Semantically Robust Unpaired Image Translation for Data with Unmatched Semantics Statistics.
ICCV(2021)
摘要
Many applications of unpaired image-to-image translation require the input contents to be preserved semantically during translations. Unaware of the inherently unmatched semantics distributions between source and target domains, existing distribution matching methods (i.e., GAN-based) can give undesired solutions. In specific, although producing visually reasonable outputs, the learned models usually flip the semantics of the inputs. To tackle this without using extra supervisions, we propose to enforce the translated outputs to be semantically invariant w.r.t. small perceptual variations of the inputs, a property we call""semantic robustness"". By optimizing a robustness loss w.r.t. multi-scale feature space perturbations of the inputs, our method effectively reduces semantics flipping and produces translations that outperform existing methods both quantitatively and qualitatively.
更多查看译文
关键词
Image and video synthesis,Neural generative models
AI 理解论文
溯源树
样例
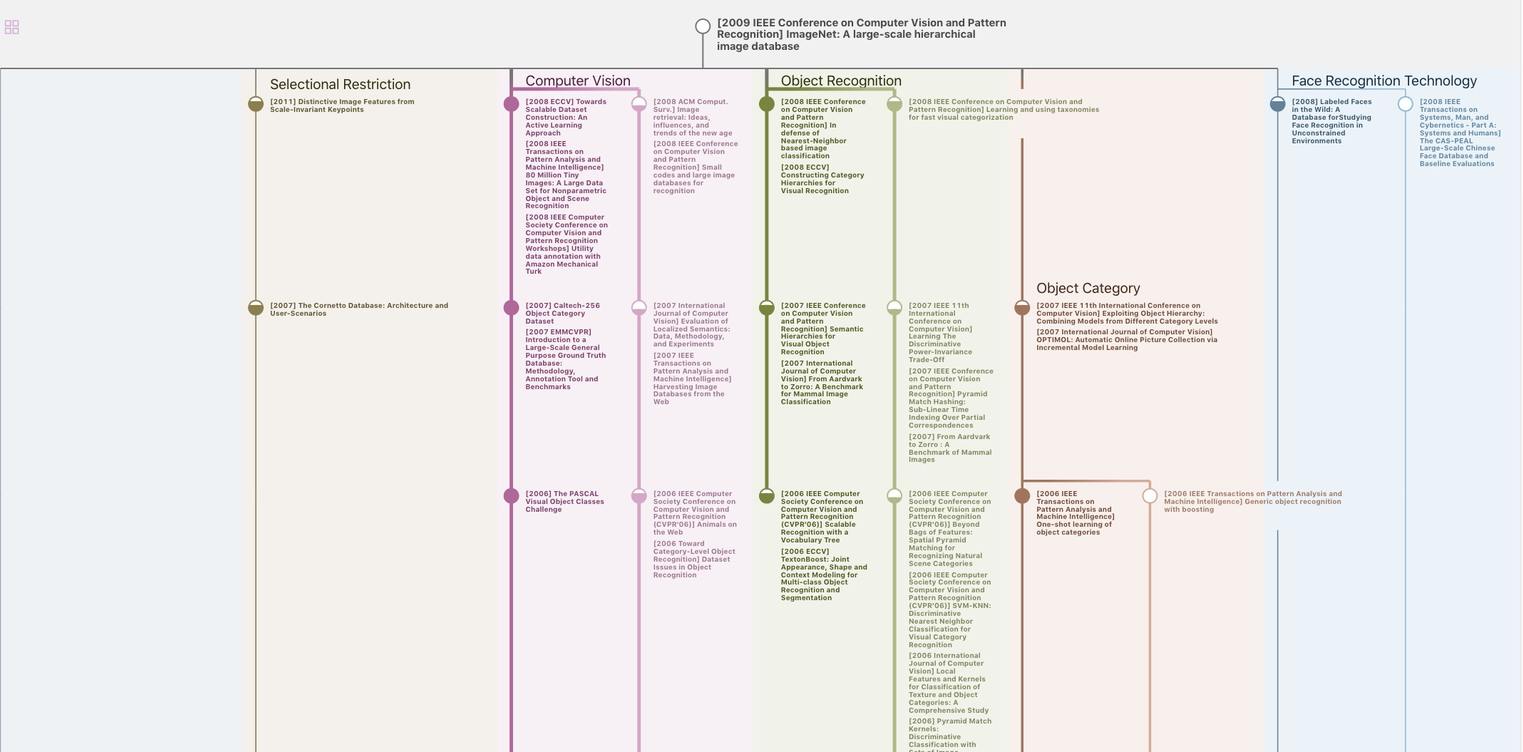
生成溯源树,研究论文发展脉络
Chat Paper
正在生成论文摘要