Three-Dimensional Convolutional Autoencoder Extracts Features of Structural Brain Images with a "diagnostic Label-Free" Approach: Application to Schizophrenia Datasets
FRONTIERS IN NEUROSCIENCE(2021)
摘要
There has been increasing interest in performing psychiatric brain imaging studies using deep learning. However, most studies in this field disregard three-dimensional (3D) spatial information and targeted disease discrimination, without considering the genetic and clinical heterogeneity of psychiatric disorders. The purpose of this study was to investigate the efficacy of a 3D convolutional autoencoder (3D-CAE) for extracting features related to psychiatric disorders without diagnostic labels. The network was trained using a Kyoto University dataset including 82 patients with schizophrenia (SZ) and 90 healthy subjects (HS) and was evaluated using Center for Biomedical Research Excellence (COBRE) datasets, including 71 SZ patients and 71 HS. We created 16 3D-CAE models with different channels and convolutions to explore the effective range of hyperparameters for psychiatric brain imaging. The number of blocks containing two convolutional layers and one pooling layer was set, ranging from 1 block to 4 blocks. The number of channels in the extraction layer varied from 1, 4, 16, and 32 channels. The proposed 3D-CAEs were successfully reproduced into 3D structural magnetic resonance imaging (MRI) scans with sufficiently low errors. In addition, the features extracted using 3D-CAE retained the relation to clinical information. We explored the appropriate hyperparameter range of 3D-CAE, and it was suggested that a model with 3 blocks may be related to extracting features for predicting the dose of medication and symptom severity in schizophrenia.
更多查看译文
关键词
deep learning,machine learning,neuroimaging,schizophrenia,structural MRI,convolutional autoencoder,diagnostic label
AI 理解论文
溯源树
样例
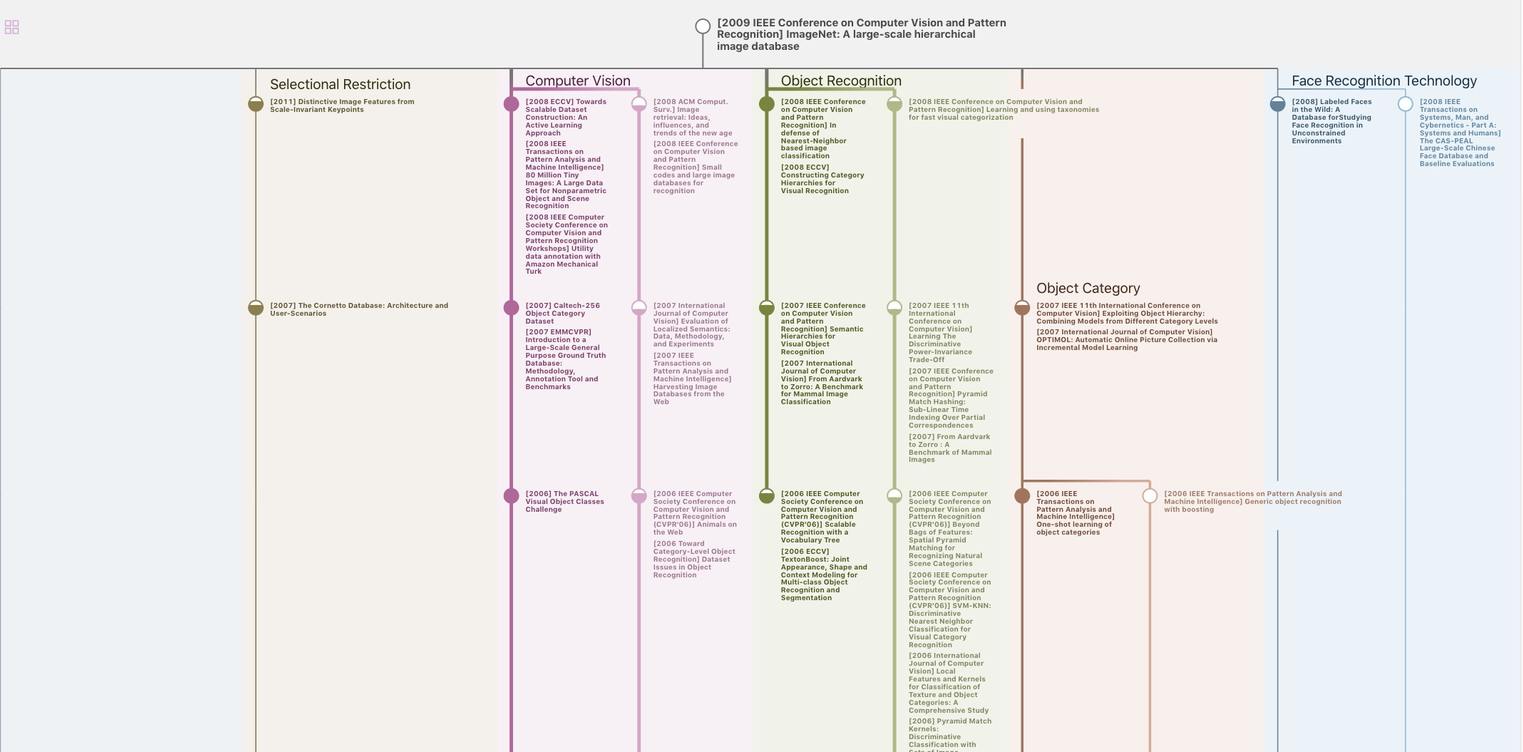
生成溯源树,研究论文发展脉络
Chat Paper
正在生成论文摘要