Artificial neural network can improve the accuracy of a markerless skeletal model in L5/S1 position estimation during symmetric lifting
Journal of Biomechanics(2022)
摘要
This study investigated whether using an artificial neural network (ANN) method for L5/S1 position estimation based on the Kinect markerless skeletal model can produce more accurate data than measurements using the original Kinect skeletal model during symmetric lifting tasks. Twenty participants performed three symmetric lifting tasks twice at three vertical lifting height paths. Their postural data were simultaneously collected by a Kinect and a reference motion tracking system (MTS). The Kinect-based data are used as the model inputs, while its outputs are based on MTS. Three-layer ANN models to predict the L5/S1 position over the entire lifting duration were trained by identifying the relationship between the seven inputs (the participant’s height and weight and the Kinect-based trunk angle, left knee angle, and left hip joint coordinates on the X-axis, Y-axis, and Z-axis) and three outputs (the reference L5/S1 position on the X-axis, Y-axis, and Z-axis). As a measure of error, the distances between the reference anatomical L5/S1 position and the predicted positions (by the ANN-Kinect system and the Kinect system) were calculated and compared. The results showed that introducing the ANN method can significantly (p < 0.0001) reduce the L5/S1 position estimation error (5.12 ± 1.83 cm) in comparison with directly using the original data output from the skeletal model driven by Kinect data (20.54 ± 3.24 cm). This method provides an alternative for L5/S1 position estimation while retaining the advantages of using Kinect such as portability, easy of use, and being equipped with the function of automatic skeletal identification.
更多查看译文
关键词
Lifting,L5/S1,Neural network,Kinect
AI 理解论文
溯源树
样例
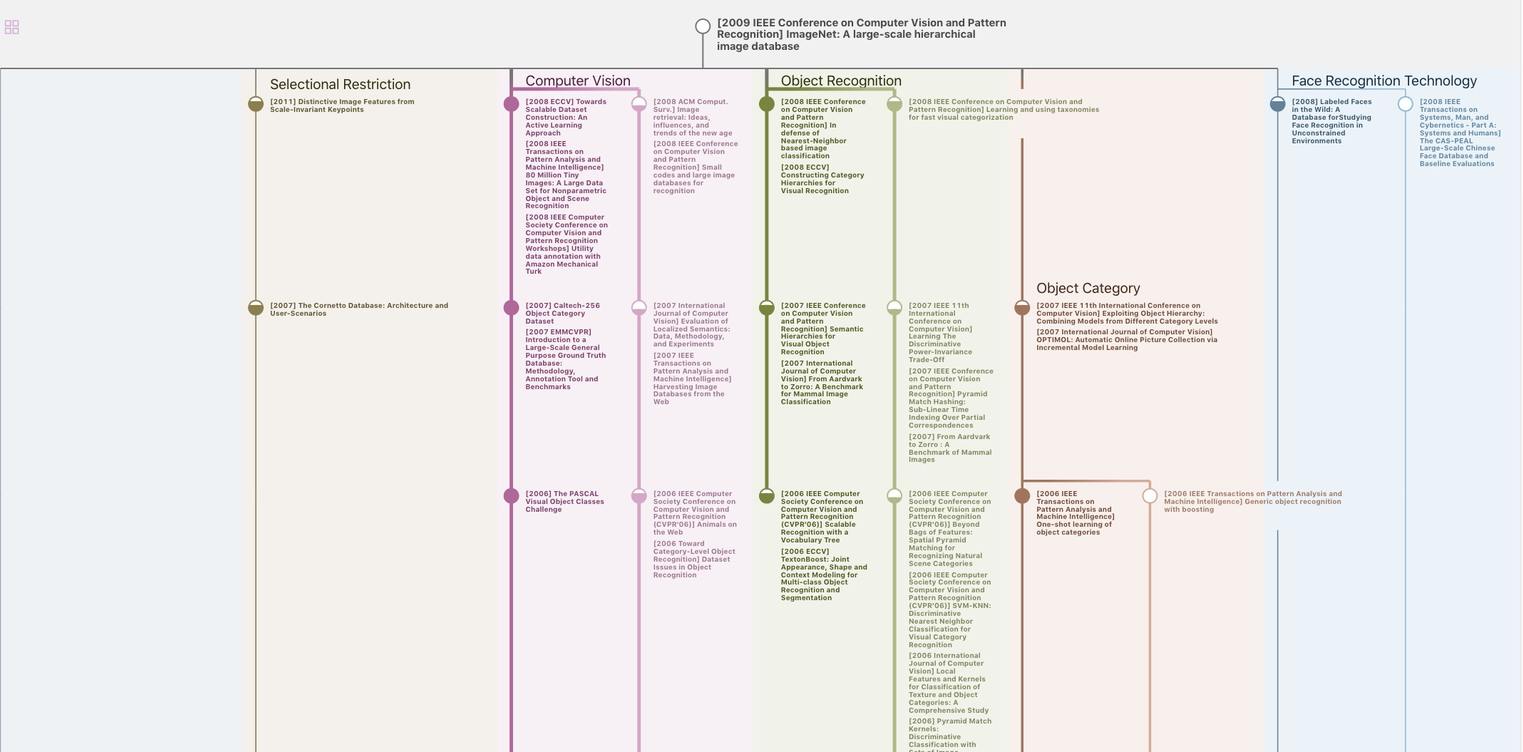
生成溯源树,研究论文发展脉络
Chat Paper
正在生成论文摘要