Comparison of Dimensionality Reduction Methods for Road Surface Identification System.
SAI (2)(2020)
摘要
Road surface identification is attracting more attention in recent years as part of the development of autonomous vehicle technologies. Most works consider multiple sensors and many features in order to produce a more reliable and robust result. However, on-board limitations and generalization concerns dictate the need for dimensionality reduction methods. This work considers four dimensionality reduction methods: principal component analysis, sequential feature selection, ReliefF, and a novel feature ranking method. These methods are used on data obtained from a modified passenger car with four types of sensors. Results were obtained using three classifiers (linear discriminant analysis, support vector machines, and random forests) and a late fusion method based on alpha integration, reaching up to 96.10% accuracy. The considered dimensionality reduction methods were able to reduce the number of features required for classification greatly and increased classification performance. Furthermore, the proposed method was faster than ReliefF and sequential feature selection and yielded similar improvements.
更多查看译文
关键词
Classification,Decision fusion,Feature selection,Road surface identification,Self-driving vehicles
AI 理解论文
溯源树
样例
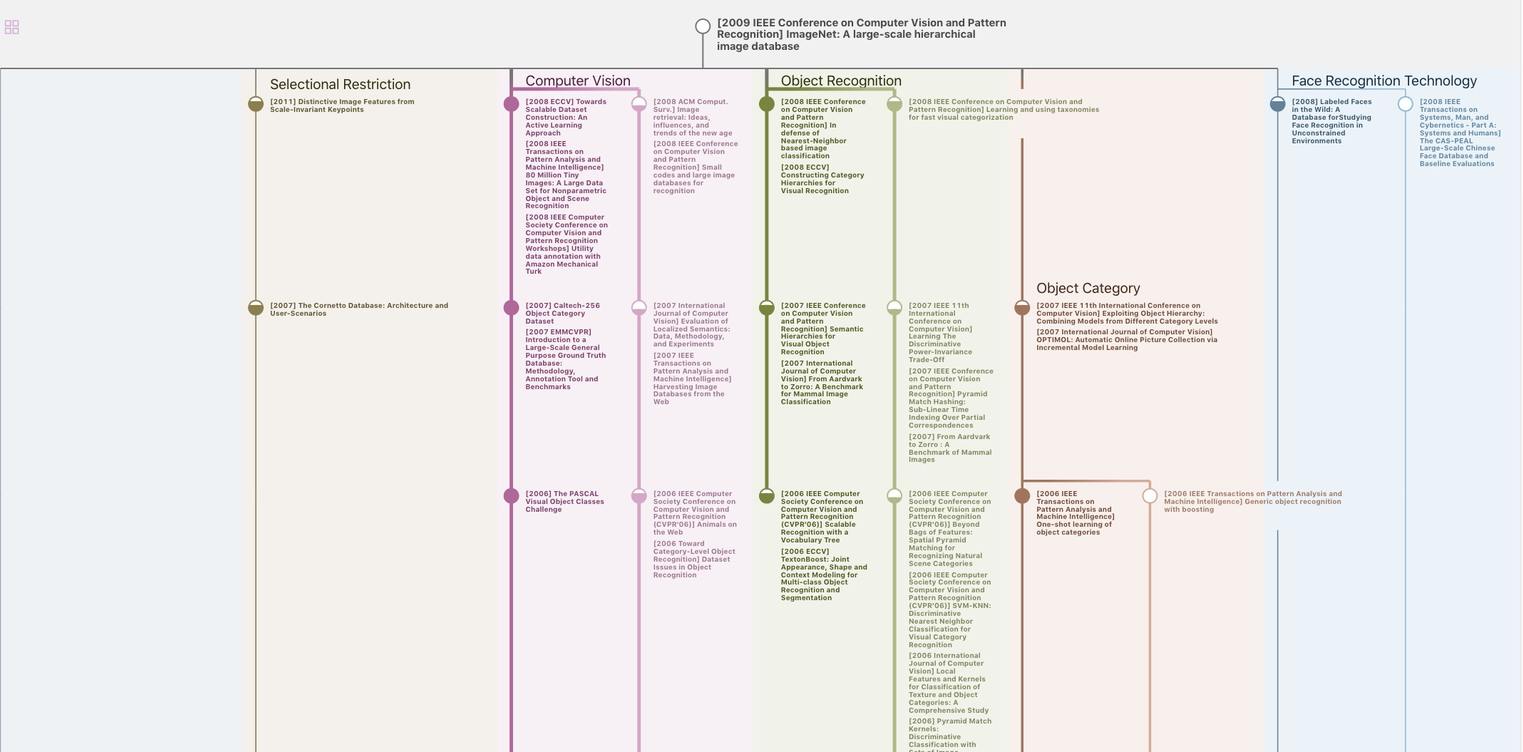
生成溯源树,研究论文发展脉络
Chat Paper
正在生成论文摘要