A Hierarchical Network Intrusion Detection Model Based on Unsupervised Clustering
Proceedings of the 13th International Conference on Management of Digital EcoSystems(2021)
摘要
In the complex Internet of Things(IoT) environment, the security of digital ecosystems connected to the Web is guaranteed by network Intrusion Detection Systems (IDS). So far, the existing unsupervised learning methods extract the features of network traffic at the overall level, which cannot guarantee real-time network intrusion detection. To fill this gap, we propose a hierarchical network intrusion detection model based on unsupervised clustering, which is realized by combining Deep Auto-Encoder(DAE) and Gaussian Mixture Model (GMM). For new network traffic, essential features are extracted based on the first few packets, which guarantee real-time network intrusion detection. The proposed model adopts a two-layer hierarchical structure. The first layer namely the anomaly detection sub-model is based on DAGMM, which can detect abnormal traffic in real-time. The second layer namely the attack recognition sub-model identifies the attack categories of abnormal traffic detected by the anomaly detection sub-model, and getting rid of the difficulty of reconstructing abnormal traffic in DAE. The experimental results on the CICIDS2017 dataset show that the proposed model has better performance in detecting abnormal traffic and identifying the attack categories of abnormal traffic than other existing unsupervised methods.
更多查看译文
AI 理解论文
溯源树
样例
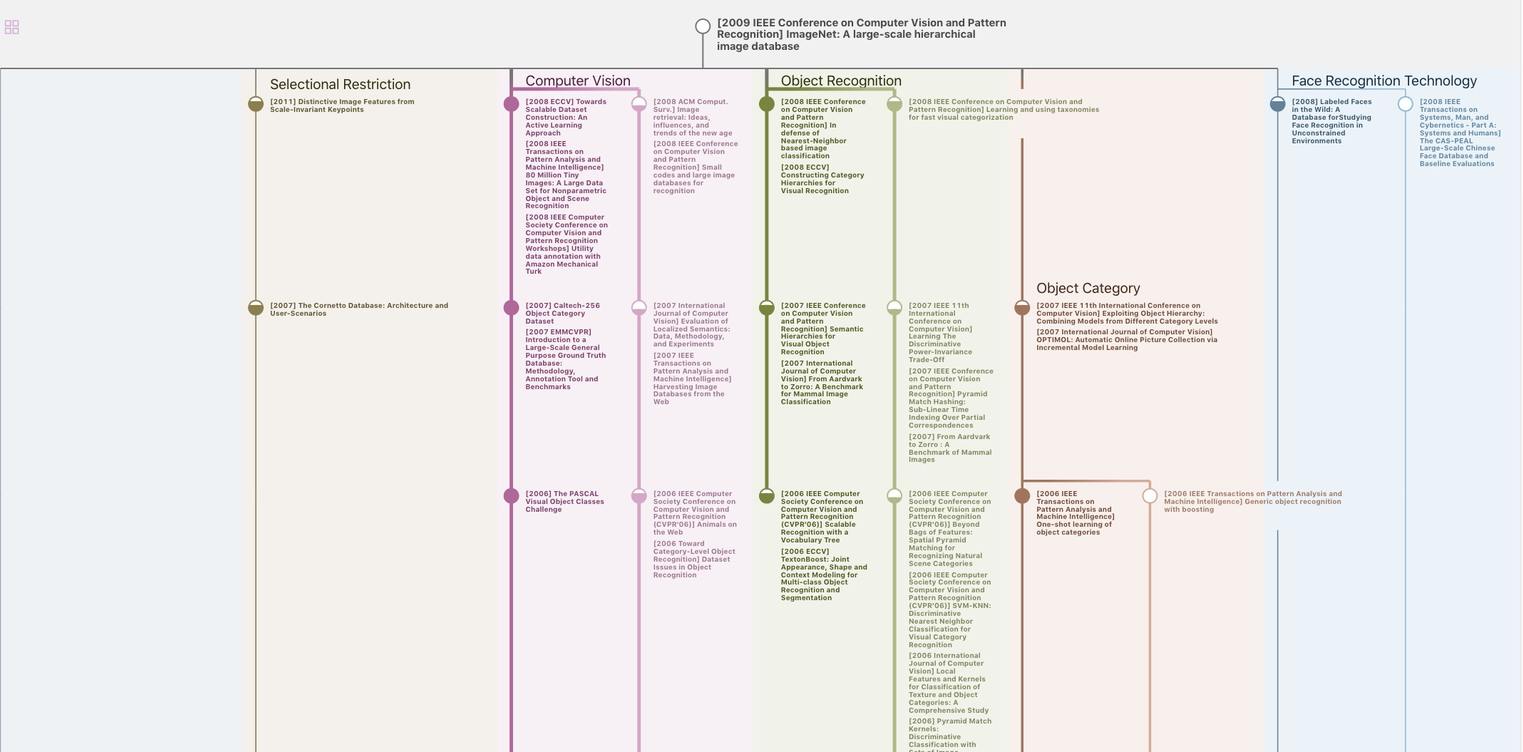
生成溯源树,研究论文发展脉络
Chat Paper
正在生成论文摘要