Interpretable Diagnosis of ADHD Based on Wavelet Features and Logistic Regression
PROGRESS IN ARTIFICIAL INTELLIGENCE AND PATTERN RECOGNITION(2021)
摘要
Despite arising in childhood, attention deficit hyperactivity disorder (ADHD) can persist into adulthood, compromising the individual's social skills. ADHD diagnosis is a real challenge due to its dependence on the clinical observation of the patient, the information provided by parents and teachers, and the clinicians' expertise. Therefore, there is great interest in studying objective biomarkers extracted from electroencephalographic (EEG) signals supporting accurate diagnoses. However, the non-stationarity and non-linearity characteristics of the EEG hinders the development of such tools. This paper presents a methodology for supporting the ADHD diagnosis by extracting features from the Discrete Wavelet transform of EEG signals. Due to the failed inhibitory control symptom, we consider EEG signals recorded under the Reward Stop Signal Task paradigm. Then, the logistic regressor learns the linear boundary discriminating between ADHD and healthy control subjects. As a benefit, the weighting vector supporting the classification also provides a straightforward interpretation of features in spatial locations and decomposition levels. The cross-validated classification results prove that the approach reaches an F1 score of 96% on a dataset of 34 children. Besides, the interpretability results support the hypothesis that the motivational effect leads the poor impulse control in ADHD.
更多查看译文
关键词
Attention deficit hyperactivity disorder (ADHD),Electroencephalograpy (EEG),Discrete Wavelet Transform (DWT),Interpretability
AI 理解论文
溯源树
样例
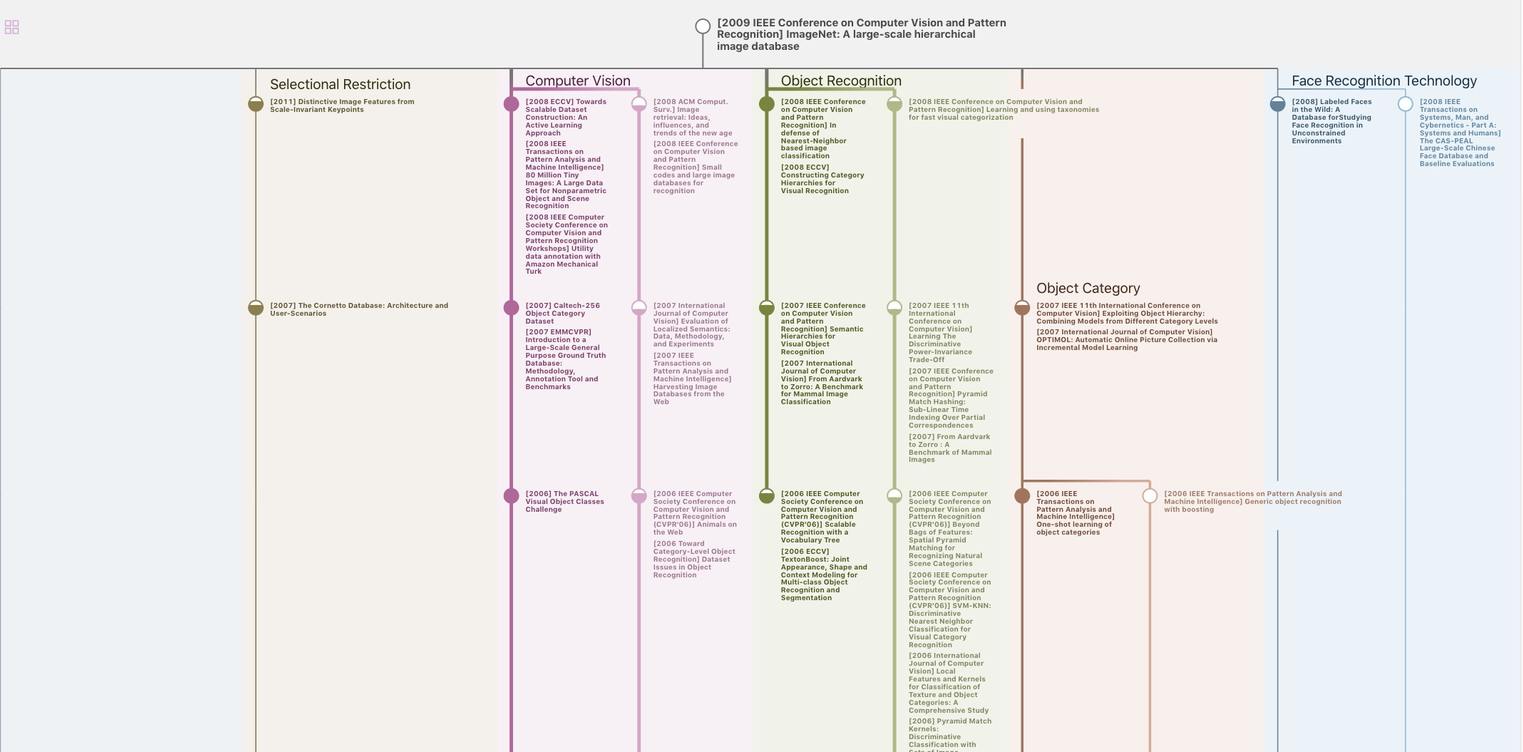
生成溯源树,研究论文发展脉络
Chat Paper
正在生成论文摘要