive calculation. Each year, petabytes of data needs to be analyzed, and data access performance is increasingly demanding. The tiered storage system scheme for building a unified namespace has been widely adopted. Generally, data is stored on storage devices with different performances and different prices according to different access frequency. When the heat of the data changes, the data is then migrated to the appropriate storage tier. At present, heuristic algorithms based on artificial experience are widely used in data heat prediction. Due to the differences in computing models of different users, the accuracy of prediction is low. A method for predicting future access popularity based on file access characteristics with the help of LSTM deep learning algorithm is proposed as the basis for data migration in hierarchical storage. This paper uses the real data of high-energy physics experiment LHAASO as an example for comparative testing. The results show that under the same test conditions, the model has higher prediction accuracy and stronger applicability than existing prediction models.
更多查看译文
关键词
Scientific Computing,Data Analysis
AI 理解论文
溯源树
样例
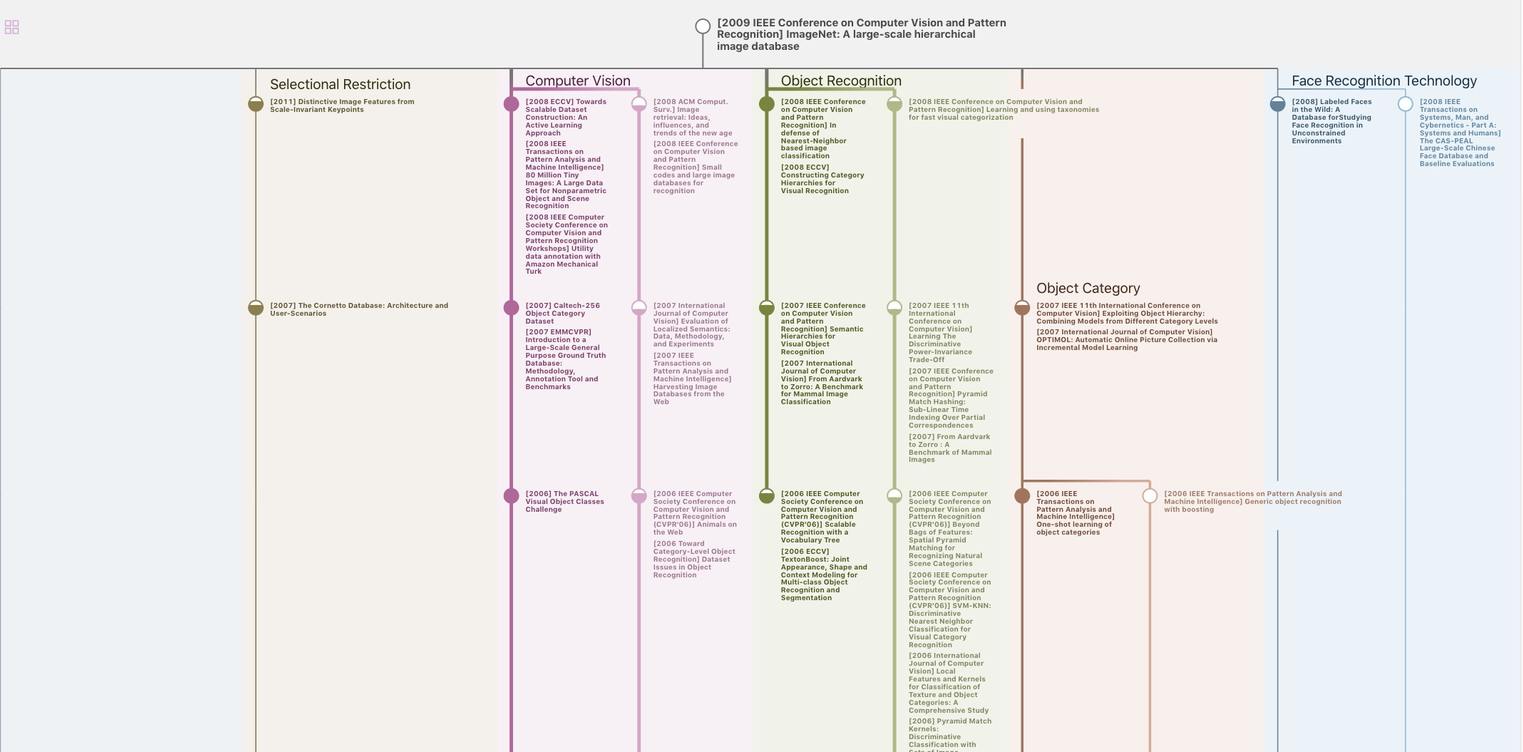
生成溯源树,研究论文发展脉络
Chat Paper
正在生成论文摘要