Code-free Deep Learning for Multi-Modality Medical Image Classification
Nature machine intelligence(2021)
摘要
A number of large technology companies have created code-free cloud-based platforms that allow researchers and clinicians without coding experience to create deep learning algorithms. In this study, we comprehensively analyse the performance and featureset of six platforms, using four representative cross-sectional and en-face medical imaging datasets to create image classification models. The mean (s.d.) F1 scores across platforms for all model–dataset pairs were as follows: Amazon, 93.9 (5.4); Apple, 72.0 (13.6); Clarifai, 74.2 (7.1); Google, 92.0 (5.4); MedicMind, 90.7 (9.6); Microsoft, 88.6 (5.3). The platforms demonstrated uniformly higher classification performance with the optical coherence tomography modality. Potential use cases given proper validation include research dataset curation, mobile ‘edge models’ for regions without internet access, and baseline models against which to compare and iterate bespoke deep learning approaches.
更多查看译文
关键词
Diagnostic markers,Eye manifestations,Mathematics and computing,Medical imaging,Science,technology and society,Engineering,general
AI 理解论文
溯源树
样例
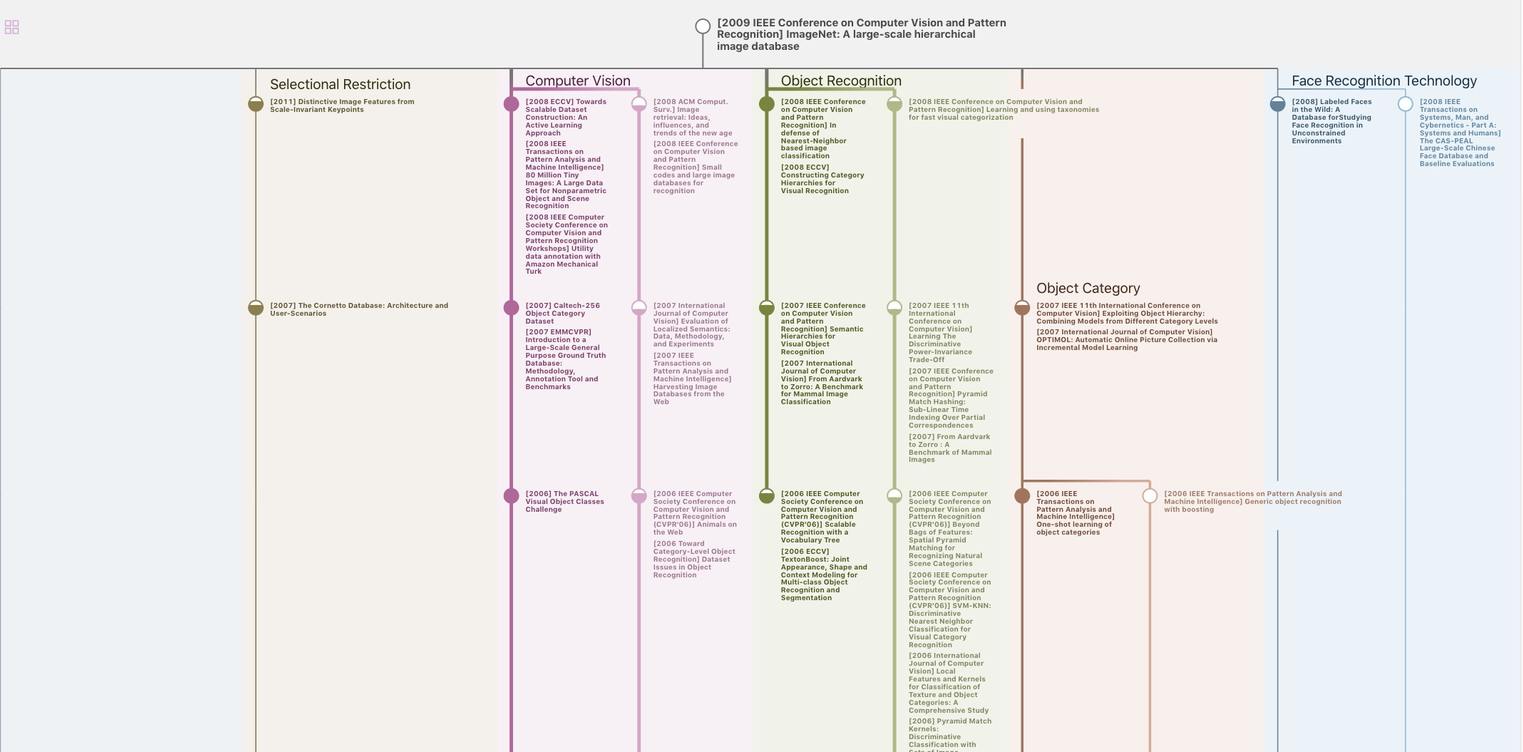
生成溯源树,研究论文发展脉络
Chat Paper
正在生成论文摘要